
An official website of the United States government
The .gov means it's official. Federal government websites often end in .gov or .mil. Before sharing sensitive information, make sure you're on a federal government site.
The site is secure. The https:// ensures that you are connecting to the official website and that any information you provide is encrypted and transmitted securely.
- Publications
- Account settings
- Browse Titles
NCBI Bookshelf. A service of the National Library of Medicine, National Institutes of Health.
Institute of Medicine (US) Committee on Sleep Medicine and Research; Colten HR, Altevogt BM, editors. Sleep Disorders and Sleep Deprivation: An Unmet Public Health Problem. Washington (DC): National Academies Press (US); 2006.


Sleep Disorders and Sleep Deprivation: An Unmet Public Health Problem.
- Hardcopy Version at National Academies Press
2 Sleep Physiology
CHAPTER SUMMARY This chapter provides a brief overview of sleep physiology and how sleep patterns change over an individual’s life span. Humans spend about one-third of their lives asleep. There are two types of sleep, non-rapid eye movement (NREM) sleep and rapid eye movement (REM) sleep. NREM sleep is divided into stages 1, 2, 3, and 4, representing a continuum of relative depth. Each has unique characteristics including variations in brain wave patterns, eye movements, and muscle tone. Circadian rhythms, the daily rhythms in physiology and behavior, regulate the sleep-wake cycle. In addition, the sleep-wake system is thought to be regulated by the interplay of two major processes, one that promotes sleep and one that maintains wakefulness.
Humans spend about one-third of their lives asleep, yet most individuals know little about sleep. Although its function remains to be fully elucidated, sleep is a universal need of all higher life forms including humans, absence of which has serious physiological consequences. This chapter provides an overview of basic sleep physiology and describes the characteristics of REM and NREM sleep. Sleep and circadian-generating systems are also reviewed. The chapter ends with a discussion about how sleep patterns change over an individual’s life span.
- SLEEP ARCHITECTURE
Sleep architecture refers to the basic structural organization of normal sleep. There are two types of sleep, non-rapid eye-movement (NREM) sleep and rapid eye-movement (REM) sleep. NREM sleep is divided into stages 1, 2, 3, and 4, representing a continuum of relative depth. Each has unique characteristics including variations in brain wave patterns, eye movements, and muscle tone. Sleep cycles and stages were uncovered with the use of electroencephalographic (EEG) recordings that trace the electrical patterns of brain activity ( Loomis et al., 1937 ; Dement and Kleitman, 1957a ).
Two Types of Sleep
Over the course of a period of sleep, NREM and REM sleep alternate cyclically ( Figure 2-1 ). The function of alternations between these two types of sleep is not yet understood, but irregular cycling and/or absent sleep stages are associated with sleep disorders ( Zepelin et al., 2005 ). For example, instead of entering sleep through NREM, as is typical, individuals with narcolepsy enter sleep directly into REM sleep ( Carskadon and Rechtschaffen, 2005 ).
Progression of sleep states across a single night in young adult. SOURCE: Carskadon and Dement (2005).
NREM and REM Sleep Cycles
A sleep episode begins with a short period of NREM stage 1 progressing through stage 2, followed by stages 3 and 4 and finally to REM. However, individuals do not remain in REM sleep the remainder of the night but, rather, cycle between stages of NREM and REM throughout the night ( Figure 2-1 ). NREM sleep constitutes about 75 to 80 percent of total time spent in sleep, and REM sleep constitutes the remaining 20 to 25 percent. The average length of the first NREM-REM sleep cycle is 70 to 100 minutes. The second, and later, cycles are longer lasting—approximately 90 to 120 minutes ( Carskadon and Dement, 2005 ). In normal adults, REM sleep increases as the night progresses and is longest in the last one-third of the sleep episode. As the sleep episode progresses, stage 2 begins to account for the majority of NREM sleep, and stages 3 and 4 may sometimes altogether disappear.
Four Stages of NREM Sleep
The four stages of NREM sleep are each associated with distinct brain activity and physiology. Figure 2-2 shows the EEG patterns characteristic of the four NREM stages. Other instruments are used to track characteristic changes in eye movement and muscle tone.
Characteristic EEG activity of each of the four stages of NREM sleep. NOTE: In stage 2, the arrow indicates a K-complex, and the underlining shows two sleep spindles.
Stage 1 Sleep
NREM stage 1 sleep serves a transitional role in sleep-stage cycling. Aside from newborns and those with narcolepsy and other specific neurological disorders, the average individual’s sleep episode begins in NREM stage 1. This stage usually lasts 1 to 7 minutes in the initial cycle, constituting 2 to 5 percent of total sleep, and is easily interrupted by a disruptive noise. Brain activity on the EEG in stage 1 transitions from wakefulness (marked by rhythmic alpha waves) to low-voltage, mixed-frequency waves. Alpha waves are associated with a wakeful relaxation state and are characterized by a frequency of 8 to 13 cycles per second ( Carskadon and Dement, 2005 ).
Stage 2 Sleep
Stage 2 sleep lasts approximately 10 to 25 minutes in the initial cycle and lengthens with each successive cycle, eventually constituting between 45 to 55 percent of the total sleep episode. An individual in stage 2 sleep requires more intense stimuli than in stage 1 to awaken. Brain activity on an EEG shows relatively low-voltage, mixed-frequency activity characterized by the presence of sleep spindles and K-complexes ( Figure 2-2 ). It is hypothesized that sleep spindles are important for memory consolidation. Individuals who learn a new task have a significantly higher density of sleep spindles than those in a control group ( Gais et al., 2002 ).
Stages 3 and 4, Slow-Wave Sleep
Sleep stages 3 and 4 are collectively referred to as slow-wave sleep (SWS), most of which occurs during the first third of the night. Each has distinguishing characteristics. Stage 3 lasts only a few minutes and constitutes about 3 to 8 percent of sleep. The EEG shows increased high-voltage, slow-wave activity ( Figure 2-2 ).
The last NREM stage is stage 4, which lasts approximately 20 to 40 minutes in the first cycle and makes up about 10 to 15 percent of sleep. The arousal threshold is highest for all NREM stages in stage 4. This stage is characterized by increased amounts of high-voltage, slow-wave activity on the EEG ( Carskadon and Dement, 2005 ).
REM sleep is defined by the presence of desynchronized (low-voltage, mixed-frequency) brain wave activity, muscle atonia, and bursts of rapid eye movements ( Carskadon and Dement, 2005 ). “Sawtooth” wave forms, theta activity (3 to 7 counts per second), and slow alpha activity also characterize REM sleep. During the initial cycle, the REM period may last only 1 to 5 minutes; however, it becomes progressively prolonged as the sleep episode progresses ( Carskadon and Dement, 2005 ). There are numerous physiological differences between NREM and REM sleep ( Table 2-1 ).
Physiological Changes During NREM and REM Sleep.
Dreaming is most often associated with REM sleep. Loss of muscle tone and reflexes likely serves an important function because it prevents an individual from “acting out” their dreams or nightmares while sleeping (see Chapter 3 ) ( Bader et al., 2003 ). Approximately 80 percent of vivid dream recall results after arousal from this stage of sleep ( Dement and Kleitman, 1957b ). REM sleep may also be important for memory consolidation ( Crick and Mitchison, 1983 ; Smith and Lapp, 1991 ).
Physiology During Sleep
In addition to the physiological changes listed in Table 2-1 , there are other body system changes that occur during sleep. Generally, these changes are well tolerated in healthy individuals, but they may compromise the sometimes fragile balance of individuals with vulnerable systems, such as those with cardiovascular diseases ( Parker and Dunbar, 2005 ). Physiological changes also occur in the following systems:
- Cardiovascular: Changes in blood pressure and heart rate occur during sleep and are primarily determined by autonomic nervous system activity. For instance, brief increases in blood pressure and heart rate occur with K-complexes, arousals, and large body movements ( Lugaresi et al., 1978 ; Catcheside et al., 2002 ; Blasi et al., 2003 ; Tank et al., 2003 ). Further, there is an increased risk of myocardial infarction in the morning due to the sharp increases in heart rate and blood pressure that accompany awakening ( Floras et al., 1978 ; Mulcahy et al., 1993 ).
- Sympathetic-nerve activity: Sympathetic-nerve activity decreases as NREM sleep deepens; however, there is a burst of sympathetic-nerve activity during NREM sleep due to the brief increase in blood pressure and heart rate that follows K-complexes. Compared to wakefulness, there is a rise in activity during REM sleep ( Somers et al., 1993 ).
- Respiratory: Ventilation and respiratory flow change during sleep and become increasingly faster and more erratic, specifically during REM sleep ( Krieger, 2000 ; Simon et al., 2002 ). Ventilation data during REM sleep are somewhat unclear, but they suggest that hypoventilation (deficient ventilation of the lungs that results in reduction in the oxygen content or increase in the carbon dioxide content of the blood or both) occurs in a similar way as during NREM sleep ( NLM, 2006 ). Several factors contribute to hypoventilation during NREM, and possibly REM, sleep such as reduced pharyngeal muscle tone ( Krieger, 2000 ; Simon et al., 2002 ). Further, during REM sleep, there is reduced rib cage movement and increased upper airway resistance due to the loss of tone in the intercostals and upper airway muscles ( Parker and Dunbar, 2005 ). More generally, ventilation and respiratory flow show less effective adaptive responses dur ing sleep. The cough reflex, which normally reacts to irritants in the airway, is suppressed during REM and NREM sleep. The hypoxic ventilatory response is also lower in NREM sleep than during wakefulness and decreases further during REM sleep. Similarly, the arousal response to respiratory resistance (for example, resistance in breathing in or out) is lowest in stage 3 and stage 4 sleep ( Douglas, 2005 ).
- Cerebral blood flow: NREM sleep is associated with significant reductions in blood flow and metabolism, while total blood flow and metabolism in REM sleep is comparable to wakefulness ( Madsen et al., 1991b ). However, metabolism and blood flow increase in certain brain regions during REM sleep, compared to wakefulness, such as the limbic system (which is involved with emotions), and visual association areas ( Madsen et al., 1991a ).
- Renal: There is a decreased excretion of sodium, potassium, chloride, and calcium during sleep that allows for more concentrated and reduced urine flow. The changes that occur during sleep in renal function are complex and include changes in renal blood flow, glomerular filtration, hormone secretion, and sympathetic neural stimulation ( Cianci et al., 1991 ; Van Cauter, 2000 ; Buxton et al., 2002 ).
- Endocrine: Endocrine functions such as growth hormone, thyroid hormone, and melatonin secretion are influenced by sleep. Growth hormone secretion typically takes place during the first few hours after sleep onset and generally occurs during SWS, while thyroid hormone secretion takes place in the late evening. Melatonin, which induces sleepiness, likely by reducing an alerting effect from the suprachiasmatic nucleus, is influenced by the light-dark cycle and is suppressed by light ( Parker and Dunbar, 2005 ).
- SLEEP-WAKE REGULATION
The Two-Process Model
The sleep-wake system is thought to be regulated by the interplay of two major processes, one that promotes sleep (process S) and one that maintains wakefulness (process C) ( Gillette and Abbott, 2005 ). Process S is the homeostatic drive for sleep. The need for sleep (process S) accumulates across the day, peaks just before bedtime at night and dissipates throughout the night.
Process C is wake promoting and is regulated by the circadian system. Process C builds across the day, serving to counteract process S and promote wakefulness and alertness. However, this wake-promoting system begins to decline at bedtime, serving to enhance sleep consolidation as the need for sleep dissipates across the night ( Gillette and Abbott, 2005 ). With an adequate night’s rest, the homeostatic drive for sleep is reduced, the circadian waking drive begins to increase, and the cycle starts over. In the absence of process C, total sleep time remains the same, but it is randomly distributed over the day and night; therefore, process C also works to consolidate sleep and wake into fairly distinct episodes ( Gillette and Abbott, 2005 ). Importantly, through synchronization of the circadian system, process C assists in keeping sleep-wakefulness cycles coordinated with environmental light-dark cycles.
Sleep-Generating Systems in the Brainstem
Sleep process S is regulated by neurons that shut down the arousal systems, thus allowing the brain to fall asleep. Many of these neurons are found in the preoptic area of the hypothalamus ( Figure 2-3A ). These neurons, containing molecules that inhibit neuronal communication, turn off the arousal systems during sleep. Loss of these nerve cells causes profound insomnia ( Saper et al., 2005a , c ). Inputs from other regions of the brain also greatly influence the sleep system. These include inputs from the lower brainstem that relay information about the state of the body (e.g., a full stomach is conducive to falling asleep), as well as from emotional and cognitive areas of the forebrain. In addition, as described further in the next section, there are inputs from the circadian system that allow the wake-sleep system to synchronize with the external day-night cycle, but also to override this cycle when it is necessitated by environmental needs.
Sleep-generating (A) and wake-generating (B) systems in the brain. NOTE: Cholinergic (ACh) cell groups; basal forebrain (BF); dopamine (DA); gamma-aminobutyric acid (GABA); galanin (Gal); histamine (His); serotonin (5-HT); locus coeruleus (LC); laterodorsal (more...)
The sleep-generating system also includes neurons in the pons that intermittently switch from NREM to REM sleep over the course of the night. These neurons send outputs to the lower brainstem and spinal cord that cause muscle atonia, REMs, and chaotic autonomic activity that characterize REM sleep. Other outputs are sent to the forebrain, including activation of the cholinergic pathways to the thalamus to activate the EEG.
Wake-Generating Systems in the Brainstem
Wakefulness is generated by an ascending arousal system from the brainstem that activates forebrain structures to maintain wakefulness ( Figure 2-3B ). This idea, originally put forward by Morruzzi and Magoun (1949) , has more recently been refined ( Jones, 2005a ; Saper et al., 2005c ). The main source for the ascending arousal influence includes two major pathways that originate in the upper brainstem. The first pathway, which takes origin from cholinergic neurons in the upper pons, activates parts of the thalamus that are responsible for maintaining transmission of sensory information to the cerebral cortex. The second pathway, which originates in cell groups in the upper brainstem that contain the monoamine neurotransmitters (norepinephrine, serotonin, dopamine, and histamine), enters the hypothalamus, rather than the thalamus, where it picks up inputs from nerve cells that contain peptides (orexin or hypocretin and melanin-concentrating hormone). These inputs then traverse the basal forebrain, where they pick up additional inputs from cells containing acetylcholine and gamma-aminobutyric acid. Ultimately, all of these inputs enter the cerebral cortex, where they diffusely activate the nerve cells and prepare them for the interpretation and analysis of incoming sensory information.
- CIRCADIAN RHYTHMS, THE 24-HOUR CLOCK
Circadian rhythms refer, collectively, to the daily rhythms in physiology and behavior. They control the sleep-wake cycle, modulate physical activity and food consumption, and over the course of the day regulate body temperature, heart rate, muscle tone, and hormone secretion. The rhythms are generated by neural structures in the hypothalamus that function as a biological clock ( Dunlap et al., 2004 ). Animals and plants possess endogenous clocks to organize daily behavioral and physiological rhythms in accord with the external day-night cycle ( Bunning, 1964 ). The basis for these clocks is believed to be a series of molecular pathways involving “clock” genes that are expressed in a nearly 24-hour rhythm ( Vitaterna et al., 2005 ).
In mammals, two proteins, Clock and Bmal1, bind together and move into the nucleus of the cell, where they bind to specific sites in the DNA that activate specific genes ( Figure 2-4 ). Among the genes that they activate are Period and Cryptochrome . The products of these genes also move back into the nucleus, where they disrupt the binding of Clock and Bmal1 to the DNA, thus inhibiting their own synthesis. This results in a rising and falling pattern of expression of the Period and Cryptochrome gene products with a periodicity that is very close to 24 hours.
Molecular mechanisms underlying the activity of the circadian clock. NOTE: The activation and deactivation of Period and Cryptochrome protein production is the basis of a negative-feedback loop that controls the ~24-hour cycle time of circadian clocks. (more...)
Many other genes are also regulated by Clock and Bmal1, and these genes cycle in this way in many tissues in the body, giving rise to daily patterns of activity. These rhythmically expressed genes contribute to many aspects of cellular function, including glucose and lipid metabolism, signal transduction, secretion, oxidative metabolism, and many others, suggesting the importance of the circadian system in many central aspects of life.
The Suprachiasmatic Nucleus
The suprachiasmatic nucleus (SCN) is responsible for regulating circadian rhythms in all organs. It receives direct inputs from a class of nerve cells in the retina that act as brightness detectors, which can reset the clock genes in the SCN on a daily basis. The SCN then transmits to the rest of the brain and body signals that bring all of the daily cycles in synchrony with the external day-night cycle.
The main influence of the SCN on sleep is due to a series of relays through the dorsomedial nucleus of the hypothalamus, which signals to the wake-sleep systems to coordinate their activity with the day-night cycles. The SCN also coordinates cycles of feeding, locomotor activity, and hormones, such as corticosteroids ( Chou et al., 2003 ). Under some conditions (e.g., limited food availability) when there are changes in the external temperature, or even under conditions of behavioral stress (e.g., the need to avoid a predator), animals must shift their daily cycles to survive. In such circumstances, the dorsomedial nucleus may shift to a new daily cycle, which can be completely out of phase with the SCN and the light-dark cycle, and its signals also shift the daily cycles of sleep, activity, feeding, and corticosteroid hormone secretion ( Saper et al., 2005b , c ).
Another major output of the SCN is to a pathway that controls the secretion of melatonin, a hormone produced by the pineal gland. Melatonin, which is mainly secreted at night, acts to further consolidate the circadian rhythms but has only limited effects directly on sleep.
Sleep and Thermoregulation
Body temperature regulation is subject to circadian system influence. An individual’s body temperature is higher during the day than at night ( Figure 2-5 ). At night there is a gradual decline in body temperature, a decrease in heat production (called the falling phase of the body temperature rhythm), and an increase in heat loss, all which promote sleep onset and maintenance, as well as EEG slow-wave activity. Conversely, there is a gradual increase in body temperature several hours before waking. The brain sends signals to other parts of the body that increase heat production and conservation in order to disrupt sleep and promote waking ( Szymusiak, 2005 ).
Body temperature in relation to time of day. SOURCE: NHBLI (2003).
- SLEEP PATTERNS CHANGE WITH AGE
Sleep architecture changes continuously and considerably with age. From infancy to adulthood, there are marked changes in how sleep is initiated and maintained, the percentage of time spent in each stage of sleep, and overall sleep efficiency (i.e., how successfully sleep is initiated and maintained). A general trend is that sleep efficiency declines with age ( Figure 2-6 ). Although the consequences of decreased sleep efficiency are relatively well documented, the reasons are complex and poorly understood. Exami nation of sleep characteristics by age, however, allows a closer understanding of the function of sleep for human development and successful aging.
Changes in sleep with age. NOTE: Time (in minutes) for sleep latency, amount of time spent awake after initially falling asleep (WASO), rapid eye movement (REM), non-rapid eye movement (NREM), stages 1, 2, and slow-wave sleep (SWS).
Newborns and Infants
At birth, sleep timing is distributed evenly across day and night for the first few weeks, with no regular rhythm or concentration of sleeping and waking. Newborns sleep about 16 to 18 hours per day; however, it is discontinuous with the longest continuous sleep episode lasting only 2.5 to 4 hours ( Adair and Bauchner, 1993 ; Roffwarg et al., 1966). Newborns have three types of sleep: quiet sleep (similar to NREM), active sleep (analogous to REM), and indeterminate sleep ( Davis et al., 2004 ). Sleep onset occurs through REM, not NREM, and each sleep episode consists of only one or two cycles ( Jenni and Carskadon, 2000 ; Davis et al., 2004 ). This distinctive sleep architecture occurs mostly because circadian rhythms have not yet been fully entrained ( Davis et al., 2004 ).
Circadian rhythms begin to arise around 2 to 3 months of age, leading to sleep consolidation that manifests in greater durations of wakefulness during the day and longer periods of sleep at night ( Sheldon, 2002 ). Circadian rhythm development in the first 3 months includes: emergence of the 24-hour core body temperature cycle (1 month of age); progression of nocturnal sleeping (2 months of age); and cycling of melatonin and cortisol hormones in a circadian rhythm (3 months of age) ( Jenni and Carskadon, 2000 ).
Sleep cycles also change because of the emergence of the circadian rhythm and a greater responsiveness to social cues (such as breast-feeding and bedtime routines). By 3 months of age, sleep cycles become more regular: sleep onset now begins with NREM, REM sleep decreases and shifts to the later part of the sleep cycle, and the total NREM and REM sleep cycle is typically 50 minutes ( Anders et al., 1995 ; Jenni and Carskadon, 2000 ). By 6 months of age, total sleep time reduces slightly and the longest continuous sleep episode lengthens to approximately 6 hours ( Anders et al., 1995 ; Jenni and Carskadon, 2000 ). As sleep cycles mature, the typical muscle paralysis of REM sleep replaces the propensity for movement in what was called “active sleep” as a newborn. By 12 months old, the infant typically sleeps 14 to 15 hours per day with the majority of sleep consolidated in the evening and during one to two naps during the day ( Anders et al., 1995 ).
Young Children
There are a limited number of studies that address normal sleep architecture in young children; however, one trend that appears to be consistent is that sleep amounts decrease as a child gets older. The reduction cannot be attributed solely to physiologic requirements, because cultural environments and social changes also influence changing sleep characteristics in young children. Total sleep time decreases by 2 hours from age 2 to age 5 (13 hours to 11) ( Roffward et al., 1966 ). Socially, the decrease in time asleep may be a result of decreased daytime napping, as most children discontinue napping between 3 and 5 years old ( Jenni and Carskadon, 2000 ). Other social and cultural factors that begin to influence sleep include how, with whom, and where children sleep and the introduction of school time routines ( Jenni and O’Connor, 2005 ).
Physiologically, it has been suggested that by the time children enter school (typically 6 years old) they begin to manifest circadian sleep phase preferences—a tendency to be a “night owl” or “morning bird” ( Jenni and Carskadon, 2000 ). Older children, however, are significantly more likely to experience challenges in initiating and maintaining sleep than younger children. In addition, older children are more likely to have nightmares, which usually disrupt sleep, making it discontinuous ( Beltramini and Hertzig, 1983 ). One study found that children appear to have longer REM sleep latencies than adolescents and consequently spend a greater percentage of sleep time in stages 3 and 4 ( Gaudreau et al., 2001 ).
Adolescents
A complex and bidirectional relationship exists between pubertal development and sleep. Studies underscore the importance of using pubertal stage, rather than chronologic age as the metric in understanding sleep, as has been found for other physiologic parameters in the second decade of life. It has been determined that adolescents require 9 to 10 hours of sleep each night ( Carskadon et al., 1993 ; Mercer et al., 1998 ), though few adolescents obtain adequate sleep. In the United States, the average total sleep time in a sample of eighth-grade students was found to be 7.9 hours ( Wolfson et al., 2003 ). Over a quarter of high school and college students were found to be sleep deprived ( Wolfson and Carskadon, 1998 ).
SWS and sleep latency time progressively declines with advancing pubertal development ( Carskadon et al., 1980 ); however, time spent in stage 2 increases ( Carskadon, 1982 ). These changes are likely in part due to pubertal and hormonal changes that accompany the onset of puberty ( Karacan et al., 1975 ). For instance, at midpuberty, there is significantly greater daytime sleepiness than at earlier stages of puberty. Afternoon sleepiness is greater than that in late afternoon and evening in more mature adolescents than in younger subjects. With increasing age, the total time spent sleeping decreases, as does REM sleep. However, if bedtime is fixed, the duration of REM sleep remains constant ( Carskadon, 1982 ; Carskadon et al., 1983 ).
Sleep architecture continues to change with age across adulthood. Two major attributes of age-related sleep changes are earlier wake time and reduced sleep consolidation ( Dijk et al., 2000 ). A hallmark change with age is a tendency toward earlier bedtimes and wake times. Older adults (approximately ages 65 to 75) typically awaken 1.33 hours earlier, and go to bed 1.07 hours earlier, than younger adults (approximately ages 20 to 30) ( Duffy et al., 1998 ). There are no conclusive studies that demonstrate why older adults experience earlier wake times, despite decreased sleep efficiency, but one hypothesis may be an advanced circadian pacemaker that accompanies age ( Dijk et al., 2000 ). It is unclear if this is due to older adults experiencing an increased sensitivity to light ( Dijk et al., 2000 ; Ancoli-Israel, 2005 ). Nonetheless, the consequences of an advanced circadian rhythm are a 1-hour advance in body temperature increase in the early morning and misaligned melatonin and cortisol secretion rhythms with the circadian clock ( Dijk et al., 2000 ).
Younger adults may experience brief awakenings, but they are usually minor and occur close to an REM sleep transition; thus, sleep remains relatively consolidated. Arousal occurring mostly from REM sleep in young adults suggests that there is a protective mechanism to keep from awakening during NREM sleep; however, this protective effect appears to also decline with age ( Dijk, 1998 ). As an individual ages (between the ages of 20 to 60), SWS declines at a rate of about 2 percent per decade ( Figure 2-6 ) ( Dijk et al., 1989 ; Astrom and Trojaborg, 1992 ; Landolt et al., 1996 ; Ancoli-Israel, 2005 ). Because arousal thresholds are typically highest during SWS, and because SWS declines with age, older adults experience more frequent awakenings during a sleep episode. Another important variable may be an age-related reduction both in homeostatic sleep pressure and circadian pacemaker effectiveness during the night ( Dijk et al., 2000 ).
Gender Differences
Although there have been few systematic studies, there appear to be gender-based differences in sleep and circadian rhythms. Available evidence is strongest in adults; however, gender differences have also been observed in infancy ( Bach et al., 2000 ; Moss and Robson, 1970 ; Hoppenbrouwers et al., 1989 ), childhood ( Meijer et al., 2000 ; Sadeh et al., 2000 ; Acebo et al., 1996 ), and adolescence ( Giannotti et al., 2002 ; Laberge et al., 2001 ). In adults, men spend greater time in stage 1 sleep ( Bixler et al., 1984 ) and experience more awakenings ( Kobayashi et al., 1998 ). Although women maintain SWS longer than men, they complain more often of difficulty falling asleep and midsleep awakenings. In contrast, men are more likely to complain of daytime sleepiness ( Ancoli-Israel, 2000 ).
In women, the menstrual cycle may influence sleep-wake activity; however, methodological challenges have limited the number of conclusive findings ( Metcalf, 1983 ; Leibenluft et al., 1994 ). There have been a number of studies that suggest that women’s sleep patterns are greatly affected during pregnancy and the postpartum period ( Karacan et al., 1968 ; Hertz et al., 1992 ; Lee and Zaffke, 1999 ; Driver and Shapiro, 1992 ). For example, women often experience considerable daytime sleepiness during pregnancy and during the first few postpartum months, and as will be discussed in greater detail in Chapter 3 , they are also at a higher risk of developing restless legs syndrome (Goodman et al., 1998; Lee et al., 2001 ).
Elderly People
Problematic sleep has adverse effects on all individuals, regardless of age; however, older people typically show an increase in disturbed sleep that can create a negative impact on their quality of life, mood, and alertness ( Ancoli-Israel, 2005 ; Bliwise, 2005 ). Elderly individuals sleep 36 percent less than children at age 5 ( Figure 2-6 ). Although the ability to sleep becomes more difficult, the need to sleep does not decrease with age ( Ancoli-Israel, 2005 ). Difficulty in initiating and maintaining sleep is cited in 43 percent of the elderly ( Foley et al., 1995 ), although these problems are more commonly among adults suffering from depression, respiratory symptoms, and physical disability, among others ( Ancoli-Israel, 2005 ). However, declining sleep efficiency and quality has also been observed in healthy older people ( Dijk et al., 2000 ).
Changes in sleep patterns affect males and females differently. The progressive decrease in SWS is one of the most prominent changes with aging; however, it appears to preferentially affect men. The gender difference is unclear, but it has been suggested that older women have “better-preserved” SWS than men ( Reynolds et al., 1985 ). Women ages 70 and older spend around 15 to 20 percent of total sleep time in stages 3 and 4; men of the same age spend only around 5 percent of total sleep time in stages 3 and 4 ( Redline et al., 2004 ). Another gender contrast is that older women go to bed and wake up earlier than older men, which suggests that body temperature rhythms are phase-advanced in elderly women ( Campbell et al., 1989 ; Moe et al., 1991 ; Monk et al., 1995 ). However, both men and women have increased stage 1 and decreased REM sleep.
Older people also experience a decrease in melatonin levels, which may be due to the gradual deterioration of the hypothalamic nuclei that drive circadian rhythms ( Ancoli-Israel, 2005 ). The inability to maintain long sleep episodes and bouts of wakefulness may reflect, in addition to other medical factors, a continuously decreasing sleep homeostasis ( Dijk et al., 2000 ; Bliwise, 2005 ). Other prominent factors are the continuous increase in sleep latency and nighttime awakenings and inconsistency of external cues such as light exposure (which tends to be low), irregular meal times, nocturia, and decreased mobility leading to a reduction in exercise ( Dijk et al., 2000 ; Ancoli-Israel, 2005 ; Bliwise, 2005 ).
- Acebo C, Millman RP, Rosenberg C, Cavallo A, Carskadon MA. Sleep, breathing, and cephalometrics in older children and young adults. Part I: Normative values. Chest. 1996; 109 (3):664–672. [ PubMed : 8617074 ]
- Adair RH, Bauchner H. Sleep problems in childhood. Current Problems in Pediatrics. 1993; 23 (4):142, 147–170. [ PubMed : 8500344 ]
- Ancoli-Israel S. Insomnia in the elderly: A review for the primary care practitioner. Sleep: Supplement. 2000; 23 (1):S23–S30. discussion S36–S38. [ PubMed : 10755805 ]
- Ancoli-Israel S. Sleep Research Society. SRS Basics of Sleep Guide. Westchester, IL: Sleep Research Society; 2005. Normal human sleep at different ages: Sleep in older adults; pp. 21–26.
- Anders TF, Sadeh A, Appareddy V. Normal sleep in neonates and children. In: Ferber RKM, editor. Principles and Practice of Sleep Medicine in the Child. Philadelphia: Saunders; 1995. pp. 7–18.
- Astrom C, Trojaborg W. Relationship of age to power spectrum analysis of EEG during sleep. Journal of Clinical Neurophysiology. 1992; 9 (3):424–430. [ PubMed : 1517410 ]
- Bach V, Telliez F, Leke A, Libert JP. Gender-related sleep differences in neonates in thermoneutral and cool environments. Journal of Sleep Research. 2000; 9 (3):249–254. [ PubMed : 11012863 ]
- Bader G, Gillberg C, Johnson M, Kadesjö B, Rasmussen P. Activity and sleep in children with ADHD. Sleep. 2003; 26 :A136.
- Beltramini AU, Hertzig ME. Sleep and bedtime behavior in preschool-aged children. Pediatrics. 1983; 71 (2):153–158. [ PubMed : 6823416 ]
- Bixler EO, Kales A, Jacoby JA, Soldatos CR, Vela-Bueno A. Nocturnal sleep and wakefulness: Effects of age and sex in normal sleepers. International Journal of Neuroscience. 1984; 23 (1):33–42. [ PubMed : 6724815 ]
- Blasi A, Jo J, Valladares E, Morgan BJ, Skatrud JB, Khoo MC. Cardiovascular variability after arousal from sleep: Time-varying spectral analysis. Journal of Applied Physiology. 2003; 95 (4):1394–1404. [ PubMed : 12819215 ]
- Bliwise D. Normal aging. In: Kryger MH, Roth T, Dement WC, editors. Principles and Practice of Sleep Medicine. 4th ed. Philadelphia: Saunders; 2005. pp. 24–38.
- Bunning E. The Physiological Clock. Berlin, Germany: Springer-Verlag; 1964.
- Buxton OM, Spiegel K, Van Cauter E. Modulation of endocrine function and metabolism by sleep and sleep loss. In: Lee-Chiong TL, Sateia MJ, Carskadon MA, editors. Sleep Medicine. Philadelphia: Hanley & Belfus; 2002. pp. 59–69.
- Campbell SS, Gillin JC, Kripke DF, Erikson P, Clopton P. Gender differences in the circadian temperature rhythms of healthy elderly subjects: Relationships to sleep quality. Sleep. 1989; 12 (6):529–536. [ PubMed : 2595176 ]
- Carskadon MA. The second decade. In: Guilleminault C, editor. Sleeping and Waking Disorders: Indications and Techniques. Menlo Park, CA: Addison-Wesley; 1982. pp. 99–125.
- Carskadon M, Dement W. Normal human sleep: An overview. In: Kryger MH, Roth T, Dement WC, editors. Principles and Practice of Sleep Medicine. 4th ed. Philadelphia: Elsevier Saunders; 2005. pp. 13–23.
- Carskadon MA, Rechtschaffen A. Monitoring and staging human sleep. In: Kryger MH, Roth TT, Dement WC, editors. Principles and Practice of Sleep Medicine. 4th ed. Philadelphia: Elsevier Saunders; 2005. pp. 1359–1377.
- Carskadon MA, Harvey K, Duke P, Anders TF, Litt IF, Dement WC. Pubertal changes in daytime sleepiness. Sleep. 1980; 2 (4):453–460. [ PubMed : 7403744 ]
- Carskadon MA, Orav EJ, Dement WC. Evolution of sleep and daytime sleepiness in adolescents. In: Guilleminault CLE, editor. Sleep/Wake Disorders: Natural History, Epidemiology, and Long-Term Evolution. New York: Raven Press; 1983. pp. 201–216.
- Carskadon MA, Vieira C, Acebo C. Association between puberty and delayed phase preference. Sleep. 1993; 16 (3):258–262. [ PubMed : 8506460 ]
- Catcheside PG, Chiong SC, Mercer J, Saunders NA, McEvoy RD. Noninvasive cardiovascular markers of acoustically induced arousal from non-rapid-eye-movement sleep. Sleep. 2002; 25 (7):797–804. [ PubMed : 12405616 ]
- Chou TC, Scammell TE, Gooley JJ, Gaus SE, Saper CB, Lu J. Critical role of dorsomedial hypothalamic nucleus in a wide range of behavioral circadian rhythms. Journal of Neuroscience. 2003; 23 (33):10691–10702. [ PMC free article : PMC6740926 ] [ PubMed : 14627654 ]
- Cianci T, Zoccoli G, Lenzi P, Franzini C. Loss of integrative control of peripheral circulation during desynchronized sleep. American Journal of Physiology. 1991; 261 (2 Pt 2):R373–R377. [ PubMed : 1877696 ]
- Crick F, Mitchison G. The function of dream sleep. Nature. 1983; 304 (5922):111–114. [ PubMed : 6866101 ]
- Davis KF, Parker KP, Montgomery GL. Sleep in infants and young children: Part one: Normal sleep. Journal of Pediatric Health Care. 2004; 18 (2):65–71. [ PubMed : 15007289 ]
- Dement W, Kleitman N. Cyclic variations in EEG during sleep and their relation to eye movements, body motility, and dreaming. Electroencephalography and Clinical Neurophysiology: Supplement. 1957a; 9 (4):673–690. [ PubMed : 13480240 ]
- Dement T, Kleitman N. The relation of eye movements during sleep to dream activity: An objective method for the study of dreaming. Journal of Experimental Psychology. 1957b; 53 (5):339–346. [ PubMed : 13428941 ]
- Dijk DCC. REM sleep as a gate to wakefulness during forced desynchrony in young and older people [abstract] Sleep. 1998; 21 (3):S298.
- Dijk DJ, Beersma DG, van den Hoofdakker RH. All night spectral analysis of EEG sleep in young adult and middle-aged male subjects. Neurobiology of Aging. 1989; 10 (6):677–682. [ PubMed : 2628779 ]
- Dijk DJ, Duffy JF, Czeisler CA. Contribution of circadian physiology and sleep homeostasis to age-related changes in human sleep. Chronobiology International. 2000; 17 (3):285–311. [ PubMed : 10841208 ]
- Douglas NJ. Respiratory physiology: Control of ventilation. In: Kryger MH, Roth T, Dement WC, editors. Principles and Practice of Sleep Medicine. 4th ed. Philadelphia: Elsevier Saunders; 2005. pp. 224–229.
- Driver HS, Shapiro CM. A longitudinal study of sleep stages in young women during pregnancy and postpartum. Sleep. 1992; 15 (5):449–453. [ PubMed : 1455129 ]
- Duffy JF, Dijk DJ, Klerman EB, Czeisler CA. Later endogenous circadian temperature nadir relative to an earlier wake time in older people. American Journal of Physiology. 1998; 275 (5 Pt 2):R1478–R1487. [ PubMed : 9791064 ]
- Dunlap JC, Loros JJ, DeCoursey PJ. Chronobiology: Biological Timekeeping. Sunderland, MA: Sinauer Associates; 2004.
- Floras JS, Jones JV, Johnston JA, Brooks DE, Hassan MO, Sleight P. Arousal and the circadian rhythm of blood pressure. Clinical Science and Molecular Medicine Supplement. 1978; 55 (4):395s–397s. [ PubMed : 282096 ]
- Foley DJ, Monjan AA, Brown SL, Simonsick EM, Wallace RB, Blazer DG. Sleep complaints among elderly persons: An epidemiologic study of three communities. Sleep. 1995; 18 (6):425–432. [ PubMed : 7481413 ]
- Gais S, Molle M, Helms K, Born J. Learning-dependent increases in sleep spindle density. Journal of Neuroscience. 2002; 22 (15):6830–6834. [ PMC free article : PMC6758170 ] [ PubMed : 12151563 ]
- Gaudreau H, Carrier J, Montplaisir J. Age-related modifications of NREM sleep EEG: From childhood to middle age. Journal of Sleep Research. 2001; 10 (3):165–172. [ PubMed : 11696069 ]
- Giannotti F, Cortesi F, Sebastiani T, Ottaviano S. Circadian preference, sleep and daytime behaviour in adolescence. Journal of Sleep Research. 2002; 11 (3):191–199. [ PubMed : 12220314 ]
- Gillette M, Abbott S. Sleep Research Society. SRS Basics of Sleep Guide. Westchester, IL: Sleep Research Society; 2005. Fundamentals of the circadian system; pp. 131–138.
- Goodman JD, Brodie C, Ayida GA. Restless leg syndrome in pregnancy. British Medical Journal. 1988; 297 (6656):1101–1102. [ PMC free article : PMC1834895 ] [ PubMed : 3143441 ]
- Hertz G, Fast A, Feinsilver SH, Albertario CL, Schulman H, Fein AM. Sleep in normal late pregnancy. Sleep. 1992; 15 (3):246–251. [ PubMed : 1621025 ]
- Hoppenbrouwers T, Hodgman J, Arakawa K, Sterman MB. Polysomnographic sleep and waking states are similar in subsequent siblings of SIDS and control infants during the first six months of life. Sleep. 1989; 12 (3):265–276. [ PubMed : 2740699 ]
- Jenni OG, Carskadon MA. Sleep Research Society. SRS Basics of Sleep Guide. Westchester, IL: Sleep Research Society; 2000. Normal human sleep at different ages: Infants to adolescents; pp. 11–19.
- Jenni OG, O'Connor BB. Children’s sleep: An interplay between culture and biology. Pediatrics. 2005; 115 (1 Suppl):204–216. [ PubMed : 15866854 ]
- Jones BE. Basic mechanisms of sleep-wake states. In: Kryger MH, Roth T, Dement WC, editors. Principles and Practice of Sleep Medicine. 4th ed. Philadelphia: Elsevier/Saunders; 2005. pp. 136–153.
- Karacan IH, Agnew H, Williams RL, Webb W, Ross J. Characteristics of sleep patterns during late pregnancy and postpartum periods. American Journal of Obstetrics and Gynecology. 1968; 297 (6656):1101–1102.
- Karacan I, Anch M, Thornby JI, Okawa M, Williams RL. Longitudinal sleep patterns during pubertal growth: Four-year follow up. Pediatrics Research. 1975; 9 (11):842–846. [ PubMed : 171619 ]
- Kobayashi R, Kohsaka M, Fukuda N, Honma H, Sakakibara S, Koyama T. Gender differences in the sleep of middle-aged individuals. Psychiatry and Clinical Neurosciences. 1998; 52 (2):186–187. [ PubMed : 9628142 ]
- Krieger J. Respiratory physiology: Breathing in normal subjects. In: Kryger M, Roth T, Dement WC, editors. Principles and Practice of Sleep Medicine. 4th ed. Philadelphia: Elsevier Saunders; 2000. pp. 229–241.
- Laberge L, Petit D, Simard C, Vitaro F, Tremblay RE, Montplaisir J. Development of sleep patterns in early adolescence. Journal of Sleep Research. 2001; 10 (1):59–67. [ PubMed : 11285056 ]
- Landolt HP, Dijk DJ, Achermann P, Borbely AA. Effect of age on the sleep EEG: Slowwave activity and spindle frequency activity in young and middle-aged men. Brain Research. 1996; 738 (2):205–212. [ PubMed : 8955514 ]
- Lee KA, Zaffke ME. Longitudinal changes in fatigue and energy during pregnancy and the postpartum period. Journal of Obstetric, Gynecologic, and Neonatal Nursing. 1999; 28 (2):183–191. [ PubMed : 10102546 ]
- Lee KA, Zaffke ME, Baratte-Beebe K. Restless legs syndrome and sleep disturbance during pregnancy: The role of folate and iron. Journal of Women’s Health and Gender-based Medicine. 2001; 10 (4):335–341. [ PubMed : 11445024 ]
- Leibenluft E, Fiero PL, Rubinow DR. Effects of the menstrual cycle on dependent variables in mood disorder research. Archives of General Psychiatry. 1994; 51 (10):761–781. [ PubMed : 7944869 ]
- Loomis AL, Harvey EN, Hobart GA. Cerebral states during sleep as studied by human brain potentials. Journal of Experimental Psychology. 1937; 21 (2):127–144.
- Lugaresi E, Coccagna G, Cirignotta F, Farneti P, Gallassi R, Di Donato G, Verucchi P. Breathing during sleep in man in normal and pathological conditions. Advances in Experimental Medicine and Biology. 1978; 99 :35–45. [ PubMed : 696501 ]
- Madsen PL, Holm S, Vorstrup S, Friberg L, Lassen NA, Wildschiodtz G. Human regional cerebral blood flow during rapid-eye-movement sleep. Journal of Cerebral Blood Flow Metabolism. 1991a; 11 (3):502–507. [ PubMed : 2016359 ]
- Madsen PL, Schmidt JF, Wildschiodtz G, Friberg L, Holm S, Vorstrup S, Lassen NA. Cerebral O 2 metabolism and cerebral blood flow in humans during deep and rapid-eye-movement sleep. Journal of Applied Physiology. 1991b; 70 (6):2597–2601. [ PubMed : 1885454 ]
- Meijer AM, Habekothe HT, Van Den Wittenboer GL. Time in bed, quality of sleep and school functioning of children. Journal of Sleep Research. 2000; 9 (2):145–153. [ PubMed : 10849241 ]
- Mercer PW, Merritt SL, Cowell JM. Differences in reported sleep need among adolescents. Journal of Adolescent Health. 1998; 23 (5):259–263. [ PubMed : 9814385 ]
- Metcalf MG. Incidence of ovulation from the menarche to the menopause: Observations of 622 New Zealand women. The New Zealand Medical Journal. 1983; 96 (738):645–648. [ PubMed : 6576257 ]
- Moe KE, Prinz PN, Vitiello MV, Marks AL, Larsen LH. Healthy elderly women and men have different entrained circadian temperature rhythms. Journal of the American Geriatrics Society. 1991; 39 (4):383–387. [ PubMed : 2010588 ]
- Monk TH, Buysse DJ, Reynolds CF III, Kupfer DJ, Houck PR. Circadian temperature rhythms of older people. Experimental Gerontology. 1995; 30 (5):455–474. [ PubMed : 8557094 ]
- Morruzzi G, Magoun HW. Brain stem reticular formation and activation of the EEG. Electroencephalography and Clinical Neurophysiology. 1949; 1 :455–473. [ PubMed : 18421835 ]
- Moss HA, Robson KS. The relation between the amount of time infants spend at various states and the development of visual behavior. Child Development. 1970; 41 (2):509–517. [ PubMed : 5431596 ]
- Mulcahy D, Wright C, Sparrow J, Cunningham D, Curcher D, Purcell H, Fox K. Heart rate and blood pressure consequences of an afternoon SIESTA (Snooze-Induced Excitation of Sympathetic Triggered Activity). American Journal of Cardiology. 1993; 71 (7):611–614. [ PubMed : 8438754 ]
- NHLBI (National Heart, Lung, and Blood Institute). Sleep, Sleep Disorders, and Biological Rhythms: NIH Curriculum Supplement Series, Grades 9-12. Colorado Springs, CO: Biological Sciences Curriculum Study; 2003.
- NLM (National Library of Medicine), NIH (National Institutes of Health). Medline Plus Online Medical Dictionary. [accessed February 6, 2006]. [Online]. Available: http://www .nlm.nih.gov /medlineplus/mplusdictionary.html .
- Parker KP, Dunbar SB. Cardiac nursing. In: Woods SL, Froelicher ESS, Motzer SU, Bridges E, editors. Sleep. 5th ed. Philadelphia: Lippincott Williams and Wilkins; 2005. pp. 197–219.
- Redline S, Kirchner HL, Quan SF, Gottlieb DJ, Kapur V, Newman A. The effects of age, sex, ethnicity, and sleep-disordered breathing on sleep architecture. Archives of Internal Medicine. 2004; 164 (4):406–418. [ PubMed : 14980992 ]
- Reynolds CF III, Kupfer DJ, Taska LS, Hoch CC, Sewitch DE, Spiker DG. Sleep of healthy seniors: A revisit. Sleep. 1985; 8 (1):20–29. [ PubMed : 3992105 ]
- Roffward HP, Muzio JN, Dement WC. Ontogenetic development of the human sleep-dream cycle. Science. 1966; 152 (3722):604–619. [ PubMed : 17779492 ]
- Sadeh A, Raviv A, Gruber R. Sleep patterns and sleep disruptions in school-age children. Developmental Psychology. 2000; 36 (3):291–301. [ PubMed : 10830974 ]
- Saper CB, Cano G, Scammell TE. Homeostatic, circadian, and emotional regulation of sleep. Journal of Comparitive Neurology. 2005a; 493 (1):92–98. [ PubMed : 16254994 ]
- Saper CB, Lu J, Chou TC, Gooley J. The hypothalamic integrator for circadian rhythms. Trends in Neuroscience. 2005b; 28 (3):152–157. [ PubMed : 15749169 ]
- Saper CB, Scammell TE, Lu J. Hypothalamic regulation of sleep and circadian rhythms. Nature. 2005c; 437 (7063):1257–1263. [ PubMed : 16251950 ]
- Sheldon SH. Sleep in infants and children. In: Lee-Choing TK, Sateia MJ, Carskadon MA, editors. Sleep Medicine. Philadelphia: Hanley and Belfus; 2002. pp. 99–103.
- Simon PM, Landry SH, Leifer JC. Respiratory control during sleep. In: Lee-Chiong TK, Sateia MJ, Carskadon MA, editors. Sleep Medicine. Philadelphia: Hanley and Belfus; 2002. pp. 41–51.
- Smith C, Lapp L. Increases in number of REMS and REM density in humans following an intensive learning period. Sleep. 1991; 14 (4):325–330. [ PubMed : 1947596 ]
- Somers V, Dyken M, Mark A, Abboud F. Sympathetic-nerve activity during sleep in normal subjects. New England Journal of Medicine. 1993; 328 (5):303–307. [ PubMed : 8419815 ]
- Szymusiak R. Sleep Research Society. SRS Basics of Sleep Guide. Westchester, IL: Sleep Research Society; 2005. Thermoregulation and sleep; pp. 119–126.
- Tank J, Diedrich A, Hale N, Niaz FE, Furlan R, Robertson RM, Mosqueda-Garcia R. Relationship between blood pressure, sleep K-complexes, and muscle sympathetic nerve activity in humans. American Journal of Physiology. Regulatory, Integrative and Comparative Physiology. 2003; 285 (1):R208–R214. [ PubMed : 12793998 ]
- Van Cauter E. Endocrine physiology. In: Kryger MH, Roth T, Dement WC, editors. Principles and Practice of Sleep Medicine. Philadelphia: Elsevier/Saunders; 2000. pp. 266–278.
- Vitaterna M, Pinto L, Turek F. Molecular genetic basis for mammalian circadian rhythms. In: Kryger MH, Roth T, Dement WC, editors. Principles and Practice of Sleep Medicine. 4th ed. Philadelphia: Elsevier/Saunders; 2005. pp. 363–374.
- Wolfson AR, Carskadon MA. Sleep schedules and daytime functioning in adolescents. Child Development. 1998; 69 (4):875–887. [ PubMed : 9768476 ]
- Wolfson AR, Carskadon MA, Acebo C, Seifer R, Fallone G, Labyak SE, Martin JL. Evidence for the validity of a sleep habits survey for adolescents. Sleep. 2003; 26 (2):213–216. [ PubMed : 12683482 ]
- Zepelin H, Siegel JM, Tobler I. Mammalian sleep. In: Kryger MH, Roth T, Dement WC, editors. Principles and Practice of Sleep Medicine. 4th ed. Philadelphia: Elsevier/Saunders; 2005. pp. 91–100.
- Cite this Page Institute of Medicine (US) Committee on Sleep Medicine and Research; Colten HR, Altevogt BM, editors. Sleep Disorders and Sleep Deprivation: An Unmet Public Health Problem. Washington (DC): National Academies Press (US); 2006. 2, Sleep Physiology.
- PDF version of this title (2.2M)
In this Page
Other titles in this collection.
- The National Academies Collection: Reports funded by National Institutes of Health
Related information
- PMC PubMed Central citations
- PubMed Links to PubMed
Recent Activity
- Sleep Physiology - Sleep Disorders and Sleep Deprivation Sleep Physiology - Sleep Disorders and Sleep Deprivation
Your browsing activity is empty.
Activity recording is turned off.
Turn recording back on
Connect with NLM
National Library of Medicine 8600 Rockville Pike Bethesda, MD 20894
Web Policies FOIA HHS Vulnerability Disclosure
Help Accessibility Careers
Thank you for visiting nature.com. You are using a browser version with limited support for CSS. To obtain the best experience, we recommend you use a more up to date browser (or turn off compatibility mode in Internet Explorer). In the meantime, to ensure continued support, we are displaying the site without styles and JavaScript.
- View all journals
- My Account Login
- Explore content
- About the journal
- Publish with us
- Sign up for alerts
- Open access
- Published: 19 July 2024
Sleep patterns and risk of chronic disease as measured by long-term monitoring with commercial wearable devices in the All of Us Research Program
- Neil S. Zheng 1 , 2 ,
- Jeffrey Annis 3 , 4 , 5 ,
- Hiral Master 3 , 4 ,
- Lide Han ORCID: orcid.org/0000-0002-0132-2656 4 , 6 ,
- Karla Gleichauf 7 ,
- Jack H. Ching ORCID: orcid.org/0000-0003-0752-8628 7 ,
- Melody Nasser 8 ,
- Peyton Coleman 4 , 9 ,
- Stacy Desine 3 ,
- Douglas M. Ruderfer ORCID: orcid.org/0000-0002-2365-386X 4 , 6 , 9 , 10 ,
- John Hernandez ORCID: orcid.org/0000-0002-3313-430X 7 ,
- Logan D. Schneider ORCID: orcid.org/0000-0001-8729-5224 7 , 11 &
- Evan L. Brittain ORCID: orcid.org/0000-0001-5843-3392 4 , 5
Nature Medicine volume 30 , pages 2648–2656 ( 2024 ) Cite this article
31k Accesses
3 Citations
672 Altmetric
Metrics details
- Epidemiology
- Lifestyle modification
Poor sleep health is associated with increased all-cause mortality and incidence of many chronic conditions. Previous studies have relied on cross-sectional and self-reported survey data or polysomnograms, which have limitations with respect to data granularity, sample size and longitudinal information. Here, using objectively measured, longitudinal sleep data from commercial wearable devices linked to electronic health record data from the All of Us Research Program, we show that sleep patterns, including sleep stages, duration and regularity, are associated with chronic disease incidence. Of the 6,785 participants included in this study, 71% were female, 84% self-identified as white and 71% had a college degree; the median age was 50.2 years (interquartile range = 35.7, 61.5) and the median sleep monitoring period was 4.5 years (2.5, 6.5). We found that rapid eye movement sleep and deep sleep were inversely associated with the odds of incident atrial fibrillation and that increased sleep irregularity was associated with increased odds of incident obesity, hyperlipidemia, hypertension, major depressive disorder and generalized anxiety disorder. Moreover, J-shaped associations were observed between average daily sleep duration and hypertension, major depressive disorder and generalized anxiety disorder. These findings show that sleep stages, duration and regularity are all important factors associated with chronic disease development and may inform evidence-based recommendations on healthy sleeping habits.
Similar content being viewed by others
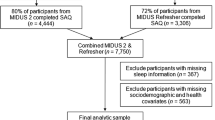
Sleep health composites are associated with the risk of heart disease across sex and race
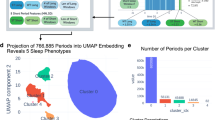
Five million nights: temporal dynamics in human sleep phenotypes
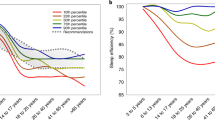
Sleep characteristics across the lifespan in 1.1 million people from the Netherlands, United Kingdom and United States: a systematic review and meta-analysis
Sleep health has been associated with all-cause mortality and chronic diseases, including psychiatric and cardiometabolic disorders 1 , 2 , 3 , 4 , 5 , 6 , 7 , 8 . Many previous studies have focused on sleep durations and have reported J-shaped associations, in which individuals with shorter (≤6 h) or longer (≥9 h) average daily sleep duration are at higher risk for a variety of poor health outcomes 1 , 2 , 6 . These studies have helped inform recommendations suggesting that approximately 7–9 h of daily sleep is appropriate for most adults 9 .
Less is known about the associations between chronic disease and other real-world sleep patterns, including sleep regularity and stages (for example, N1, N2, N3, rapid eye movement (REM)). Most epidemiologic studies on sleep and chronic diseases have relied on self-reported sleep data 1 , 2 , 3 , 4 , 5 , 6 , 7 . For example, several landmark epidemiologic studies, including the Nurses’ Health Study and the National Health and Nutrition Examination Survey, simply asked participants to estimate their typical sleep duration over a 24-h period 1 , 4 . Self-reported sleep data are unable to capture sleep stages and offer inaccurate representations of longitudinal sleep patterns. Studies using polysomnography rely on a limited number of nights of sleep data because of time and monetary constraints 10 , 11 . Other studies have used actigraphy-based sleep measures, but these studies rely on sleep data from 7 to 14 days of actigraphy data, which cannot capture longitudinal sleep patterns including seasonal or individual variations in sleep 8 , 12 . Newer ambulatory electroencephalographic devices have comparable performance to gold-standard polysomnograms 13 , but there have been no large-scale studies using these devices to date because of their limited use by the general population. Recent developments in commercial wearable devices, such as Fitbit, now enable objective longitudinal measurements of sleep patterns in the general population with good performance when compared with polysomnograms 14 , 15 , 16 , 17 , 18 , 19 , 20 , 21 . The growing popularity of these devices now makes possible large-scale epidemiological studies on associations between wearables-derived metrics and chronic diseases 22 , 23 , 24 , 25 .
The All of Us Research Program (AoU) is a National Institutes of Health-funded initiative to gather health data from more than one million diverse persons living in the United States 26 , 27 . Participants are enrolled digitally and invited to share multiple longitudinal sources of health-related information, including electronic health records (EHRs), genomics, physical measures and participant surveys 26 . Participants who owned Fitbit devices were invited to voluntarily share their Fitbit data, including physical activity and sleep patterns with a median monitoring time of 4.5 years. By connecting Fitbit data with EHRs, the AoU has made it possible, for the first time, to perform large-scale, longitudinal studies of objectively measured physical activity and sleep patterns in association with clinical outcomes data 22 .
In this study, we leveraged the longitudinal EHR data and daily sleep patterns in the AoU to investigate associations between sleep patterns over time and incident chronic disease. Our approach included both phenome-wide association studies (PheWAS) aimed at discovery and targeted assessment of specific chronic diseases associated with poor sleep with findings relevant to the general US population.
We identified 14,892 individuals with Fitbit-derived sleep data. A total of 6,785 adult participants with linked EHR data, a Fitbit monitoring period of at least 6 months and fewer than 30% of nights with <4 h of sleep were included for analysis, resulting in 6,477,023 person-nights (Extended Data Fig. 1 ). The median age was 50.2 years (interquartile range = 35.7, 61.5) (Table 1 ). Most participants were female (71%), white (84%) and college educated (71%). The median Fitbit monitoring length was 4.49 (2.53, 6.45) years and median average daily step count was 7,798 (5,898, 9,947) steps per day. Median sleep patterns were 11:10 p.m. (10:30, 00:00) for sleep onset time (Fig. 1 ), 6.7 (6.2, 7.2) h for sleep duration, 0.3 (0.2, 0.5) h for restless sleep duration and 1.5 (1.2, 1.8) for sleep irregularity (standard deviation (s.d.) of average daily sleep duration in hours). The median proportion of weekdays with sleep onset during ‘traditional’ hours (8:00 p.m. to 2:00 a.m.) was 93.5% (85.2, 97.3). For sleep stages, the median percentage spent in REM, light and deep sleep were 20.7% (17.8, 23.1), 64.2% (60.3, 68.5) and 15.1% (12.7, 17.5), respectively. Population median for Fitbit-derived sleep metrics remained relatively stable from 2017 to 2022 (Extended Data Fig. 2 ). There were significant differences in median sleep duration when stratified by participant demographics (self-reported sex, self-reported race/ethnicity, education) and lifestyle factors (smoking, alcohol intake) (Table 1 ).
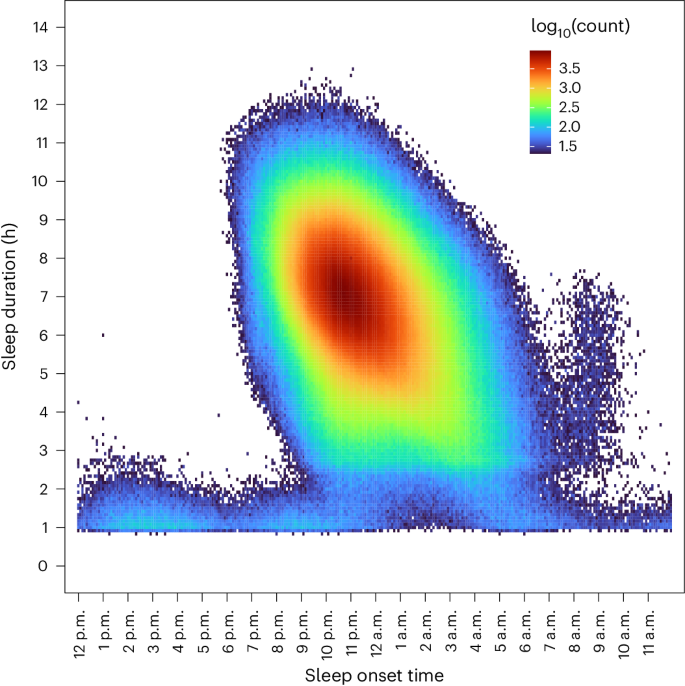
Heatmap of sleep duration is plotted versus sleep onset time across all person-nights. All sleep periods were flagged as ‘main sleep’ by Fitbit devices.
Association of sleep patterns with incident disease
Discovery-focused PheWAS identified 48 significant associations with Fitbit-derived sleep patterns after Bonferroni correction (Fig. 2 ), including 2 associations for sleep duration, 3 for restless sleep duration, 14 for sleep stages, 24 for sleep irregularity and 5 for traditional versus nontraditional sleep onset. There were several phenotypes with significant associations across multiple sleep patterns, such as the association between insomnia and average restless sleep duration, sleep irregularity and traditional sleep onset proportion (Fig. 3 ).
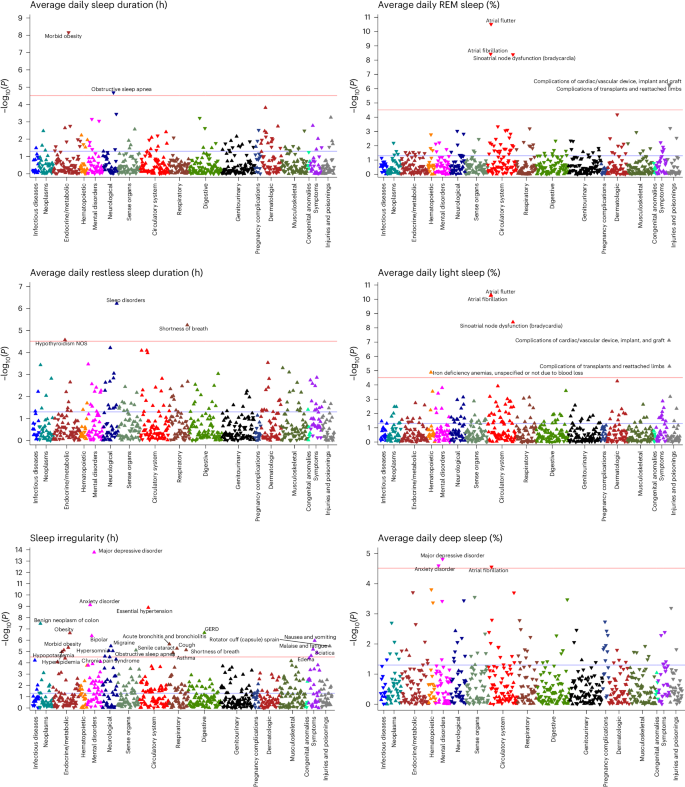
Phenome-wide analyses were performed to identify associations between each Fitbit-derived sleep metric and incident disease. All phenome-wide analyses were performed using multiple logistic regression models adjusted for age, sex, average daily step count across the entire monitoring period and EHR length. All reported P values were based on two-tailed probability. The Bonferroni significance line of 3.06 × 10 − 5 is indicated by a red line and a P value of 0.05 is indicated by the blue line. Upwards pointing triangles indicate OR >1 and downwards pointing triangles indicated OR <1. GERD, gastroesophageal reflux disease; NOS, not otherwise specified.
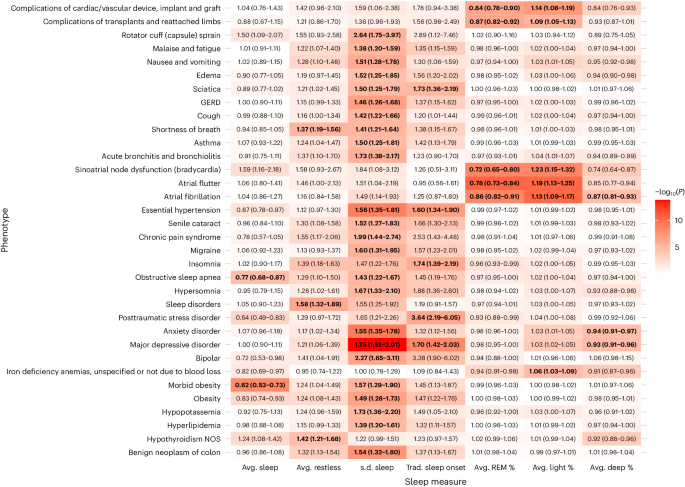
Heatmap of −log 10 ( P ) is overlaid on a table of significant associations between all incident phenotypes and Fitbit-derived sleep metrics. OR values (95% CI) are reported within each heatmap table box. OR values for average (avg.) sleep, average restless and s.d. of sleep duration are reported per hour change. OR values for average percent sleep stage are reported per 10% change. All reported ORs are from multiple logistic regression models adjusted for age, sex, average daily step count across the entire monitoring period and EHR length. Boxes that include bold text indicate associations that were significant after Bonferroni correction line of 3.06 × 10 −5 . All reported P values were based on two-tailed probability. Trad., traditional.
Each hour increase in average daily sleep duration was associated with decreased odds of receiving a new diagnosis for morbid obesity (odds ratio (OR) = 0.62; 95% confidence interval (CI) = 0.53–0.73) and obstructive sleep apnea (0.77; 0.68–0.87). Increased average daily restless sleep (per hour) was associated with increased odds of sleep disorders (1.58; 1.32–1.89), hypothyroidism (1.42; 1.21–1.68) and shortness of breath (1.37; 1.19–1.56).
Increased sleep irregularity (per hour change in s.d. of daily sleep duration) was associated with a variety of incident psychiatric, sleep and metabolic disorders. Chronic conditions associated with increased sleep irregularity included essential hypertension (1.56; 1.35–1.81), hyperlipidemia (1.39; 1.20–1.61) and obesity (1.49; 1.28–1.73). We also observed increased odds of several psychiatric disorders, including major depressive disorder (1.75; 1.52–2.01), anxiety disorder (1.55; 1.35–1.78) and bipolar disorder (2.27; 1.65–3.11). In addition, increased sleep irregularity was associated with conditions that may disrupt sleep, including gastroesophageal reflux disease (1.46; 1.26–1.68), obstructive sleep apnea (1.43; 1.22–1.67), asthma (1.50; 1.25–1.81) and migraine (1.60; 1.31–1.95). The majority (23 of 24) of PheWAS associations for sleep irregularity were still significant after adjustment for average daily sleep duration (Extended Data Table 1 ).
When examining Fitbit-derived sleep stages, each percent increase in REM sleep was associated with a reduced incidence of heart rhythm and rate abnormalities, including atrial fibrillation (OR 0.86; 95% CI 0.82–0.91), atrial flutter (0.78; 0.73–0.84) and sinoatrial node dysfunction/bradycardia (0.72; 0.65–0.80). Higher light sleep percentage (per percent) was associated with increased odds of atrial fibrillation (1.13; 1.09–1.17), atrial flutter (1.19; 1.13–1.25), sinoatrial node dysfunction/bradycardia (1.23; 1.15–1.32) and iron deficiency anemia (1.06; 1.03–1.09). Higher deep sleep percentage (per percent) was also associated with lower odds of atrial fibrillation (0.87; 0.81–0.93), major depressive disorder (0.93; 0.91–0.96) and anxiety disorder (0.94; 0.91–0.97).
When comparing participants with sleep onset proportion greater and less than the median (93.5%), participants who had lower proportions of traditional sleep onset had higher odds for incident major depressive disorder (1.70; 1.42–2.03), post-traumatic stress disorder (3.64; 2.19–6.05), insomnia (1.74; 1.39–2.19), essential hypertension (1.60; 1.34–1.90) and sciatica (1.73; 1.36–2.19) (Extended Data Fig. 3 ). The association for sciatica was no longer significant after adjusting for average daily sleep duration. Every 10% decrease in the proportion of days with sleep onset within the ‘traditional’ time window was associated with a 19% and 37% increase in odds of hypersomnia (1.19; 1.10–1.27) and circadian rhythm sleep disorders (1.37; 1.20–1.56), respectively.

Time-varying analysis of sleep patterns and chronic disease
We performed Cox proportional hazard analyses for chronic diseases selected a priori from reported associations in previous studies (Table 2 ) 1 , 2 , 3 , 4 , 5 , 6 , 7 , 28 . Increased average sleep duration was associated with lower risk for obesity (hazard ratio (HR) = 0.90; 95% CI = 0.83–0.98), whereas increased sleep irregularity was associated with increased risk of obesity (1.21; 1.08–1.37). Increased REM sleep percentage was associated with decreased risk of incident heart failure (HR 0.51; 95% 0.26–0.99) and generalized anxiety disorder (0.80; 0.69–0.92). Increased light sleep percentage was associated with increased risk of incident heart failure (2.30; 1.05–5.04), generalized anxiety disorder (1.31; 1.13–1.52) and atrial fibrillation (1.76; 1.02–3.05). Increased deep sleep percentage was associated with decreased risk of atrial fibrillation (0.59; 0.35–0.99) and generalized anxiety disorder (0.84; 0.72–0.98).
In stratified analyses of the significant associations (Extended Data Fig. 4 ), we observed that sleep duration and sleep irregularity were most associated with risk for obesity in participants who were younger than 50 years, male, white or lived in the Northeast (versus West, South, Midwest). Increased Fitbit-derived deep or light sleep percentages were most associated with risk of generalized anxiety disorder in participants who were older than 50 years, female, white or lived in the Northeast. Increased REM sleep percentage was associated with reduced risk of generalized anxiety disorder in participants who were younger than 50 years, female, white and lived in the Northeast. Stratified analyses for associations between Fitbit-derived sleep stages and incident atrial fibrillation or heart failure showed no major differences, albeit several of the stratum had fewer than 20 cases and could not be reported because of AoU data and statistics dissemination policies.
We identified significant nonlinear, J-shaped relationships between average daily sleep duration and hypertension ( P for nonlinearity = 0.003), major depressive disorder ( P < 0.001) and generalized anxiety disorder ( P < 0.001) (Fig. 4 ). Compared with the median of average daily sleep (6.8 h), participants who had an average daily sleep duration of 5 h had 29% increased risk of hypertension (HR = 1.29; 95% CI = 1.09–1.54), 64% increased risk of major depressive disorder (1.64; 1.27–2.12) and 46% increased risk of generalized anxiety disorder (1.46; 1.16–1.83) (Extended Data Table 2 ). Participants with an average daily sleep duration of 10 h had 61% increased risk of hypertension (1.61; 1.01–2.58), 163% increased risk of major depressive disorder (2.63; 1.31–5.31) and 130% increased risk of generalized anxiety disorder (2.30; 1.27–4.17).
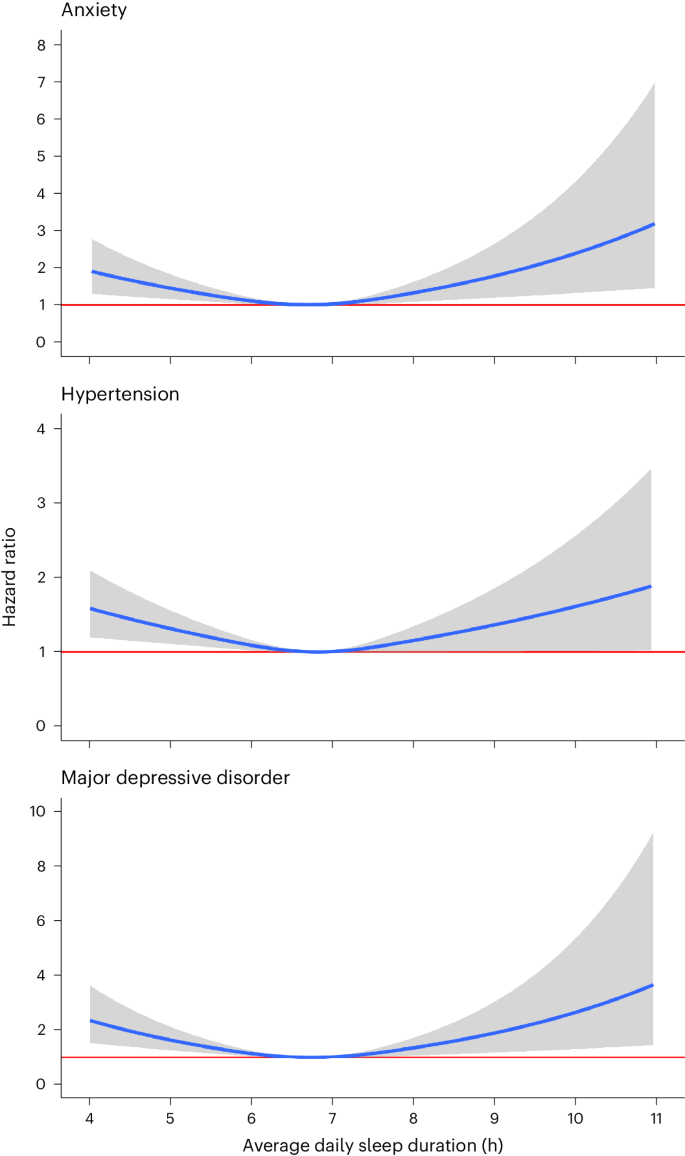
Cox proportional hazard models were used to compute HR values as a function of average daily sleep duration and plotted as blue curves for generalized anxiety disorder, hypertension and major depressive disorder. The median of average daily sleep (6.8 h) was used as the reference. Gray area indicates 95% CI. The red horizontal line indicates an HR of 1. All Cox proportional hazards models were adjusted for age, sex, baseline BMI, baseline systolic blood pressure, smoking status, alcohol drinking status, education status, time-varying average daily step count and previous diagnoses of cancer or coronary artery disease.
Sensitivity analysis for sleep apnea
Previous studies have shown that obstructive sleep apnea is highly associated with atrial fibrillation and several cardiometabolic disorders, including obesity, diabetes and hypertension 29 , 30 . To assess the influence of sleep apnea in our findings, we showed that a majority (27 of 41) of reported PheWAS associations were still significant after adjustment for previous diagnoses of sleep apnea (Extended Data Table 3 ). Increased REM sleep percentage remained associated with lower odds of atrial fibrillation and increased sleep irregularity remained associated with increased odds of several psychiatric (major depressive disorder, bipolar disorder, generalized anxiety disorder) and cardiometabolic disorders (obesity, essential hypertension). There remained an inverse association between deep sleep percentage and atrial fibrillation (OR = 0.86; 95% CI = 0.81–0.93; P = 3.98 × 10 −5 ), but the association did not reach the Bonferroni significance threshold of 3.06 × 10 −5 .
After adjusting the Cox proportional hazard models for previous diagnosis of sleep apnea, a majority (8 of 9) of the reported associations remained significant (Extended Data Table 4 ). In addition, three of the Fitbit-derived sleep metrics were now significantly associated with obesity after adjustment for sleep apnea, including average restless sleep duration (HR = 1.14; CI = 1.01–1.29), average REM sleep percentage (1.12; 1.01–1.23) and average light sleep percentage (0.88; 0.79–0.97). The inverse association between deep sleep percentage and atrial fibrillation remained significant (0.59; 0.35–0.99).
Sensitivity analysis for atrial fibrillation
It is possible that the atrial fibrillation associations we observed were an artifact of the Fitbit algorithm, which assigns sleep stages based, in part, on heart rate. The Fitbit devices used by AoU participants do not report heart rate irregularity. Therefore, to examine the potential for atrial fibrillation during sleep, we calculated and compared heart rate variability (standard deviation of average normal-to-normal intervals (SDANN)) among participants with and without atrial fibrillation during sleep and wake times. Higher heart rate variability may suggest a higher preponderance of atrial fibrillation during sleep. There were no significant differences in heart rate or heart rate variability during sleep or wake times between participants with atrial fibrillation and matched controls without atrial fibrillation. However, we did observe lower SDANN during REM (median 3.6 versus 3.8; P = 0.021) and deep sleep (3.1 versus 3.2; P = 0.019) among those with atrial fibrillation (Extended Data Table 5 ). In addition, participants with atrial fibrillation were more likely to have prevalent cardiometabolic disease, including coronary artery disease (30% versus 11%; P < 0.001) and obesity (39% versus 30%; P = 0.014).
We conducted the largest study to date that analyzes sleep patterns objectively and longitudinally across many years using direct measures of sleep from commercial wearable devices linked to EHR data. We observed clinically and statistically significant relationships between sleep quantity, quality and regularity and the onset of important chronic diseases even after accounting for daily activity (that is, step counts).
Our discovery analyses demonstrated several expected findings, such as the association between increased restless sleep duration and increased odds of incident sleep disorders or insomnia. Similarly, participants who had fewer sleep onset times between 8:00 p.m. and 2:00 a.m. had higher odds of incident sleep disorders, including insomnia, hypersomnia and circadian rhythm disorders. In addition, many of our findings are supported by previous studies using large-scale population surveys, such as our observations that decreased daily sleep duration and increased sleep irregularity are associated with obesity and sleep apnea 4 , 7 . Daily average sleep duration had nonlinear, J-shaped associations with hypertension, major depressive disorder and generalized anxiety disorder, a well-documented phenomenon reported in previous studies 1 , 2 , 3 , 4 , 5 , 6 , 31 , 32 . Our findings, along with those from previous epidemiologic studies, support the notion that 7 h of objectively measured sleep may be the middle of the healthy range for adults rather than the floor 33 , highlighting that the range of healthy sleep depends on the tool used to measure sleep.
Sleep irregularity has been difficult to study because of the limitations of data derived from cross-sectional surveys or polysomnograms and the infeasibility of obtaining objective measurements in the general population before the availability of modern consumer wearables. A 2023 study showed that sleep irregularity was associated with all-cause mortality in the UK Biobank, a large biobank linked to EHRs in the United Kingdom 8 . However, in that study, sleep patterns were only monitored for a 7-day period and were derived from wearable actigraphy data, which is less accurate than Fitbit objective measures of sleep 8 , 17 , 18 , 19 , 21 . Another study demonstrated associations between sleep irregularity and hypertension using data collected across 9 months with under-the-bed monitors 31 . Our study showed similar findings using a larger sample of person-nights and identified many additional associations across the phenome that are consistent with previous cross-sectional studies 12 , 25 , 34 , 35 . Furthermore, we showed that a lower proportion of ‘traditional’ sleep onset times was associated with hypertension and several psychiatric disorders. Notably, there were more associations for sleep irregularity than any other sleep pattern included in our study. One possible explanation could be that sleep irregularity can contribute to desynchronization of sleep–wake cycles and circadian rhythms 36 . Circadian rhythm disruptions are linked to a number of adverse health outcomes and have negative effects on human physiology, such as reduced insulin sensitivity, increased inflammation and downregulation of serotonin receptors 37 . We also observed that the majority of associations related to sleep irregularity were still significant after adjusting for average daily sleep duration, suggesting that sleep irregularity is an independent risk factor and highlighting the importance of longitudinal sleep monitoring for healthy sleep patterns.
There is conflicting evidence regarding the connection between REM or deep sleep and atrial fibrillation. In the Multi-Ethnic Study of Atherosclerosis cohort ( N = 2,048), longer polysomnographic slow-wave (deep) sleep was associated with decreased atrial fibrillation prevalence but REM sleep was not 38 . By contrast, the Health eHeart study ( N = 1,127) found that longer REM sleep was associated with decreased atrial fibrillation prevalence but longer deep sleep was not 39 . As the largest of these studies and the only one with longitudinal data, we found that increased Fitbit-derived REM and deep sleep duration were both associated with decreased risk of incident atrial fibrillation in our discovery analyses but only the deep sleep association was significant in time-varying analyses. Sleep stages may be linked to atrial fibrillation via their influence on signaling from the autonomic nervous system to the heart. The sympathetic and parasympathetic nervous systems are both involved in the development of atrial fibrillation 40 . Sympathetic activity is high during REM sleep, whereas parasympathetic activity is high during deep sleep 41 . It is possible that decreased REM or deep sleep may lead to abnormal autonomic nervous system signaling to the heart, increasing the risk of atrial fibrillation. However, it is important to acknowledge that Fitbit identifies sleep stages by monitoring heart rate and movement, which may be affected in participants with atrial fibrillation or cardiac conduction and cardiovascular abnormalities. In our study, there was a higher prevalence of coronary artery disease and obesity in participants with atrial fibrillation, even after matching for age, sex and baseline body mass index (BMI). Our findings that decreased proportions of REM or deep sleep are associated with incident atrial fibrillation may instead be identifying markers for occult atrial fibrillation and/or poor overall cardiovascular health. More longitudinal studies are needed to elucidate the relationship between Fitbit-derived sleep stages and atrial fibrillation. If confirmed, these findings have the potential to inform clinical guidance on sleep hygiene to reduce atrial fibrillation incidence or recurrence in participants at high risk.
Previous studies have shown that obstructive sleep apnea is highly prevalent among patients with atrial fibrillation and several cardiometabolic disorders, including obesity, diabetes and hypertension 29 , 30 . We observed that the majority of our reported findings for Fitbit-derived sleep metrics remained significant after adjusting for previous diagnosis of sleep apnea in both the discovery-focused PheWAS and time-varying analyses, suggesting that sleep patterns are risk factors for chronic disease independent of sleep apnea.
There are several limitations to this study. First, the study cohort is relatively young, majority female, white and college educated. The generalizability of the findings to underrepresented communities or those in areas of deprivation is unclear and, thus, a high priority for future studies. Notably, there is an active effort within the AoU Research Program to expand the diversity of participants with Fitbit data through the WEAR study by providing free Fitbit devices to invited participants from underrepresented communities. Nonetheless, many of our findings are supported by previous studies using diverse populations with survey or polysomnogram data. In addition, our findings will be highly relevant to the increasing proportion of the general US population that owns a commercial wearable device, which reached nearly 30% in 2020 42 . Moreover, because the owners of commercial wearable devices are broadly healthier than the general population, the reported effect sizes and impact of poor sleep health in this study may actually be stronger in the general population 22 . Second, the sleep data included in our analyses are reported from and calculated by Fitbit. The Fitbit algorithms have been evaluated against gold-standard polysomnograms in many studies 14 , 15 , 16 , 17 , 18 , 19 , 20 , 43 , 44 . The largest of these validation studies showed that Fitbit did not significantly differ in estimation of total sleep time or deep sleep compared with polysomnograms, but underestimated REM sleep by 11.4 min (ref. 18 ). Therefore, our findings may not generalize to sleep data from non-Fitbit sources because of potential systematic misestimation of sleep stage proportions 14 , 15 , 16 , 17 , 18 , 19 , 20 , 43 , 44 . Although there may be inherent biases in Fitbit’s estimation of sleep stages compared with polysomnograms, the Fitbit algorithm has not been changed since launching in 2017. Therefore, such biases would likely manifest in the same way in the same individual over time. Nonetheless, our findings are consistent with those from studies using polysomnograms, highlighting that Fitbit devices still meaningfully capture the fundamental aspects of sleep as it relates to health and disease despite modest reductions in performance compared with polysomnograms. It is also important to consider that polysomnogram-assigned sleep stages are limited by high inter- and intra-rater variability 45 , 46 . Third, we cannot exclude the possibility that our findings are the result of reverse causation. We focused on incident diseases after the first 180 days of the sleep monitoring period to mitigate this risk. It is also possible that sleep disturbances may be an early indicator for some conditions identified in the analysis, such as obstructive sleep apnea, gastroesophageal reflux disease or asthma. Fourth, there were some associations identified in the PheWAS that did not achieve significance in the time-varying analyses. This may be due to various aspects including the characteristics of the statistical model, available covariates and the population sample analyzed. Fifth, we acknowledge the limitations of using diagnosis codes from EHRs as outcomes and the possibility of misclassification. Lastly, there are other potential confounders that we are unable to account for because of the nature of the AoU data resource and individual patterns of commercial wearable devices usage, such as the variability in participants’ occupations.
Despite these limitations, the unique features of our study design and data sources set it apart from previous studies, yielding results that are both novel and clinically relevant. To our knowledge, this is the largest study to objectively analyze sleep patterns longitudinally across many years using direct measures of sleep. Using data from commercial wearable devices, we were able to assess detailed sleep patterns across nearly 6.5 million person-nights, which was previously not possible with surveys or was prohibitively expensive with polysomnograms. Previous studies have often focused on a narrow set of phenotypes, whereas we are able to analyze associations across 1,636 diverse phenotypes because of the AoU’s linkage of sleep data with EHR data. This study also includes time-varying analyses, which account for changes in sleep behavior over time, unlike previous cross-sectional studies. Finally, our study also accounts for the impact of sleep on disease risk accounting for concomitant, longitudinal activity behavior, which is novel compared with other studies and shows the independent impact of sleep.
Our study helps advance the current understanding on the relationship between behavior and health and has important clinical and public health implications. Wearable devices with sleep monitoring capabilities are becoming increasingly popular. Our results, which are based on sleep patterns directly reported to consumers, will be highly relevant for participants monitoring their sleep and for providers counseling on healthy sleep habits. The integration of patient-generated sleep data with EHRs in routine care in the future could enable providers to monitor changes in sleep patterns as early disease indicators and to provide evidence-based guidance tailored to an individual’s unique clinical circumstances and risk profile.
In summary, we show that insufficient sleep quantity, quality and regularity are all associated with increased incidence of numerous chronic diseases, including obesity, atrial fibrillation, hypertension, major depressive disorder and generalized anxiety disorder. These findings, if validated, may provide evidence for updated recommendations on healthy sleeping habits, especially in individuals at high risk for chronic conditions. Furthermore, our study supports the value of integrating data from commercial wearable device with EHRs to advance scientific discoveries and to improve patient care.
Study participants
All study participants consented to participate in the All of Us Research Program, which has been described at length elsewhere 26 , 27 . Briefly, all adults (≥18 years) in the United States are eligible to enroll in the AoU research programs, except for individuals who are in prison or are unable to consent on their own. For this study, we used registered and controlled tier data (C2022Q4R9) available on the AoU Researcher Workbench. Controlled tier data were used only for analyses that required absolute dates. Data from 413,457 individuals who were enrolled from May 2018 to July 2022 were available at the time of analysis. Detailed information was collected, including participant demographics and survey data during the digital enrollment, physical measurements and vital signs measured at a partnered healthcare provider organization, voluntarily shared EHR data from partnered healthcare provider organization, and voluntarily shared Fitbit device data by linking their Google Fitbit account in the AoU participant portal 26 , 27 , 47 .
Fitbit sleep data
We used the Fitbit-derived daily summary data that are displayed to consumers, including daily duration of sleep time, restless sleep time (defined by Fitbit as sleep with movement but not indicating wakefulness) and Fitbit-derived sleep stages (light, deep, REM). Fitbit estimates sleep stages using a proprietary algorithm based on heart rate and movement and only estimates sleep stages for sleep periods of duration >3 h (ref. 43 ). Based on algorithm validation studies, Fitbit maps ‘light’ sleep to N1 + N2, ‘deep’ sleep to N3, and ‘REM’ to rapid eye movement sleep 43 .
For the discovery analyses, we averaged daily sleep patterns for each participant across their entire monitoring period. Only sleep periods with available Fitbit-derived sleep stage data were used to estimate average daily sleep stages. The Fitbit monitoring period began when the participant created a Fitbit account, not on the date of enrollment in AoU. Therefore, the initiation of monitoring precedes AoU enrollment in participants. We defined sleep irregularity as the s.d. of the daily sleep duration. We calculated a daily percentage (time in sleep stage/total sleep time) for each sleep stage. To characterize typical sleep schedule patterns, we calculated the proportion of sleep onset during ‘traditional’ times, defined as between 8:00 p.m. and 2:00 a.m., which was chosen because the median sleep onset in our cohort was 11:10 p.m. and a large proportion of sleep onset times were within this timeframe when plotted on a heatmap (Fig. 1 ); weekends were excluded from the analysis of ‘traditional’ sleep onset times because of their low representation of typical sleep schedules.
Quality control
We included adult (≥18 years) participants with EHR data and at least six months of Fitbit sleep data from the start to the end of each participant’s Fitbit monitoring period. To enrich for consistent device wearers and ensure our data provided an accurate reflection of typical and realistic sleep patterns, we excluded participants who had <4 h of sleep data on ≥30% of days. These thresholds were informed by mathematical models as the theoretical cutoff at which insufficient sleep would be unsustainable 48 . We only included sleep periods that were flagged as the ‘main sleep’ (that is, longest sleep per day) by the Fitbit algorithm. We also only included data from dates for which both step and sleep data were available to align both variables for time-varying analyses.
Study outcomes and covariates
The primary outcomes were incident diagnoses coded in the EHR. We excluded incident diagnoses in the first 180 days of Fitbit monitoring to reduce the risk of reverse causation, assuming those conditions already existed but were not yet reflected in billing codes. Incident diagnoses were identified by International Classification of Diseases billing codes and mapped to 1,636 phecodes 49 , 50 , 51 . Phecodes consolidate similar International Classification of Diseases codes to reduce collinearity and multiple comparisons. Nonspecific phecodes (for example, 512.9 = Other dyspnea) were not reported. Phecodes with ≤20 cases were not reported to comply with AoU Data and Statistics dissemination policies.
Participant demographics (age, self-reported sex, self-reported race/ethnicity, education) and lifestyle factors (smoking, alcohol intake) were derived from survey data completed at the time of enrollment. Average daily step count was derived from the Fitbit physical activity data, as previously described 22 . We calculated total EHR length as the time between the first and last billing code (diagnosis or procedure), vital sign documentation or laboratory measurement in the participant’s EHR.
Statistical analyses
Discovery-focused PheWAS were performed for each sleep pattern averaged across the entire Fitbit monitoring period using multiple logistic regression models. For the analysis of a given disease of interest, we excluded participants with any previous diagnosis of that particular disease of interest before Fitbit monitoring or any incident diagnoses of that particular disease of interest within the initial 180 days of Fitbit monitoring to mitigate the risk for reverse causation. After Bonferroni correction for multiple comparisons, the significance threshold was α < 0.05/1,636 = 3.06 × 10 −5 . PheWAS analyses were adjusted for age, sex, average daily step count across the entire monitoring period and EHR length.
We performed Cox proportional hazards regression models for incident phecodes by sleep pattern for chronic disease phenotypes of interest, selected a priori from reported associations in previous studies 1 , 2 , 3 , 4 , 5 , 6 , 7 , 28 . We excluded participants with any previous diagnosis of the chronic disease phenotype before Fitbit monitoring or any incident diagnoses within the initial 180 days of Fitbit monitoring to mitigate the risk for reverse causation. HR values and 95% CI were calculated comparing the 75th and 25th percentile of each sleep pattern. Participants were censored at their last medical encounter, which was defined as their last billing code, recorded vital sign or laboratory measurement. We established the ‘baseline’ sleep patterns and steps counts as the average across the initial 180 days of Fitbit monitoring for each individual. Participants with ≤15 days of monitoring in the ‘baseline’ period were excluded from the models for average sleep duration and sleep irregularity, but those individuals were included in the restless sleep duration and sleep stages models to increase the sample size because not all Fitbit devices report restless sleep duration or sleep stages. Sleep patterns and step counts were averaged on a monthly basis starting from the last date of the 180-day ‘baseline’ period and extending to the date of censor/incident diagnosis. The monthly averages were entered into the Cox models as time-varying variables. Months with ≤15 days of observations were excluded. Wald χ 2 tests were used to assess for nonlinear relationships between sleep patterns and chronic diseases. We calculated HRs as a function of each sleep metric when compared with the median of that sleep metric in the filtered cohort. All Cox models were adjusted for age, sex, baseline BMI, baseline systolic blood pressure, smoking status, alcohol drinking status, education status, time-varying average daily step count and previous diagnoses of cancer or coronary artery disease. Missing data for covariates were imputed using multiple imputation with predictive mean matching. Continuous variables were modeled as restricted cubic splines with 3-knots.
Statistical analyses were performed in R (v.4.2.2, R Project https://www.r-project.org ) on the AoU Researcher Workbench, a secure cloud-based platform. Statistical tests were based on two-tailed probability.
Sleep apnea sensitivity analyses
Sensitivity analyses were performed to assess for the influence of sleep apnea on our findings. Discovery PheWAS and Cox proportional hazard regression models were repeated for each Fitbit-derived sleep metric as described above with an additional covariate for previous diagnosis of sleep apnea.
Sleep stage-specific sensitivity analyses
Analyses showing an association between sleep stage and atrial fibrillation were subjected to a sensitivity analysis because of concern for either active atrial fibrillation or algorithm-based misclassification driving the association 43 . We compared heart rate variability (SDANN) between participants with previous diagnosis of atrial fibrillation (excluding those with pacemakers) and matched controls without previous diagnosis of atrial fibrillation. Average minute-level heart rate was computed for consecutive 5-min intervals over a 24-h period (00:00 to 23:59). The average RR interval duration was inferred by dividing each average heart rate by 1/6,000. The SDANN was then estimated by taking the s.d. across all average RR interval durations for the entire day 52 . Controls were matched on age, sex and nearest BMI measurement to baseline using Mahalanobis distance matching.
Reporting summary
Further information on research design is available in the Nature Portfolio Reporting Summary linked to this article.
Data availability
To ensure privacy of participants, data used for this study are available to approved researchers following registration, completion of ethics training and attestation of a data use agreement through the All of Us Research Workbench platform, which can be accessed via https://workbench.researchallofus.org/ .
Code availability
Code used for this study can be made immediately available to any approved researchers on the All of Us Research Workbench platform by contacting our study team.
Ayas, N. T. et al. A prospective study of sleep duration and coronary heart disease in women. Arch. Intern. Med. 163 , 205–209 (2003).
Article PubMed Google Scholar
Cappuccio, F. P., D’Elia, L., Strazzullo, P. & Miller, M. A. Quantity and quality of sleep and incidence of type 2 diabetes: a systematic review and meta-analysis. Diabetes Care 33 , 414–420 (2010).
Gangwisch, J. E. et al. Short sleep duration as a risk factor for hypertension: analyses of the first National Health and Nutrition Examination Survey. Hypertension 47 , 833–839 (2006).
Article PubMed CAS Google Scholar
Gangwisch, J. E., Malaspina, D., Boden-Albala, B. & Heymsfield, S. B. Inadequate sleep as a risk factor for obesity: analyses of the NHANES I. Sleep 28 , 1289–1296 (2005).
von Ruesten, A., Weikert, C., Fietze, I. & Boeing, H. Association of sleep duration with chronic diseases in the European Prospective Investigation into Cancer and Nutrition (EPIC)-Potsdam study. PLoS ONE 7 , e30972 (2012).
Article Google Scholar
Cappuccio, F. P., D’Elia, L., Strazzullo, P. & Miller, M. A. Sleep duration and all-cause mortality: a systematic review and meta-analysis of prospective studies. Sleep 33 , 585–592 (2010).
Article PubMed PubMed Central Google Scholar
Foley, D., Ancoli-Israel, S., Britz, P. & Walsh, J. Sleep disturbances and chronic disease in older adults: results of the 2003 National Sleep Foundation Sleep in America Survey. J. Psychosom. Res. 56 , 497–502 (2004).
Cribb, L. et al. Sleep regularity and mortality: a prospective analysis in the UK Biobank. eLife 12 , RP88359 (2023).
Article PubMed PubMed Central CAS Google Scholar
Watson, N. F. et al. Recommended amount of sleep for a healthy adult: a joint consensus statement of the American Academy of Sleep Medicine and Sleep Research Society. Sleep 38 , 843–844 (2015).
PubMed PubMed Central Google Scholar
Quan, S. F. et al. The Sleep Heart Health Study: design, rationale, and methods. Sleep 20 , 1077–1085 (1997).
PubMed CAS Google Scholar
Moon, C., Hagen, E. W., Johnson, H. M., Brown, R. L. & Peppard, P. E. Longitudinal sleep characteristics and hypertension status: results from the Wisconsin Sleep Cohort Study. J. Hypertens. 39 , 683–691 (2021).
Full, K. M. et al. Sleep irregularity and subclinical markers of cardiovascular disease: the multi-ethnic study of atherosclerosis. J. Am. Heart Assoc. 12 , e027361 (2023).
Arnal, P. J. et al. The Dreem Headband compared to polysomnography for electroencephalographic signal acquisition and sleep staging. Sleep 43 , zsaa097 (2020).
de Zambotti, M., Goldstone, A., Claudatos, S., Colrain, I. M. & Baker, F. C. A validation study of Fitbit Charge 2 compared with polysomnography in adults. Chronobiol. Int. 35 , 465–476 (2018).
Lee, X. K. et al. Validation of a consumer sleep wearable device with actigraphy and polysomnography in adolescents across sleep opportunity manipulations. J. Clin. Sleep. Med. 15 , 1337–1346 (2019).
Stucky, B. et al. Validation of Fitbit Charge 2 sleep and heart rate estimates against polysomnographic measures in shift workers: naturalistic study. J. Med. Internet Res. 23 , e26476 (2021).
Eylon, G., Tikotzky, L. & Dinstein, I. Performance evaluation of Fitbit Charge 3 and actigraphy vs. polysomnography: sensitivity, specificity, and reliability across participants and nights. Sleep Health 9 , 407–416 (2023).
Chinnoy, E. D. et al. Performance of seven consumer sleep-tracking devices compared with polysomnography. Sleep 44 , zsaa291 (2021).
Chinoy, E. D., Cuellar, J. A., Jameson, J. T. & Markwald, R. R. Performance of four commercial wearable sleep-tracking devices tested under unrestricted conditions at home in healthy young adults. Nat. Sci. Sleep 14 , 493–516 (2022).
Haghayegh, S., Khoshnevis, S., Smolensky, M. H., Diller, K. R. & Castriotta, R. J. Performance assessment of new-generation Fitbit technology in deriving sleep parameters and stages. Chronobiol. Int. 37 , 47–59 (2020).
Burkart, S. et al. Comparison of multichannel and single-channel wrist-based devices with polysomnography to measure sleep in children and adolescents. J. Clin. Sleep Med. 17 , 645–652 (2021).
Master, H. et al. Association of step counts over time with the risk of chronic disease in the All of Us Research Program. Nat. Med. 28 , 2301–2308 (2022).
Perry, A. S. et al. Association of longitudinal activity measures and diabetes risk: an analysis from the National Institutes of Health All of Us Research Program. J. Clin. Endocrinol. Metab. 108 , 1101–1109 (2023).
Stamatakis, E. et al. Association of wearable device-measured vigorous intermittent lifestyle physical activity with mortality. Nat. Med. 28 , 2521–2529 (2022).
Fang, Y., Forger, D. B., Frank, E., Sen, S. & Goldstein, C. Day-to-day variability in sleep parameters and depression risk: a prospective cohort study of training physicians. npj Digit. Med. 4 , 28 (2021).
All of Us Research Program Investigators et al. The ‘All of Us’ Research Program. N. Engl. J. Med. 381 , 668–676 (2019).
Mayo, K. R. et al. The All of Us data and research center: creating a secure, scalable, and sustainable ecosystem for biomedical research. Annu. Rev. Biomed. Data Sci. 6 , 443–464 (2023).
Mc Carthy, C. E. et al. Sleep patterns and the risk of acute stroke: results from the INTERSTROKE international case–control study. Neurology 100 , e2191–e2203 (2023).
Al Lawati, N. M., Patel, S. R. & Ayas, N. T. Epidemiology, risk factors, and consequences of obstructive sleep apnea and short sleep duration. Prog. Cardiovasc. Dis. 51 , 285–293 (2009).
Linz, D. et al. Associations of obstructive sleep apnea with atrial fibrillation and continuous positive airway pressure treatment: a review. JAMA Cardiol. 3 , 532–540 (2018).
Scott, H. et al. Sleep irregularity is associated with hypertension: findings from over 2 million nights with a large global population sample. Hypertension 80 , 1117–1126 (2023).
Zhai, L., Zhang, H. & Zhang, D. Sleep duration and depression among adults: a meta-analysis of prospective studies. Depress. Anxiety 32 , 664–670 (2015).
Li, Y. et al. The brain structure and genetic mechanisms underlying the nonlinear association between sleep duration, cognition and mental health. Nat. Aging 2 , 425–437 (2022).
Ford, D. E. & Kamerow, D. B. Epidemiologic study of sleep disturbances and psychiatric disorders. An opportunity for prevention? JAMA 262 , 1479–1484 (1989).
Huang, T., Mariani, S. & Redline, S. Sleep irregularity and risk of cardiovascular events: the multi-ethnic study of atherosclerosis. J. Am. Coll. Cardiol. 75 , 991–999 (2020).
Irish, L. A., Kline, C. E., Gunn, H. E., Buysse, D. J. & Hall, M. H. The role of sleep hygiene in promoting public health: a review of empirical evidence. Sleep. Med. Rev. 22 , 23–36 (2015).
Zuraikat, F. M. et al. Sleep regularity and cardiometabolic heath: is variability in sleep patterns a risk factor for excess adiposity and glycemic dysregulation? Curr. Diab. Rep. 20 , 38 (2020).
Kwon, Y. et al. Association of sleep characteristics with atrial fibrillation: the Multi-Ethnic Study of Atherosclerosis. Thorax 70 , 873–879 (2015).
Christensen, M. A. et al. Sleep characteristics that predict atrial fibrillation. Heart Rhythm 15 , 1289–1295 (2018).
Chen, P. S., Chen, L. S., Fishbein, M. C., Lin, S. F. & Nattel, S. Role of the autonomic nervous system in atrial fibrillation: pathophysiology and therapy. Circ. Res. 114 , 1500–1515 (2014).
Somers, V. K., Dyken, M. E., Mark, A. L. & Abboud, F. M. Sympathetic-nerve activity during sleep in normal subjects. N. Engl. J. Med. 328 , 303–307 (1993).
Dhingra, L. S. et al. Use of wearable devices in individuals with or at risk for cardiovascular disease in the US, 2019 to 2020. JAMA Netw. Open 6 , e2316634 (2023).
Beattie, Z. et al. Estimation of sleep stages in a healthy adult population from optical plethysmography and accelerometer signals. Physiol. Meas. 38 , 1968–1979 (2017).
Lim, S. E., Kim, H. S., Lee, S. W., Bae, K. H. & Baek, Y. H. Validation of Fitbit Inspire 2(TM) against polysomnography in adults considering adaptation for use. Nat. Sci. Sleep 15 , 59–67 (2023).
Younes, M. et al. Reliability of the American Academy of Sleep Medicine rules for assessing sleep depth in clinical practice. J. Clin. Sleep Med. 14 , 205–213 (2018).
Younes, M., Raneri, J. & Hanly, P. Staging sleep in polysomnograms: analysis of inter-scorer variability. J. Clin. Sleep Med. 12 , 885–894 (2016).
Master, H. et al. How Fitbit data are being made available to registered researchers in All of Us Research Program. Pac. Symp. Biocomput. 28 , 19–30 (2023).
McCauley, P. et al. A new mathematical model for the homeostatic effects of sleep loss on neurobehavioral performance. J. Theor. Biol. 256 , 227–239 (2009).
Denny, J. C. et al. PheWAS: demonstrating the feasibility of a phenome-wide scan to discover gene–disease associations. Bioinformatics 26 , 1205–1210 (2010).
Wu, P. et al. Mapping ICD-10 and ICD-10-CM codes to phecodes: workflow development and initial evaluation. JMIR Med. Inform. 7 , e14325 (2019).
Zheng, N. S. et al. PheMap: a multi-resource knowledge base for high-throughput phenotyping within electronic health records. J. Am. Med. Inform. Assoc. 27 , 1675–1687 (2020).
Friligkou, E. et al. Integrating genome-wide information and wearable device data to explore the link of anxiety and antidepressants with heart rate variability. Preprint at medRxiv https://doi.org/10.1101/2023.08.02.23293170 (2023).
Download references
Acknowledgements
This material is based on work that is partially funded by an unrestricted gift from Google. The study was supported by National Institutes of Health (NIH) grant nos. R21 HL172038 (E.L.B., D.M.R.), R61/R33 HL158941 (E.L.B.) and R01 FD007627 (E.L.B.). We thank All of Us participants for their contributions, without whom this research would not have been possible. We also thank the NIH’s All of Us Research Program for making available the cohort examined in this study. The All of Us Research Program is supported by the NIH, Office of the Director: Regional Medical Centers (1 OT2 OD026549, 1 OT2 OD026554, 1 OT2 OD026557, 1 OT2 OD026556, 1 OT2 OD026550, 1 OT2 OD 026552, 1 OT2 OD026553, 1 OT2 OD026548, 1 OT2 OD026551, 1 OT2 OD026555; IAA: AOD21037, AOD22003, AOD16037, AOD21041); Federally Qualified Health Centers (HHSN 263201600085U); Data and Research Center (5 U2C OD023196); Biobank (1 U24 OD023121); The Participant Center (U24 OD023176); Participant Technology Systems Center (1 U24 OD023163); Communications and Engagement (3 OT2 OD023205, 3 OT2 OD023206); and Community Partners (1 OT2 OD025277, 3 OT2 OD025315, 1 OT2 OD025337, 1 OT2 OD025276).
Author information
Authors and affiliations.
Yale School of Medicine, Yale University, New Haven, CT, USA
Neil S. Zheng
Brigham and Women’s Hospital, Boston, MA, USA
Vanderbilt Institute for Clinical and Translational Research, Vanderbilt University Medical Center, Nashville, TN, USA
Jeffrey Annis, Hiral Master & Stacy Desine
Center for Digital Genomic Medicine, Department of Medicine, Vanderbilt University Medical Center, Nashville, TN, USA
Jeffrey Annis, Hiral Master, Lide Han, Peyton Coleman, Douglas M. Ruderfer & Evan L. Brittain
Division of Cardiovascular Medicine, Vanderbilt University Medical Center, Nashville, TN, USA
Jeffrey Annis & Evan L. Brittain
Division of Genetic Medicine, Department of Medicine, Vanderbilt Genetics Institute, Vanderbilt University Medical Center, Nashville, TN, USA
Lide Han & Douglas M. Ruderfer
Google, Mountain View, CA, USA
Karla Gleichauf, Jack H. Ching, John Hernandez & Logan D. Schneider
Nelson Connects, San Francisco, CA, USA
Melody Nasser
Department of Psychiatry and Behavioral Sciences, Vanderbilt University Medical Center, Nashville, TN, USA
Peyton Coleman & Douglas M. Ruderfer
Department of Biomedical Informatics, Vanderbilt University Medical Center, Nashville, TN, USA
Douglas M. Ruderfer
Sleep Medicine Center, Department of Psychiatry and Behavioral Sciences, Stanford University School of Medicine, Redwood City, CA, USA
Logan D. Schneider
You can also search for this author in PubMed Google Scholar
Contributions
N.S.Z., J.A., H.M., L.H., D.M.R. and E.L.B. conceived of and designed the study. J.A. performed statistical analyses. N.S.Z. and J.A. prepared the figures. All authors participated in data interpretation. N.S.Z., J.A. and E.L.B. drafted the paper. All authors reviewed and approved the final paper.
Corresponding author
Correspondence to Evan L. Brittain .
Ethics declarations
Competing interests.
D.M.R. has served on advisory boards for Illumina and Alkermes and has received research funds unrelated to this work from PTC Therapeutics. E.L.B. has received research funds unrelated to this work from United Therapeutics. K.G., J.H.C., J.H. and L.D.S. are employees of Google and own Alphabet stock as part of the standard compensation package. L.D.S. has been compensated for participation in speakers’ bureaus and advisory boards by Eisai Pharmaceuticals, Jazz Pharmaceuticals, Avadel Pharmaceuticals and Harmony Biosciences, unrelated to this work. J.H. serves on the board of directors for ResMed and owns ResMed stock. Fitbit and Google were not involved in the collection, management and analysis of the data, nor in the decision to submit the manuscript for publication. Team members from Fitbit and Google provided input during the design and conduct of the study, participated in interpretation of results, and participated in preparation, review and approval of the manuscript. The All of Us Research Program was not involved in the design and conduct of the study; collection, management and analysis of the data; preparation, review or approval of the manuscript; and decision to submit the manuscript for publication. To ensure privacy of participants, data used for this study were accessed and available to approved researchers only following registration, completion of ethics training and attestation of a data use agreement through the All of Us Research Workbench platform, which can be accessed via https://workbench.researchallofus.org/login . The other authors declare no competing interests.
Peer review
Peer review information.
Nature Medicine thanks Hannah Scott and the other, anonymous, reviewer(s) for their contribution to the peer review of this work. Primary Handling Editor: Michael Basson, in collaboration with the Nature Medicine team.
Additional information
Publisher’s note Springer Nature remains neutral with regard to jurisdictional claims in published maps and institutional affiliations.
Extended data
Extended data fig. 1 consort diagram showing inclusion/exclusion criteria..
The figure shows the inclusion and exclusion criteria for participants and participants-nights for the study. Boxes on the left-hand side of the figure describe the inclusion criteria and boxes on the right-hand side of the figure describe the exclusion criteria.
Extended Data Fig. 2 Population medians of Fitbit-derived sleep metrics from 2017 to 2022.
Collection for Fitbit data for sleep duration, restless sleep duration, and sleep irregularity started prior to 2017 whereas collection for Fitbit-derived sleep stages started in 2017. Point and number indicate the population median. Errors bars indicate the 25th and 75th percentile. Sample sizes were 3,617 for 2017, 4,345 for 2018, 5,319 for 2019, 5,647 for 2020, 5,585 for 2021, and 4,626 for 2022.
Extended Data Fig. 3 Forest plots from stratified analyses of Cox proportional hazard models for associations between 75th vs. 25th percentile Fitbit-derived sleep metric and chronic disease.
The figure shows significant findings from a total of 112 stratified analyses (13 strata 9 significant associations from overall Cox proportional hazard models). The points indicate the hazard ratios and the error bars indicate the 95% confidence intervals. Strata with fewer than 20 cases were excluded to comply with All of Us Data and Statistics dissemination policies. All Cox proportional hazards models were adjusted for age, sex, baseline body mass index (BMI), baseline systolic blood pressure, smoking status, alcohol drinking status, education status, time-varying average daily step count, and prior diagnoses of cancer or coronary artery disease.
Extended Data Fig. 4 Phenome-wide analyses exploring relationship between traditional sleep onset times (8:00 PM to 2:00 AM) and incident disease.
All phenome-wide analyses were performed using multiple logistic regression models adjusted for age, sex, average daily step count across the entire monitoring period, and EHR length. All reported P-values were based on two-tailed probability. Bonferroni significance line of 3.06 × 10–5 is indicated by red line and P-value of 0.05 is indicated by the blue line. Upwards triangles indicate odds ratios > 1 and downwards triangles indicated odds ratios < 1.
Supplementary information
Reporting summary, rights and permissions.
Open Access This article is licensed under a Creative Commons Attribution 4.0 International License, which permits use, sharing, adaptation, distribution and reproduction in any medium or format, as long as you give appropriate credit to the original author(s) and the source, provide a link to the Creative Commons licence, and indicate if changes were made. The images or other third party material in this article are included in the article’s Creative Commons licence, unless indicated otherwise in a credit line to the material. If material is not included in the article’s Creative Commons licence and your intended use is not permitted by statutory regulation or exceeds the permitted use, you will need to obtain permission directly from the copyright holder. To view a copy of this licence, visit http://creativecommons.org/licenses/by/4.0/ .
Reprints and permissions
About this article
Cite this article.
Zheng, N.S., Annis, J., Master, H. et al. Sleep patterns and risk of chronic disease as measured by long-term monitoring with commercial wearable devices in the All of Us Research Program. Nat Med 30 , 2648–2656 (2024). https://doi.org/10.1038/s41591-024-03155-8
Download citation
Received : 27 December 2023
Accepted : 25 June 2024
Published : 19 July 2024
Issue Date : September 2024
DOI : https://doi.org/10.1038/s41591-024-03155-8
Share this article
Anyone you share the following link with will be able to read this content:
Sorry, a shareable link is not currently available for this article.
Provided by the Springer Nature SharedIt content-sharing initiative
This article is cited by
Influence of sleep on physiological systems in atherosclerosis.
- Máté G. Kiss
- Filip K. Swirski
Nature Cardiovascular Research (2024)
Quick links
- Explore articles by subject
- Guide to authors
- Editorial policies
Sign up for the Nature Briefing newsletter — what matters in science, free to your inbox daily.


IMAGES
VIDEO