Have a language expert improve your writing
Run a free plagiarism check in 10 minutes, generate accurate citations for free.
- Knowledge Base
Methodology
- How to Write a Literature Review | Guide, Examples, & Templates

How to Write a Literature Review | Guide, Examples, & Templates
Published on January 2, 2023 by Shona McCombes . Revised on September 11, 2023.
What is a literature review? A literature review is a survey of scholarly sources on a specific topic. It provides an overview of current knowledge, allowing you to identify relevant theories, methods, and gaps in the existing research that you can later apply to your paper, thesis, or dissertation topic .
There are five key steps to writing a literature review:
- Search for relevant literature
- Evaluate sources
- Identify themes, debates, and gaps
- Outline the structure
- Write your literature review
A good literature review doesn’t just summarize sources—it analyzes, synthesizes , and critically evaluates to give a clear picture of the state of knowledge on the subject.
Instantly correct all language mistakes in your text
Upload your document to correct all your mistakes in minutes
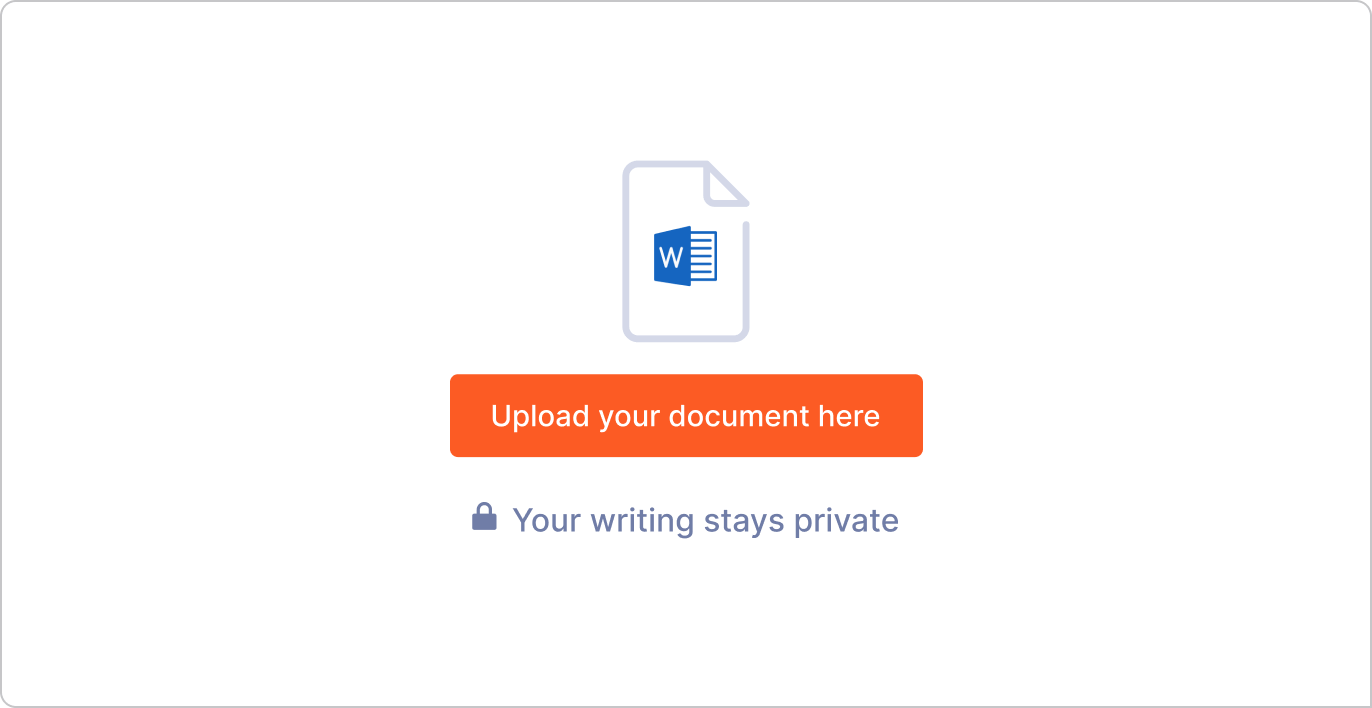
Table of contents
What is the purpose of a literature review, examples of literature reviews, step 1 – search for relevant literature, step 2 – evaluate and select sources, step 3 – identify themes, debates, and gaps, step 4 – outline your literature review’s structure, step 5 – write your literature review, free lecture slides, other interesting articles, frequently asked questions, introduction.
- Quick Run-through
- Step 1 & 2
When you write a thesis , dissertation , or research paper , you will likely have to conduct a literature review to situate your research within existing knowledge. The literature review gives you a chance to:
- Demonstrate your familiarity with the topic and its scholarly context
- Develop a theoretical framework and methodology for your research
- Position your work in relation to other researchers and theorists
- Show how your research addresses a gap or contributes to a debate
- Evaluate the current state of research and demonstrate your knowledge of the scholarly debates around your topic.
Writing literature reviews is a particularly important skill if you want to apply for graduate school or pursue a career in research. We’ve written a step-by-step guide that you can follow below.
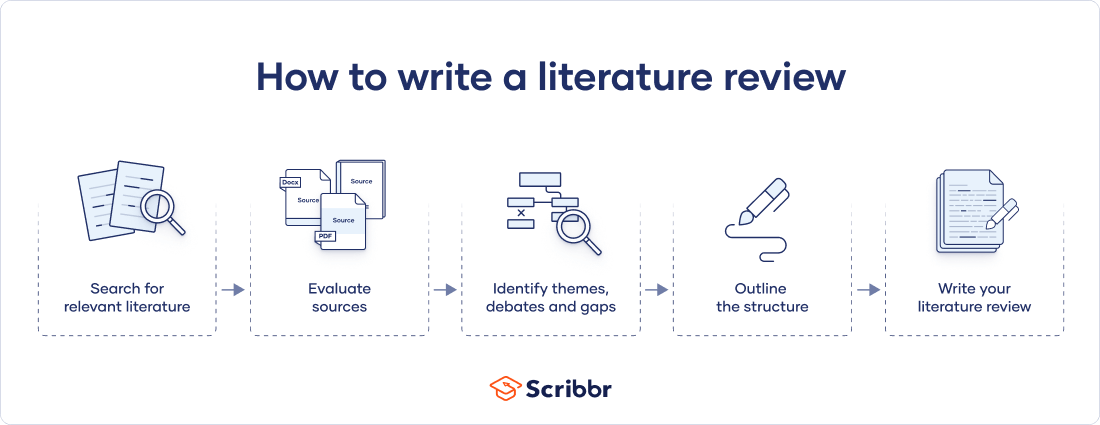
Don't submit your assignments before you do this
The academic proofreading tool has been trained on 1000s of academic texts. Making it the most accurate and reliable proofreading tool for students. Free citation check included.
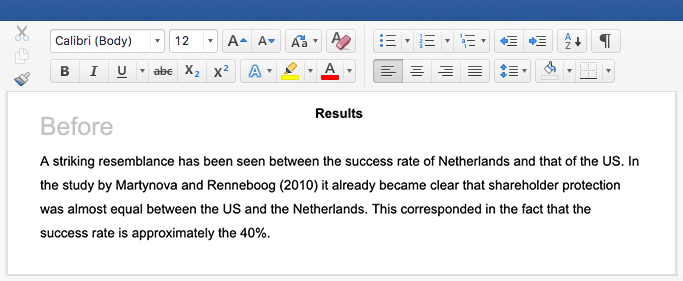
Try for free
Writing literature reviews can be quite challenging! A good starting point could be to look at some examples, depending on what kind of literature review you’d like to write.
- Example literature review #1: “Why Do People Migrate? A Review of the Theoretical Literature” ( Theoretical literature review about the development of economic migration theory from the 1950s to today.)
- Example literature review #2: “Literature review as a research methodology: An overview and guidelines” ( Methodological literature review about interdisciplinary knowledge acquisition and production.)
- Example literature review #3: “The Use of Technology in English Language Learning: A Literature Review” ( Thematic literature review about the effects of technology on language acquisition.)
- Example literature review #4: “Learners’ Listening Comprehension Difficulties in English Language Learning: A Literature Review” ( Chronological literature review about how the concept of listening skills has changed over time.)
You can also check out our templates with literature review examples and sample outlines at the links below.
Download Word doc Download Google doc
Before you begin searching for literature, you need a clearly defined topic .
If you are writing the literature review section of a dissertation or research paper, you will search for literature related to your research problem and questions .
Make a list of keywords
Start by creating a list of keywords related to your research question. Include each of the key concepts or variables you’re interested in, and list any synonyms and related terms. You can add to this list as you discover new keywords in the process of your literature search.
- Social media, Facebook, Instagram, Twitter, Snapchat, TikTok
- Body image, self-perception, self-esteem, mental health
- Generation Z, teenagers, adolescents, youth
Search for relevant sources
Use your keywords to begin searching for sources. Some useful databases to search for journals and articles include:
- Your university’s library catalogue
- Google Scholar
- Project Muse (humanities and social sciences)
- Medline (life sciences and biomedicine)
- EconLit (economics)
- Inspec (physics, engineering and computer science)
You can also use boolean operators to help narrow down your search.
Make sure to read the abstract to find out whether an article is relevant to your question. When you find a useful book or article, you can check the bibliography to find other relevant sources.
You likely won’t be able to read absolutely everything that has been written on your topic, so it will be necessary to evaluate which sources are most relevant to your research question.
For each publication, ask yourself:
- What question or problem is the author addressing?
- What are the key concepts and how are they defined?
- What are the key theories, models, and methods?
- Does the research use established frameworks or take an innovative approach?
- What are the results and conclusions of the study?
- How does the publication relate to other literature in the field? Does it confirm, add to, or challenge established knowledge?
- What are the strengths and weaknesses of the research?
Make sure the sources you use are credible , and make sure you read any landmark studies and major theories in your field of research.
You can use our template to summarize and evaluate sources you’re thinking about using. Click on either button below to download.
Take notes and cite your sources
As you read, you should also begin the writing process. Take notes that you can later incorporate into the text of your literature review.
It is important to keep track of your sources with citations to avoid plagiarism . It can be helpful to make an annotated bibliography , where you compile full citation information and write a paragraph of summary and analysis for each source. This helps you remember what you read and saves time later in the process.
Receive feedback on language, structure, and formatting
Professional editors proofread and edit your paper by focusing on:
- Academic style
- Vague sentences
- Style consistency
See an example
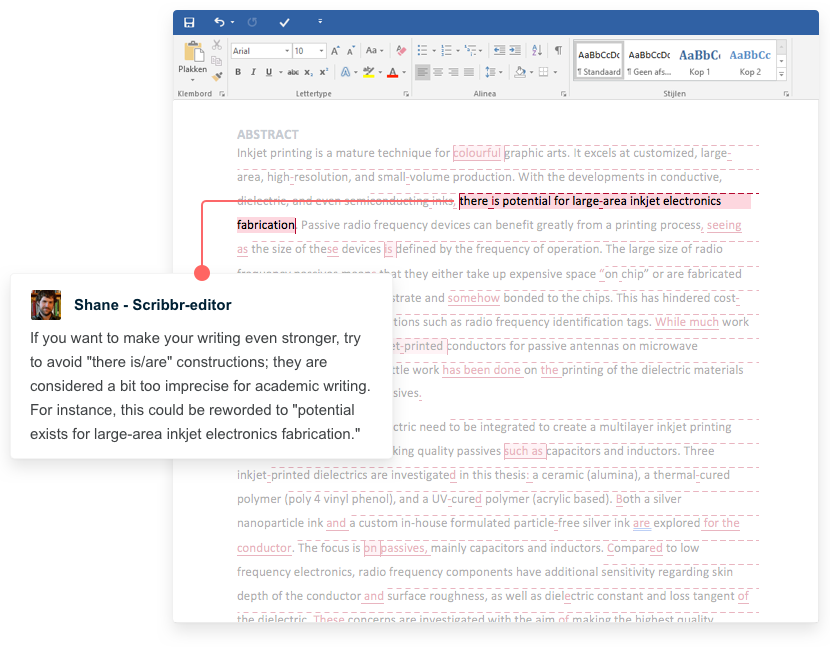
To begin organizing your literature review’s argument and structure, be sure you understand the connections and relationships between the sources you’ve read. Based on your reading and notes, you can look for:
- Trends and patterns (in theory, method or results): do certain approaches become more or less popular over time?
- Themes: what questions or concepts recur across the literature?
- Debates, conflicts and contradictions: where do sources disagree?
- Pivotal publications: are there any influential theories or studies that changed the direction of the field?
- Gaps: what is missing from the literature? Are there weaknesses that need to be addressed?
This step will help you work out the structure of your literature review and (if applicable) show how your own research will contribute to existing knowledge.
- Most research has focused on young women.
- There is an increasing interest in the visual aspects of social media.
- But there is still a lack of robust research on highly visual platforms like Instagram and Snapchat—this is a gap that you could address in your own research.
There are various approaches to organizing the body of a literature review. Depending on the length of your literature review, you can combine several of these strategies (for example, your overall structure might be thematic, but each theme is discussed chronologically).
Chronological
The simplest approach is to trace the development of the topic over time. However, if you choose this strategy, be careful to avoid simply listing and summarizing sources in order.
Try to analyze patterns, turning points and key debates that have shaped the direction of the field. Give your interpretation of how and why certain developments occurred.
If you have found some recurring central themes, you can organize your literature review into subsections that address different aspects of the topic.
For example, if you are reviewing literature about inequalities in migrant health outcomes, key themes might include healthcare policy, language barriers, cultural attitudes, legal status, and economic access.
Methodological
If you draw your sources from different disciplines or fields that use a variety of research methods , you might want to compare the results and conclusions that emerge from different approaches. For example:
- Look at what results have emerged in qualitative versus quantitative research
- Discuss how the topic has been approached by empirical versus theoretical scholarship
- Divide the literature into sociological, historical, and cultural sources
Theoretical
A literature review is often the foundation for a theoretical framework . You can use it to discuss various theories, models, and definitions of key concepts.
You might argue for the relevance of a specific theoretical approach, or combine various theoretical concepts to create a framework for your research.
Like any other academic text , your literature review should have an introduction , a main body, and a conclusion . What you include in each depends on the objective of your literature review.
The introduction should clearly establish the focus and purpose of the literature review.
Depending on the length of your literature review, you might want to divide the body into subsections. You can use a subheading for each theme, time period, or methodological approach.
As you write, you can follow these tips:
- Summarize and synthesize: give an overview of the main points of each source and combine them into a coherent whole
- Analyze and interpret: don’t just paraphrase other researchers — add your own interpretations where possible, discussing the significance of findings in relation to the literature as a whole
- Critically evaluate: mention the strengths and weaknesses of your sources
- Write in well-structured paragraphs: use transition words and topic sentences to draw connections, comparisons and contrasts
In the conclusion, you should summarize the key findings you have taken from the literature and emphasize their significance.
When you’ve finished writing and revising your literature review, don’t forget to proofread thoroughly before submitting. Not a language expert? Check out Scribbr’s professional proofreading services !
This article has been adapted into lecture slides that you can use to teach your students about writing a literature review.
Scribbr slides are free to use, customize, and distribute for educational purposes.
Open Google Slides Download PowerPoint
If you want to know more about the research process , methodology , research bias , or statistics , make sure to check out some of our other articles with explanations and examples.
- Sampling methods
- Simple random sampling
- Stratified sampling
- Cluster sampling
- Likert scales
- Reproducibility
Statistics
- Null hypothesis
- Statistical power
- Probability distribution
- Effect size
- Poisson distribution
Research bias
- Optimism bias
- Cognitive bias
- Implicit bias
- Hawthorne effect
- Anchoring bias
- Explicit bias
A literature review is a survey of scholarly sources (such as books, journal articles, and theses) related to a specific topic or research question .
It is often written as part of a thesis, dissertation , or research paper , in order to situate your work in relation to existing knowledge.
There are several reasons to conduct a literature review at the beginning of a research project:
- To familiarize yourself with the current state of knowledge on your topic
- To ensure that you’re not just repeating what others have already done
- To identify gaps in knowledge and unresolved problems that your research can address
- To develop your theoretical framework and methodology
- To provide an overview of the key findings and debates on the topic
Writing the literature review shows your reader how your work relates to existing research and what new insights it will contribute.
The literature review usually comes near the beginning of your thesis or dissertation . After the introduction , it grounds your research in a scholarly field and leads directly to your theoretical framework or methodology .
A literature review is a survey of credible sources on a topic, often used in dissertations , theses, and research papers . Literature reviews give an overview of knowledge on a subject, helping you identify relevant theories and methods, as well as gaps in existing research. Literature reviews are set up similarly to other academic texts , with an introduction , a main body, and a conclusion .
An annotated bibliography is a list of source references that has a short description (called an annotation ) for each of the sources. It is often assigned as part of the research process for a paper .
Cite this Scribbr article
If you want to cite this source, you can copy and paste the citation or click the “Cite this Scribbr article” button to automatically add the citation to our free Citation Generator.
McCombes, S. (2023, September 11). How to Write a Literature Review | Guide, Examples, & Templates. Scribbr. Retrieved August 29, 2024, from https://www.scribbr.com/dissertation/literature-review/
Is this article helpful?
Shona McCombes
Other students also liked, what is a theoretical framework | guide to organizing, what is a research methodology | steps & tips, how to write a research proposal | examples & templates, "i thought ai proofreading was useless but..".
I've been using Scribbr for years now and I know it's a service that won't disappoint. It does a good job spotting mistakes”
- UConn Library
- Literature Review: The What, Why and How-to Guide
- Introduction
Literature Review: The What, Why and How-to Guide — Introduction
- Getting Started
- How to Pick a Topic
- Strategies to Find Sources
- Evaluating Sources & Lit. Reviews
- Tips for Writing Literature Reviews
- Writing Literature Review: Useful Sites
- Citation Resources
- Other Academic Writings
What are Literature Reviews?
So, what is a literature review? "A literature review is an account of what has been published on a topic by accredited scholars and researchers. In writing the literature review, your purpose is to convey to your reader what knowledge and ideas have been established on a topic, and what their strengths and weaknesses are. As a piece of writing, the literature review must be defined by a guiding concept (e.g., your research objective, the problem or issue you are discussing, or your argumentative thesis). It is not just a descriptive list of the material available, or a set of summaries." Taylor, D. The literature review: A few tips on conducting it . University of Toronto Health Sciences Writing Centre.
Goals of Literature Reviews
What are the goals of creating a Literature Review? A literature could be written to accomplish different aims:
- To develop a theory or evaluate an existing theory
- To summarize the historical or existing state of a research topic
- Identify a problem in a field of research
Baumeister, R. F., & Leary, M. R. (1997). Writing narrative literature reviews . Review of General Psychology , 1 (3), 311-320.
What kinds of sources require a Literature Review?
- A research paper assigned in a course
- A thesis or dissertation
- A grant proposal
- An article intended for publication in a journal
All these instances require you to collect what has been written about your research topic so that you can demonstrate how your own research sheds new light on the topic.
Types of Literature Reviews
What kinds of literature reviews are written?
Narrative review: The purpose of this type of review is to describe the current state of the research on a specific topic/research and to offer a critical analysis of the literature reviewed. Studies are grouped by research/theoretical categories, and themes and trends, strengths and weakness, and gaps are identified. The review ends with a conclusion section which summarizes the findings regarding the state of the research of the specific study, the gaps identify and if applicable, explains how the author's research will address gaps identify in the review and expand the knowledge on the topic reviewed.
- Example : Predictors and Outcomes of U.S. Quality Maternity Leave: A Review and Conceptual Framework: 10.1177/08948453211037398
Systematic review : "The authors of a systematic review use a specific procedure to search the research literature, select the studies to include in their review, and critically evaluate the studies they find." (p. 139). Nelson, L. K. (2013). Research in Communication Sciences and Disorders . Plural Publishing.
- Example : The effect of leave policies on increasing fertility: a systematic review: 10.1057/s41599-022-01270-w
Meta-analysis : "Meta-analysis is a method of reviewing research findings in a quantitative fashion by transforming the data from individual studies into what is called an effect size and then pooling and analyzing this information. The basic goal in meta-analysis is to explain why different outcomes have occurred in different studies." (p. 197). Roberts, M. C., & Ilardi, S. S. (2003). Handbook of Research Methods in Clinical Psychology . Blackwell Publishing.
- Example : Employment Instability and Fertility in Europe: A Meta-Analysis: 10.1215/00703370-9164737
Meta-synthesis : "Qualitative meta-synthesis is a type of qualitative study that uses as data the findings from other qualitative studies linked by the same or related topic." (p.312). Zimmer, L. (2006). Qualitative meta-synthesis: A question of dialoguing with texts . Journal of Advanced Nursing , 53 (3), 311-318.
- Example : Women’s perspectives on career successes and barriers: A qualitative meta-synthesis: 10.1177/05390184221113735
Literature Reviews in the Health Sciences
- UConn Health subject guide on systematic reviews Explanation of the different review types used in health sciences literature as well as tools to help you find the right review type
- << Previous: Getting Started
- Next: How to Pick a Topic >>
- Last Updated: Sep 21, 2022 2:16 PM
- URL: https://guides.lib.uconn.edu/literaturereview

- Skip to main content
- Skip to primary sidebar
- Request Info
- Search Search Site Faculty/Staff
- Open Navigation Menu Menu Close Navigation Menu
- Literature Review Guidelines
Making sense of what has been written on your topic.
Goals of a literature review:.
Before doing work in primary sources, historians must know what has been written on their topic. They must be familiar with theories and arguments–as well as facts–that appear in secondary sources.
Before you proceed with your research project, you too must be familiar with the literature: you do not want to waste time on theories that others have disproved and you want to take full advantage of what others have argued. You want to be able to discuss and analyze your topic.
Your literature review will demonstrate your familiarity with your topic’s secondary literature.
GUIDELINES FOR A LITERATURE REVIEW:
1) LENGTH: 8-10 pages of text for Senior Theses (485) (consult with your professor for other classes), with either footnotes or endnotes and with a works-consulted bibliography. [See also the citation guide on this site.]
2) NUMBER OF WORKS REVIEWED: Depends on the assignment, but for Senior Theses (485), at least ten is typical.
3) CHOOSING WORKS:
Your literature review must include enough works to provide evidence of both the breadth and the depth of the research on your topic or, at least, one important angle of it. The number of works necessary to do this will depend on your topic. For most topics, AT LEAST TEN works (mostly books but also significant scholarly articles) are necessary, although you will not necessarily give all of them equal treatment in your paper (e.g., some might appear in notes rather than the essay). 4) ORGANIZING/ARRANGING THE LITERATURE:
As you uncover the literature (i.e., secondary writing) on your topic, you should determine how the various pieces relate to each other. Your ability to do so will demonstrate your understanding of the evolution of literature.
You might determine that the literature makes sense when divided by time period, by methodology, by sources, by discipline, by thematic focus, by race, ethnicity, and/or gender of author, or by political ideology. This list is not exhaustive. You might also decide to subdivide categories based on other criteria. There is no “rule” on divisions—historians wrote the literature without consulting each other and without regard to the goal of fitting into a neat, obvious organization useful to students.
The key step is to FIGURE OUT the most logical, clarifying angle. Do not arbitrarily choose a categorization; use the one that the literature seems to fall into. How do you do that? For every source, you should note its thesis, date, author background, methodology, and sources. Does a pattern appear when you consider such information from each of your sources? If so, you have a possible thesis about the literature. If not, you might still have a thesis.
Consider: Are there missing elements in the literature? For example, no works published during a particular (usually fairly lengthy) time period? Or do studies appear after long neglect of a topic? Do interpretations change at some point? Does the major methodology being used change? Do interpretations vary based on sources used?
Follow these links for more help on analyzing historiography and historical perspective .
5) CONTENTS OF LITERATURE REVIEW:
The literature review is a research paper with three ingredients:
a) A brief discussion of the issue (the person, event, idea). [While this section should be brief, it needs to set up the thesis and literature that follow.] b) Your thesis about the literature c) A clear argument, using the works on topic as evidence, i.e., you discuss the sources in relation to your thesis, not as a separate topic.
These ingredients must be presented in an essay with an introduction, body, and conclusion.
6) ARGUING YOUR THESIS:
The thesis of a literature review should not only describe how the literature has evolved, but also provide a clear evaluation of that literature. You should assess the literature in terms of the quality of either individual works or categories of works. For instance, you might argue that a certain approach (e.g. social history, cultural history, or another) is better because it deals with a more complex view of the issue or because they use a wider array of source materials more effectively. You should also ensure that you integrate that evaluation throughout your argument. Doing so might include negative assessments of some works in order to reinforce your argument regarding the positive qualities of other works and approaches to the topic.
Within each group, you should provide essential information about each work: the author’s thesis, the work’s title and date, the author’s supporting arguments and major evidence.
In most cases, arranging the sources chronologically by publication date within each section makes the most sense because earlier works influenced later ones in one way or another. Reference to publication date also indicates that you are aware of this significant historiographical element.
As you discuss each work, DO NOT FORGET WHY YOU ARE DISCUSSING IT. YOU ARE PRESENTING AND SUPPORTING A THESIS ABOUT THE LITERATURE.
When discussing a particular work for the first time, you should refer to it by the author’s full name, the work’s title, and year of publication (either in parentheses after the title or worked into the sentence).
For example, “The field of slavery studies has recently been transformed by Ben Johnson’s The New Slave (2001)” and “Joe Doe argues in his 1997 study, Slavery in America, that . . . .”
Your paper should always note secondary sources’ relationship to each other, particularly in terms of your thesis about the literature (e.g., “Unlike Smith’s work, Mary Brown’s analysis reaches the conclusion that . . . .” and “Because of Anderson’s reliance on the president’s personal papers, his interpretation differs from Barry’s”). The various pieces of the literature are “related” to each other, so you need to indicate to the reader some of that relationship. (It helps the reader follow your thesis, and it convinces the reader that you know what you are talking about.)
7) DOCUMENTATION:
Each source you discuss in your paper must be documented using footnotes/endnotes and a bibliography. Providing author and title and date in the paper is not sufficient. Use correct Turabian/Chicago Manual of Style form. [See Bibliography and Footnotes/Endnotes pages.]
In addition, further supporting, but less significant, sources should be included in content foot or endnotes . (e.g., “For a similar argument to Ben Johnson’s, see John Terry, The Slave Who Was New (New York: W. W. Norton, 1985), 3-45.”)
8 ) CONCLUSION OF LITERATURE REVIEW:
Your conclusion should not only reiterate your argument (thesis), but also discuss questions that remain unanswered by the literature. What has the literature accomplished? What has not been studied? What debates need to be settled?
Additional writing guidelines
History and American Studies
- About the Department
- Major Requirements & Courses
- What courses will I take as an History major?
- What can I do with my History degree?
- History 485
- Methodology
- Choosing a Topic
- Book Reviews
- Historiographic Clues
- Understanding Historical Perspective
- Sample Literature Review
- Using Quotations
- Ellipses and Brackets
- Footnotes and Endnotes
- Content Notes
- Citation Guide
- Citing Non-Print Resources
- How to Annotate
- Annotated Examples
- Journals vs. Magazines
- Understanding Plagiarism
- Historians Define Plagiarism
- Plagiarism Tutorial
- UMW Honor System
- Presentation Guidelines
- Tips for Leading Seminars
- Hints for Class Discussion
- Speaking Center
- Guidelines for a Research Paper
- Library Research Plan
- How to Use ILL
- Database Guide
- Guide to Online Research
- Writing Guidelines
- Recognizing Passive Voice
- Introduction and Conclusion
- MS Word’s Grammar and Spellcheck
- Writing Center
- What You Need to Know
- Links to Online Primary Sources by Region
- What will I learn from my American Studies major?
- What courses will I take as an American Studies major?
- What can I do with my American Studies degree?
- American Studies 485
- For Prospective Students
- Honors and Award Recipients
- Internships
Alumni Intros
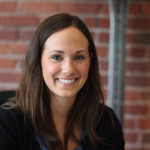
How have History & American Studies majors built careers after earning their degrees? Learn more by clicking the image above.
Recent Posts
- History and American Studies Symposium–April 26, 2024
- Fall 2024 Courses
- Fall 2023 Symposium – 12/8 – All Welcome!
- Spring ’24 Course Flyers
- Internship Opportunity – Chesapeake Gateways Ambassador
- Congratulations to our Graduates!
- History and American Studies Symposium–April 21, 2023
- View umwhistory’s profile on Facebook
- View umwhistory’s profile on Twitter
Academia.edu no longer supports Internet Explorer.
To browse Academia.edu and the wider internet faster and more securely, please take a few seconds to upgrade your browser .
Enter the email address you signed up with and we'll email you a reset link.
- We're Hiring!
- Help Center
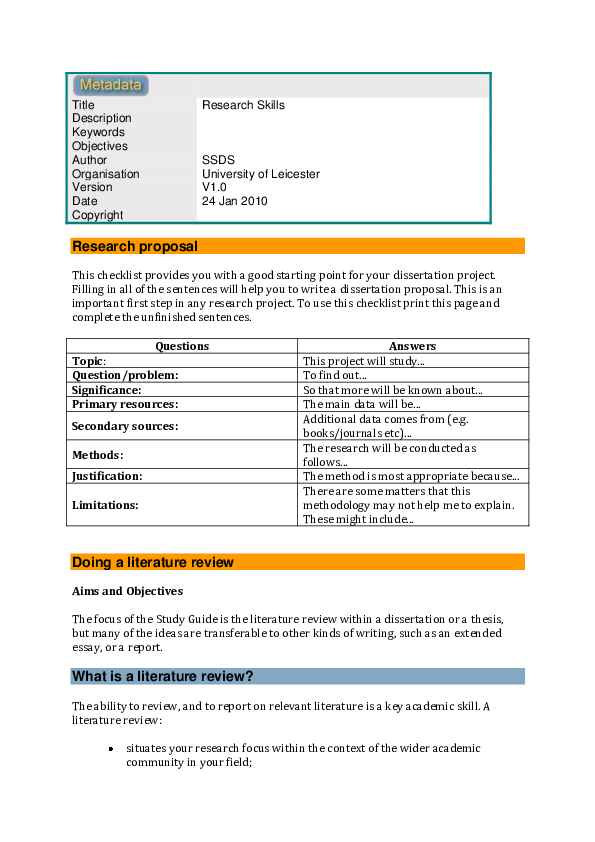
Doing a literature review Aims and Objectives What is a literature review

Related Papers
HUMANUS DISCOURSE
Humanus Discourse
The importance of literature review in academic writing of different categories, levels, and purposes cannot be overemphasized. The literature review establishes both the relevance and justifies why new research is relevant. It is through a literature review that a gap would be established, and which the new research would fix. Once the literature review sits properly in the research work, the objectives/research questions naturally fall into their proper perspective. Invariably, other chapters of the research work would be impacted as well. In most instances, scanning through literature also provides you with the need and justification for your research and may also well leave a hint for further research. Literature review in most instances exposes a researcher to the right methodology to use. The literature review is the nucleus of a research work that might when gotten right spotlights a work and can as well derail a research work when done wrongly. This paper seeks to unveil the practical guides to writing a literature review, from purpose, and components to tips. It follows through the exposition of secondary literature. It exposes the challenges in writing a literature review and at the same time recommended tips that when followed will impact the writing of the literature review.
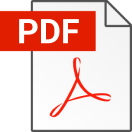
Ignacio Illan Conde
Andrew Johnson
This chapter describes the process of writing a literature review and what the product should look like
Rebekka Tunombili
Cut Oktaviani
Tatam Chiway , Abdullah Ramdhani , Muhammad Ali Ramdhani
How to Practice Academic Medicine and Publish from Developing Countries?
In scientific writing, whether it is a research paper, thesis, or dissertation, it is important to investigate a problem that has not been tackled before—that is, to fill a gap in the current knowledge. The first question an editor or reviewer asks after seeing a submission is ‘Why did the authors do the work, is the subject original?’
Alfi Rahman
This Study Guide explains why literature reviews are needed, and how they can be conducted and reported. Related Study Guides are: Referencing and bibliographies, Avoiding plagiarism, Writing a dissertation, What is critical reading? What is critical writing? The focus of the Study Guide is the literature review within a dissertation or a thesis, but many of the ideas are transferable to other kinds of writing, such as an extended essay, or a report. After reading your literature review, it should be clear to the reader that you have up-to-date awareness of the relevant work of others, and that the research question you are asking is relevant. However, don't promise too much! Be wary of saying that your research will solve a problem, or that it will change practice. It would be safer and probably more realistic to say that your research will 'address a gap', rather than that it will 'fill a gap'.
A literature review is a critical consideration of the work by authors and researchers who have written on a particular topic. IT involves synthesising these writings so that a 'picture' of the issue under review forms. Therefore, it requires you to use summarising, analytical and evaluative skills. The effectiveness of these will, to a large extent, depend on your ability to link the work of various authors highlighting similarities, differences, strengths and weaknesses. A Literature Review is not a list describing or summarising one piece of literature after another, so avoid beginning each paragraph with the name of the researcher.
yakubu nawati
Loading Preview
Sorry, preview is currently unavailable. You can download the paper by clicking the button above.
RELATED PAPERS
tecnico emergencias
Gavin Mount
Auxiliadora Padilha
Christopher N Lawrence
Amanda Bolderston
KABASO OLIVER
Dr. oliver kabaso
Mohammed I S
Nirmal Kumar Raut
Frances Slack
Gazala Hasan
Scientific Research Publishing: Creative Education
Dr. Qais Faryadi
KANNANAYAKAL RAJAN
InSITE Conference
shardul pandya
Oluwatobi Balogun
Diane Keeble-Ramsay
Mike Lambert
Evaluation & Research in Education
Colum Cronin
Journal of Practical Studies in Education
Research Methodology Assignment
Meriel Louise Anne Villamil
Journal of Asian Development
Erni Murniarti
- We're Hiring!
- Help Center
- Find new research papers in:
- Health Sciences
- Earth Sciences
- Cognitive Science
- Mathematics
- Computer Science
- Academia ©2024
This paper is in the following e-collection/theme issue:
Published on 30.8.2024 in Vol 11 (2024)
Evaluation of Digital Mental Health Technologies in the United States: Systematic Literature Review and Framework Synthesis
Authors of this article:

- Julianna Catania 1 , MPH ;
- Steph Beaver 1 , MChem ;
- Rakshitha S Kamath 1 , MS, MSL ;
- Emma Worthington 2 , MPH ;
- Minyi Lu 3 , PhD ;
- Hema Gandhi 3 , PhD ;
- Heidi C Waters 3 , PhD ;
- Daniel C Malone 4 , PhD
1 Costello Medical, Boston, MA, United States
2 Costello Medical, Cambridge, United Kingdom
3 Otsuka Pharmaceutical Development & Commercialization Inc, Princeton, NJ, United States
4 Department of Pharmacotherapy, Skaggs College of Pharmacy, University of Utah, Salt Lake City, UT, United States
Corresponding Author:
Daniel C Malone, PhD
Department of Pharmacotherapy
Skaggs College of Pharmacy
University of Utah
30 S 2000 East
Salt Lake City, UT, 84112
United States
Phone: 1 801 581 6257
Email: [email protected]
Background: Digital mental health technologies (DMHTs) have the potential to enhance mental health care delivery. However, there is little information on how DMHTs are evaluated and what factors influence their use.
Objective: A systematic literature review was conducted to understand how DMHTs are valued in the United States from user, payer, and employer perspectives.
Methods: Articles published after 2017 were identified from MEDLINE, Embase, PsycINFO, Cochrane Library, the Health Technology Assessment Database, and digital and mental health congresses. Each article was evaluated by 2 independent reviewers to identify US studies reporting on factors considered in the evaluation of DMHTs targeting mental health, Alzheimer disease, epilepsy, autism spectrum disorder, or attention-deficit/hyperactivity disorder. Study quality was assessed using the Critical Appraisal Skills Program Qualitative and Cohort Studies Checklists. Studies were coded and indexed using the American Psychiatric Association’s Mental Health App Evaluation Framework to extract and synthesize relevant information, and novel themes were added iteratively as identified.
Results: Of the 4353 articles screened, data from 26 unique studies from patient, caregiver, and health care provider perspectives were included. Engagement style was the most reported theme (23/26, 88%), with users valuing DMHT usability, particularly alignment with therapeutic goals through features including anxiety management tools. Key barriers to DMHT use included limited internet access, poor technical literacy, and privacy concerns. Novel findings included the discreetness of DMHTs to avoid stigma.
Conclusions: Usability, cost, accessibility, technical considerations, and alignment with therapeutic goals are important to users, although DMHT valuation varies across individuals. DMHT apps should be developed and selected with specific user needs in mind.
Introduction
Digital health comprises a broad range of technologies, including mobile health, health information technology, wearable devices, and personalized medicine, which serve as tools to enhance health care delivery. Recently, several digital mental health (MH) therapeutics, a category of digital MH technologies (DMHTs), have received US Food and Drug Administration (FDA) approval to prevent, manage, or treat a medical disorder or disease based on evidence from superiority trials and compliance with technical guidelines [ 1 , 2 ]. However, most DMHTs, particularly apps, fall outside FDA jurisdiction because they are not intended to diagnose, treat, or prevent disease and because they are “low risk” in that they would not cause harm in the event of malfunction [ 3 ]. Due to this lack of regulatory framework, few DMHTs are supported by published efficacy studies. One study found that only 16% of MH apps recommended by college counseling centers were supported by efficacy studies published in peer-reviewed journals [ 4 ].
Nonetheless, many health care providers (HCPs) use MH apps in clinical practice. Up to 83% of behavioral health providers in a small study covering the Greater Boston area reported using apps as part of their clinical care, particularly mindfulness apps for patient anxiety management [ 5 ]. As many DMHTs are currently widely used in clinical practice without undergoing any formal assessment for quality or relevance, understanding how DMHTs should be assessed based on factors impacting their value from the perspective of key stakeholders, such as patients, caregivers, providers, payers, and employers, could improve the selection of DMHTs for use by patients, thereby increasing care quality and outcomes for those seeking MH support.
To address identified gaps, a systematic literature review (SLR) was conducted using a published framework to synthesize emerging themes from mixed methods evidence in order to understand how digital health solutions, encompassing both digital therapeutics and direct-to-consumer digital health technologies, are valued, with a focus on MH disorders, Alzheimer disease, epilepsy, autism spectrum disorder (ASD), and attention-deficit/hyperactivity disorder (ADHD) in the United States.
The SLR was performed in accordance with a prespecified protocol and reported in line with the Enhancing Transparency in Reporting the Synthesis of Qualitative Research and PRISMA (Preferred Reporting Items for Systematic Reviews and Meta-Analyses) guidelines [ 6 , 7 ]. The protocol was not registered.
Search Strategy
Electronic databases, encompassing MEDLINE (including MEDLINE In-Process, MEDLINE Daily, and MEDLINE Epub Ahead of Print); Embase; the Cochrane Library (including Cochrane Database of Systematic Reviews and Cochrane Central Register of Controlled Trials); PsycINFO; and the Health Technology Assessment Database, were selected in alignment with this SLR’s target indications and were searched on June 17, 2022. The search terms included combinations of free-text and Medical Subject Heading or Emtree terms related to indications of interest, DMHTs, and relevant outcomes or assessment types (eg, technology assessments and cost; Tables S1-S5 in Multimedia Appendix 1 ). Searches were limited to studies performed in the United States and to those published from 2017 onward.
Manual hand searches of gray literature, namely, the bibliographies of relevant SLRs identified from the electronic database searches and key conference proceedings (2019-2022), were performed to identify additional studies of relevance (Table S6 in Multimedia Appendix 1 ). The FDA website was also searched to identify factors involved in the FDA’s appraisal of relevant MH apps, which could supplement the factors identified in this SLR (Table S7 in Multimedia Appendix 1 ).
Study Selection
Studies were included in the SLR if they met prespecified criteria defined using the SPIDER (Sample, Phenomenon of Interest, Design, Evaluation, Research type) framework, which is appropriate for mixed methods research questions. Eligible studies were published in the English language, were set in the United States, and reported quantitative or qualitative outcomes relating to the factors considered in the evaluation of DMHTs. Only studies published in 2017 or later were included because of the rapidly evolving research area. Eligible studies reported on MH, Alzheimer disease, epilepsy, ASD, or ADHD from user, payer, or employer perspectives (Table S8 in Multimedia Appendix 1 ). While the primary focus of the SLR was MH, neurological conditions were also of interest because their pathologies, symptoms, and treatment strategies can overlap with those of mental illnesses. Alzheimer disease, epilepsy, ASD, and ADHD were selected because they are highly researched and represent diverse types of neurological conditions.
The titles and abstracts of records were assessed for inclusion against these eligibility criteria by 2 independent reviewers, and discrepancies were resolved by consensus, with arbitration by a third reviewer if necessary. Full texts of potentially relevant articles were acquired and screened using the same methodology.
Study Prioritization
Due to the large volume of the evidence identified, additional eligibility criteria were applied to prioritize primary research on theoretical DMHT valuation factors. In line with the thematic framework synthesis objective, theoretical valuation factors were defined as user or DMHT attributes that impact interaction with or perception of DMHTs; therefore, studies that reported only efficacy outcomes, such as mental illness symptom improvement, were deprioritized for full-text review. Secondary research was also deprioritized for full-text review. Studies that reviewed a select app against a framework and studies that reported only the outcomes specific to a select app were deprioritized for data extraction. For example, a study reporting the usability of a specific app’s features would have been deprioritized, while a study reporting what types of features increase MH app usability in general would not.
Data Extraction
All relevant data were extracted into a prespecified Microsoft Excel grid, and a quality assessment was performed for each study. Studies that reported only qualitative data were assessed with the Critical Appraisal Skills Program Qualitative Studies Checklist. Studies that reported only quantitative data were evaluated with the Critical Appraisal Skills Program Cohort Study Checklist, and studies reporting both qualitative and quantitative data were evaluated with both checklists [ 8 ]. Data extractions and quality assessments were performed by a single individual for each study, with the information verified by a second independent individual. Discrepancies were resolved by consensus, with arbitration by a third individual if necessary.
Framework Synthesis
A framework synthesis approach was undertaken to synthesize qualitative and quantitative data identified from the SLR. In line with the “best fit” framework synthesis approach, data were indexed deductively against an existing framework where possible, and novel themes were added inductively as needed [ 9 , 10 ]. The American Psychiatric Association (APA) Mental Health App Evaluation framework was considered the most appropriate framework to address the research objectives of this SLR because its key valuation themes were developed using psychiatrist and patient input, are broadly shared by other evaluation frameworks, are widely acknowledged in the literature, and have been described as durable and adaptable [ 11 - 13 ].
The APA model follows a hierarchical and chronological order whereby the evaluator moves through the framework using prompting questions (eg, “Does the app work offline?”). For this SLR, these questions were either thematically grouped into subthemes or left as prompting questions, as appropriate. The framework was therefore ultimately adapted into 3 levels: themes, subthemes, and more granular valuation criteria. It should be emphasized that this SLR did not aim to formally develop an updated framework to be used in practice by HCPs and their patients but rather was used to form a theoretical basis for understanding DMHT valuation factors, for which novel themes were expected to emerge.
A data-based convergent approach was used to synthesize quantitative and qualitative data [ 14 ]. Data were initially indexed deductively against the prespecified themes within the data collection instrument and then further synthesized within Docear [ 15 ], a mind-map software used to organize and connect data and concepts. Indexing was performed by 1 reviewer and checked by a second independent reviewer. New themes and subthemes that emerged from the literature through inductive coding were added post hoc to the thematic framework, with all extracted data then considered against both the prespecified and novel themes. The evidence identified for each theme was synthesized narratively, taking into consideration the context and design of each study.
Included Studies
A total of 4974 records were retrieved from the electronic databases. Of the 3374 (67.83%) unique records identified following deduplication across the databases, 2891 (85.68%) were excluded based on the eligibility criteria, and an additional 456 (13.52%) were deprioritized because they were not directly related to the topic of interest for this SLR. Excluded and deprioritized full texts are listed in Tables S9 and S10 in Multimedia Appendix 1 , respectively. Therefore, 27 (0.54%) articles were included from the electronic database searches. In addition, 1 article reporting on the same study as an already-included conference abstract was identified during supporting targeted searches and included as a supplementary record, resulting in a total of 28 articles reporting on 26 unique studies (Figure S1 in Multimedia Appendix 1 ). No relevant FDA appraisals were identified in the supplementary search.
Of the 26 included studies, 8 (31%) were quantitative, 12 (46%) were qualitative, and 6 (23%) used a mixed methods approach. While 5 (19%) studies assessed prospective cohorts, 22 (85%) used a cross-sectional approach, including 1 (4%) study that contained both a prospective cohort and a cross-sectional cohort ( Table 1 ). All studies (26/26, 100%) investigated a user perspective, with none specifically investigating payer or employer perspectives. Only 1 (4%) study, which examined ingestible sensor pills and smart pill dispensers to track adherence, investigated a DMHT that was not an app [ 16 ].
Study (author, year) | Design | Perspective and population | Objectives | Data collection methods |
Afra et al [ ], 2018 | Cross-sectional, quantitative | To develop a drug-device combination product using an app in combination with antiseizure medications as an epilepsy treatment | Custom survey | |
Beard et al [ ], 2019 | Cross-sectional, quantitative | , BD , anxiety, OCD , stress-related disorders, and psychotic disorders (N=322) | To characterize general smartphone app and social media use in an acute transdiagnostic psychiatric sample with high smartphone ownership, characterize current engagement and interest in the use of smartphone apps to support MH , and test demographic and clinical predictors of smartphone use | Custom survey |
Borghouts et al [ ], 2022 | Cross-sectional, mixed methods | : members of the Center on Deafness Inland Empire, comprised people with lived experience as members of the deaf or hard-of-hearing community (N=10) | To investigate the MH needs of the deaf or hard-of-hearing community and how MH apps might support these needs | Custom survey; focus group |
Boster and McCarthy [ ], 2018 | Cross-sectional, qualitative | recruited through social media and professional listserves (N=8) | To gain insight from speech-language pathologists and parents of children with ASD regarding appealing features of augmentative and alternative communication apps | Focus groups; poll questions |
Buck et al [ ], 2021a | Cross-sectional, quantitative | referrals or ads (N=43) | To assess caregivers’ interest in an array of specific potential mHealth functions to guide the development of mHealth for caregivers of young adults with early psychosis | Custom survey |
Buck et al [ ], 2021b | Cross-sectional, quantitative | To understand the needs, interests, and preferences of young adults with early psychosis regarding mHealth by surveying interest in mHealth features and delivery modalities and by collecting information about their digital and web-based behaviors | Custom survey | |
Carpenter-Song et al [ ], 2018 | Prospective cohort, qualitative | To examine current practices and orientations toward technology among consumers in 3 mental health settings in the United States | Semistructured interviews | |
Casarez et al [ ], 2019 | Cross-sectional, qualitative | To explore how the well-being of spouses and partners of patients with BD can be improved through mHealth technology | Focus groups; minimally structured, open-ended individual interviews | |
Connolly et al [ ], 2018 | Cross-sectional, qualitative | , alcohol use disorder, or MDD during the previous year at 9 community-based VA outpatient clinics (N=66) | To examine veterans’ attitudes toward smartphone apps and to assess whether openness toward this technology varies by age or rurality | Semistructured interviews informed by the State of the Art Access Model |
Cummings et al [ ], 2019 | Cross-sectional, qualitative | treatment at 4 safety-net clinics (N=37) | To examine stakeholder perspectives regarding whether mHealth tools can improve MH treatment for low-income youth with ADHD in safety-net settings and what functions would improve treatment | Focus groups (caregivers) and interviews (HCPs and staff), both semistructured and including open-ended questions and targeted probes |
Dinkel et al [ ], 2021 | Cross-sectional, qualitative | To explore patient and clinic-level perceptions of the use of depression self-management apps within an integrated primary care setting | Semistructured focus groups; semistructured interviews | |
Forma et al [ ], 2022 | Cross-sectional, quantitative | To assess caregivers’ preferences and willingness to pay for digital (ingestible sensor pill, medication containers with electronic monitoring, mobile apps, and smart pill dispensers) and nondigital (medication diary and simple pill organizer) tools | Custom discrete choice experiment survey | |
Hoffman et al [ ], 2019 | Prospective interventional, mixed methods | To test the feasibility of using mHealth apps to augment integrated primary care services, solicit feedback from patients and providers to guide implementation, and develop an MH app toolkit for system-wide dissemination | Custom survey | |
Huberty et al [ ], 2022 | Cross-sectional (current Calm (Calm.com, Inc) users) and prospective interventional (nonusers of Calm, HCPs), qualitative | : patients with cancer and survivors of cancer with smartphones, some of whom were current subscribers of Calm, a meditation app (N=17) | To develop a mobile meditation app prototype specifically designed for patients with cancer and survivors of cancer | Custom surveys; focus groups |
Kern et al [ ], 2018 | Cross-sectional, quantitative | : students from a midwestern university with smartphones (N=721) | To investigate the potential usefulness of MH apps and attitudes toward using them | Custom survey |
Knapp et al [ ], 2021 | Prospective cohort, qualitative | To learn about considerations and perspectives of community behavioral HCPs on incorporating digital tools into their clinical care for children and adolescents | Focus groups | |
Kornfield et al [ ], 2022 | Prospective cohort, qualitative | or GAD-7 questionnaires, but without serious mental illnesses (eg, BD, schizophrenia), who were not receiving formal care and recruited upon completing free web-based MH self-screening surveys hosted by Mental Health America (N=28) | To investigate how digital technologies can engage young adults in self-managing their MH outside the formal care system | Web-based asynchronous discussion; synchronous web-based design workshop |
Lipschitz et al [ ], 2019 | Cross-sectional, quantitative | To assess patients’ interest in mHealth interventions for MH, to identify whether provider endorsement would impact interest, to determine reasons for nonuse of mHealth interventions for MH, and to identify what mHealth content or features are of most interest to patients | Custom survey | |
Mata-Greve et al [ ], 2021 | Cross-sectional, mixed methods | : essential workers during the COVID-19 pandemic or workers who were unemployed or furloughed because of the COVID-19 pandemic, recruited from a web-based research platform (N=1987) | To document psychological stress, to explore DMHT use in response to COVID-19–related stress, to explore the usability and user burden of DMHTs, and to explore which aspects and features of DMHTs were seen as necessary for managing stress during a pandemic by having participants design their own ideal DMHTs | Survey combining custom and validated measures (System Usability Scale, Use Burden Scale) |
Melcher et al [ ], 2022 and Melcher and Torous [ ], 2020 | Cross-sectional, mixed methods | : college students aged 18-25 years, recruited through social media and word of mouth (N=100) | To examine why college students show poor engagement with MH apps and how apps may be adapted to suit this population | Custom survey; interviews |
Schueller et al [ ], 2018 | Cross-sectional, mixed methods | : smartphone owners recruited from a research registry (N=827) | To understand where users search for MH apps, what aspects of MH apps they find appealing, and what factors influence their decisions to use MH apps | Custom survey; focus group interviews |
Schueller et al [ ], 2021 | Cross-sectional, qualitative | : participants who had used an app that allowed them to track their mood, feelings, or mental well-being for ≥2 weeks, recruited from a research registry (N=22) | To understand motivations for and experiences in using mood-tracking apps from people who used them in real-world contexts | Semistructured interviews |
Stiles-Shields et al [ ], 2017 | Cross-sectional, qualitative | : participants recruited from web-based postings; approximately equal numbers of participants were above and below the criteria for a referral for psychotherapy for depression (N=20) | To identify the barriers to the use of a mobile app to deliver treatment for depression and to provide design implications on the basis of identified barriers | Card sorting task |
Storm et al [ ], 2021 | Cross-sectional, qualitative | To identify stakeholders’ perspectives on partnering to inform the software development life cycle of a smartphone health app intervention for people with serious mental illness | Semistructured interviews | |
Torous et al [ ], 2018 | Cross-sectional, quantitative | To understand how individuals with mental illness use their mobile phones by exploring their access to mobile phones and their use of MH apps | Custom survey | |
Zhou and Parmanto [ ], 2020 | Cross-sectional, mixed methods | To determine user preferences among the several privacy protection methods used in current mHealth apps and the reasons behind those preferences | Custom survey; interview |
a Only information relevant to this systematic literature review is reported in this table.
b MDD: major depressive disorder.
c BD: bipolar disorder.
d OCD: obsessive-compulsive disorder.
e MH: mental health.
f General users are participants who were not necessarily diagnosed with indications of interest.
g ASD: autism spectrum disorder.
h HCP: Health care provider.
i mHealth: mobile health.
j PTSD: posttraumatic stress disorder.
k VA: Veterans Affairs.
l ADHD: attention-deficit/hyperactivity disorder.
m PHQ-9: Personal Health Questionnaire-9.
n GAD-7: Generalized Anxiety Disorder-7.
o DMHT: digital mental health technology.
Most frequently, studies focused on indications for mood, anxiety, or psychotic disorders (15/26, 58%), with other indications of focus including ADHD (2/26, 8%), ASD (1/26, 4%), and epilepsy (1/26, 4%). No relevant studies focused on Alzheimer disease were identified.
A total of 8 (31%) studies assessed the perspectives toward DMHTs of general population participants who were not necessarily diagnosed with relevant conditions [ 19 , 28 , 29 , 33 - 37 ]. Of these populations, several were identified as having an increased risk of MH conditions, such as patients with cancer [ 28 ], college students [ 29 , 34 ], deaf or hard-of-hearing individuals [ 19 ], and people who were unemployed or furloughed during the COVID-19 pandemic [ 33 ]. In addition, 1 (4%) study included a mix of patients who were above and below the referral criteria for psychotherapy for depression [ 37 ].
Thematic Analysis
Evidence was identified for all 5 themes included in the APA framework: engagement style (23/26, 88%), background and accessibility (16/26, 62%), privacy and security (13/26, 50%), therapeutic goal (12/26, 46%), and clinical foundation (8/26, 31%; Table 2 ). Five novel criteria were identified and added to the framework post hoc, 1 each under engagement style (forgetting or feeling unmotivated to use DMHTs) and privacy and security (personal image and stigma) and 3 under background and accessibility (willingness to pay, insurance restrictions, and cost savings compared with professional care).
Subtheme | Criteria (study reference) | ||
Short-term usability | , , , ] - , , , , , , ] | ||
Long-term usability | , - , , , - , - ] [ , , , ] | ||
Customizability | , , , , , , ] | ||
Technical | , , , , , ] , , , - , , , ] | ||
Business model | | ||
Costs | , ] , , , ] [ ] [ ] - , ] | ||
Medical claims | | ||
Stability | , ] | ||
No specific subtheme | , , ] | ||
Data collection and storage | , , , , ] | ||
Privacy policy | , , ] ] ] | ||
Personal health information | ] , , , ] | ||
Security measures | , , ] | ||
Impressions of use | , ] | ||
User feedback | , ] | ||
Clinical validity | , ] , - ] , , ] | ||
Clinically actionable | , , - , , , , ] - , ] | ||
Therapeutic alliance | , , , , , ] , ] | ||
Data ownership, access, and export | |
a Novel findings that emerged from this systematic literature review.
b These subthemes and criteria were included in the American Psychiatric Association’s framework but were not reported on by studies included in this systematic literature review.
c HCP: health care provider.
Theme 1: Engagement Style
Engagement style was the most reported theme, with evidence identified from 23 (88%) of the 26 studies. Engagement style encompasses how and why users do or do not interact with DMHTs. The long-term usability subtheme was reported by 96% (22/23) of studies, short-term usability by 12 (52%) studies, and customizability by 7 (30%) studies. Findings from short- and long-term usability subthemes were highly interconnected.
A total of 4 studies reported that ease of use promoted short-term DMHT engagement. In the study by Schueller et al [ 35 ], 89.6% of a general population of smartphone users reported ease of use for MH apps as “important” or “very important,” and users qualitatively reported dislike of “overwhelming,” difficult-to-navigate apps. In addition, users valued apps that were “simplistic” [ 34 ], fit into their daily schedules, and were available when needed (eg, during acute symptom experiences) [ 5 , 25 ]. Select supporting qualitative data are presented in Table 3 .
Subtheme and criteria: findings | Key quotes | |||
Ease of use | ] ] | |||
Available engagement styles: use of animation and visuals | ] ] [ ] | |||
Alignment of app with needs and priorities: gamification | ] | |||
Alignment of app with needs and priorities: anxiety management | center peer support specialist] [ ] ] | |||
Alignment of app with needs and priorities: tracking mood, symptoms, or sleep | ] ] [ ] | |||
Alignment of app with needs and priorities: social media–like features | ] | |||
Alignment of app with needs and priorities: peer support and chat functions | ] ] [ ] | |||
Forgot or unmotivated to use | ] ] ] | |||
Accessibility: mobility barriers | ] | |||
Accessibility: technical literacy | ] | |||
Offline functionality: internet and mobile data access as a barrier to use | ] ] | |||
Willingness to pay | ] ] | |||
Security associated with collection, use, and transmission of sensitive data (including personal health information) | ] ] | |||
Transparency and accessibility of privacy policy | ] | |||
Personal image and stigma | that is protected in the same way my EMR is protected.” [Patient in routine behavioral health care] [ ] | |||
Security systems used | ] | |||
Positive change or skill acquisition: apps that impart skills and encourage positive change, in an easy way | ] in cancer care] [ ] | |||
Ease of sharing and interpretation of data: increase of engagement and symptom reporting | ] | |||
Therapeutic alliance between patient and HCP | ] | |||
Evidence of specific benefit: HCP recommendations | ] | |||
Evidence of specific benefit: increased usage if supported by research, academic institution, or reputable professional society | ] ] |
a ASD: autism spectrum disorder.
b MH: mental health.
c ADHD: attention-deficit/hyperactivity disorder
d BD: bipolar disorder.
e Novel criteria identified by this systematic literature review.
f CHA: Cambridge Health Alliance.
g EMR: electronic medical record.
h HCP: health care provider.
Users valued DMHT features that aligned with their needs and priorities, as reflected by findings within the long-term usability subtheme. Across 9 studies, quantitative and qualitative findings demonstrated high interest in anxiety management features such as relaxation tools, breathing exercises, and mindfulness or meditation activities, and 10 studies identified interest in mood, symptom, or sleep tracking ( Tables 3 and 4 ). While most studies (24/26, 92%) focused on MH, patients with epilepsy also reported high interest in features to record seizure dates and types [ 17 ]. Importantly, users in 2 studies emphasized the need for developers to tailor DMHTs to the needs and priorities of the target population ( Table 3 ) [ 28 , 31 ]. Relatedly, mixed attitudes were reported toward positive affirmations and words of encouragement, with many users expressing interest but others emphasizing the value of a human component to DMHTs or cautioning against blanket encouragement and automated messages that could feel insincere [ 19 , 25 , 31 ].
Features, study, perspective, and finding | Patients, n (%) | Likert score, mean (SD) | |||||
], 2021b | |||||||
Interest in skill practices for managing stress and improving mood | 64 (84.2) | 3.30 (0.98) | |||||
Interest in skill practices for relaxation | 57 (76) | 3.09 (1.12) | |||||
Interest in information about relaxation exercises | 59 (77.6) | 3.00 (1.16) | |||||
Interest in information about healthy sleep practices | 56 (73.7) | 2.93 (1.15) | |||||
Interest in mindfulness or meditation practices | 44 (59.4) | 2.61 (1.34) | |||||
], 2018 | |||||||
Interest in music to help seizure control | — (75) | — | |||||
Interest in relaxing music that may help alleviate stress | — (68) | — | |||||
Interest in relaxing imagery that may help alleviate stress | — (40) | — | |||||
Interest in drawing or writing while listening to music | — (35) | — | |||||
Interest in practicing mindfulness | — (63) | — | |||||
], 2018 | |||||||
Comfort level for mindfulness and therapy | — | 3.75 | |||||
Comfort level for mindfulness and therapy | — | 3.17 | |||||
], 2019 | |||||||
Current use of an MH app with the primary purpose being mindfulness or meditation | — (71) | — | |||||
], 2021 | |||||||
Most frequently endorsed mindfulness tools as a feature when provided options to build their own app | 687 (67.8) | — | |||||
Most frequently endorsed mindfulness tools as a feature when provided options to build their own app | 584 (60) | — | |||||
Most frequently endorsed mindfulness tools as a feature when provided options to build their own app | 305 (61.4) | — | |||||
Most frequently endorsed mindfulness tools as a feature when provided options to build their own app | 966 (65.3) | — | |||||
], 2019 | |||||||
The ability to manage mood, anxiety, or substance use through the use of DMHTs was seen as a benefit of incorporating DMHTs into clinical care | 13 (57) | — | |||||
], 2018 | |||||||
Willingness to use an MH app to track mood or anxiety | 41 (10.3) | — | |||||
], 2018 | |||||||
Interest in a diary to record the date of seizures | — (85) | — | |||||
Interest in a digital diary to record the type of seizure | — (73) | — | |||||
Interest in digital diary to log the missed dosages of their medications | — (78) | — | |||||
], 2019 | |||||||
, or PTSD | |||||||
Interested in progress monitoring (track mood, stress, anxiety, or PTSD symptoms) | 95 (63.8) | — | |||||
Interested in progress monitoring (track mood, stress, anxiety, or PTSD symptoms) | 80 (67.2) | — | |||||
], 2021b | |||||||
Interest in a feature to set and track goals | 60 (78) | 3.10 (1.05) | |||||
Interest in a feature to track symptoms over time | 70 (90.9) | 3.44 (0.90) | |||||
Interest in a feature to track changes in progress toward goals | 66 (86.9) | 3.37 (0.86) | |||||
Interest in a feature to track wellness behaviors (eg, steps or activity) | 48 (64.9) | 2.86 (1.22) | |||||
], 2019 | |||||||
Current use of an MH app with the primary purpose being mood tracking | — (10) | — | |||||
Willingness to use an MH app daily to monitor condition | 262 (81) | — | |||||
Willingness to use an MH app daily to monitor condition | — (85) | — | |||||
Willingness to use an MH app daily to monitor condition | — (77) | — | |||||
], 2021 | |||||||
Most frequently endorsed symptom tracking (tracking sleep or mood) as a feature when provided options to build their app | 605 (59.7) | — | |||||
Most frequently endorsed symptom tracking (tracking sleep or mood) as a feature when provided options to build their app | 555 (57) | — | |||||
Most frequently endorsed symptom tracking (tracking sleep or mood) as a feature when provided options to build their app | 270 (54.3) | — | |||||
Most frequently endorsed symptom tracking (tracking sleep or mood) as a feature when provided options to build their own app | 890 (60.2) | — | |||||
], 2018 | |||||||
Comfort level for in-app symptom surveys | — | 3.50 | |||||
Comfort level for in-app symptom surveys | — | 3.11 | |||||
Comfort level for passive call or text monitoring | — | 2.32 | |||||
Comfort level for passive call or text monitoring | — | 2.39 | |||||
Comfort level for passive GPS monitoring | — | 2.31 | |||||
Comfort level for passive GPS monitoring | — | 2.78 |
a A 5-point Likert scale (0-4) was used.
b Not available.
c A 5-point Likert scale (1-5) was used.
d MH: mental health.
e DMHT: digital mental health technology.
f MDD: major depressive disorder.
g PTSD: posttraumatic stress disorder.
Both patients and caregivers expressed interest in psychoeducational content that aligned with their needs and priorities. When surveyed, >60% of veterans with anxiety or major depressive disorder (MDD), patients with epilepsy, young adults with psychosis, and essential and furloughed workers during the COVID-19 pandemic expressed interest in relevant psychoeducational content [ 17 , 22 , 32 , 33 ]. In contrast, only 4% of college students in another study reported using an MH app for information about MH, although an MH diagnosis was not required for study participation [ 29 ].
Caregivers of young adults with psychosis, caregivers of children with ADHD, and spouses and partners of people with bipolar disorder (BD) were all interested in information related to caring for the individual with the given disorder, such as information on psychological and pharmacological treatments, symptoms and symptom changes, and the MH system [ 21 , 24 , 26 ]. Comparatively smaller, but still notable, proportions of caregivers of patients with psychosis were interested in caregiver-focused information; for instance, 62% to 69% were interested in relaxation exercises, stress and mood management, and community events for caregivers, while 85% to 90% were interested in the aforementioned patient-focused information [ 21 ].
Information delivery–style preference was captured under the short-term usability subtheme. One study in young adults with psychosis and another study with their caregivers revealed that delivering information in a variety of formats was important; when presented with nonmutually exclusive options, >50% of both populations were interested in text content, video content, audio content, and discussion boards [ 21 , 22 ].
Social interaction promoted long-term engagement. Qualitatively, 3 studies found that users valued learning about similar experiences from others via social media–like features, which normalized their experiences and could provide new symptom management strategies ( Table 3 ) [ 28 , 31 , 36 ]. Similarly, 67% of both young adults with psychosis and deaf or hard-of-hearing survey participants (N=9) reported interest in peer support via chat features [ 19 , 22 ]. However, a comparatively smaller proportion of veterans with anxiety or MDD (48.3% of the full cohort and 51.3% of the smartphone user subgroup) were interested in peer support [ 32 ].
Overall, users endorsed social features to support their MH. In the study by Casarez et al [ 24 ], spouses and partners of people with BD likewise desired features to communicate with other caregivers and also emphasized that DMHTs could facilitate conversation and understanding with patients, a sentiment echoed by peer support specialists by Storm et al [ 38 ] ( Table 3 ). However, one oncology HCP cautioned that similar to support groups, “very strict guidelines of what is said” should be implemented to manage potential risks from shared social media–like content, although little additional context was provided [ 28 ].
Spouses and partners of people with BD also suggested both in-app information on accessing professional resources and direct counseling for the patient at times when other support might be inaccessible [ 24 ]. More than half of all workers, employed or unemployed during the COVID-19 pandemic, likewise endorsed links to resources, counseling, and crisis support as DMHT features, and 81.6% of young adults with psychosis endorsed a feature to communicate with professional experts [ 22 , 33 ]. Importantly, compared with patients attending public clinics, patients attending private psychiatric clinics expressed a higher comfort level for in-app communication with HCPs, suggesting demographic differences in the valuation of access to professional support through DMHTs [ 39 ].
A total of 9 studies reported an interest in DMHT reminders and notifications. Across 3 studies, >70% of patients or caregivers were interested in appointment reminders [ 17 , 21 , 22 ]. In addition, 73% and 68% of patients with epilepsy reported interest in reminders for medication refills and adherence, respectively [ 17 ]. Beyond apps, caregivers of patients with MDD, BD, and schizophrenia preferred an ingestible pill sensor that tracked medication adherence, physical activity, mood, and rest 9.79 (95% CI 4.81-19.9), 7.47 (95% CI 3.81-14.65), and 6.71 (95% CI 3.29-13.69) times more than a nondigital pill organizer, respectively [ 16 ]. Qualitatively, patients and caregivers also appreciated reminders, especially if reasonably timed or delivered via text messages [ 27 , 31 ].
Short-term DMHT engagement was also supported by games and graphics, which could communicate information in an accessible way [ 24 ], provide tools for stress management [ 17 , 33 ], and be used therapeutically with children [ 20 , 30 ]. However, some HCPs and caregivers expressed concerns that graphics and games may be distracting for certain children ( Table 3 ) [ 20 ].
In a novel finding, 3 studies reported forgetfulness or lack of motivation as an influence on DMHT engagement. In some cases, disuse was related to stress, other MH symptoms, or poor technical literacy ( Table 3 ) [ 5 , 25 , 31 ]. In contrast, “forgetting to use” DMHTs and “lack of motivation” were perceived as relatively small barriers to use in the study by Stiles-Shields et al [ 37 ].
The third subtheme under engagement style was customizability, which was generally valued by users; 70.9% of a general population of smartphone users noted customization was an important factor [ 35 ]. Similarly, 9.4% of all surveyed veterans and 10.9% of those with smartphones reported disliking a prior DMHT due to a lack of personalization [ 32 ]. Users specifically wanted to be able to opt out of irrelevant features, customize audiovisual and design elements, add personal notes to tracked mood data, and provide ongoing feedback to facilitate personalization [ 20 , 24 , 28 , 31 , 34 ].
Theme 2: Background and Accessibility
A total of 16 (62%) studies reported findings related to DMHT background and accessibility, which considers the developer of the DMHT, as well as functionality and accessibility. Of these, 12 (75%) studies reported on the technical considerations subtheme, 9 (56%) on costs, and 2 (13%) on stability.
Under technical considerations, 9 studies assessed diverse accessibility concerns. Broadly, Storm et al [ 38 ] emphasized that DMHTs should be developed in consideration of patients’ social, cognitive, and environmental needs to avoid overwhelming users. Specifically, 2 studies reported language as a barrier. Deaf or hard-of-hearing participants recommended visual content presentation, such as videos and icons, alongside text and American Sign Language translations where possible [ 19 ]. Similarly, when discussing English-only apps, 1 provider stated as follows: “language is a barrier for some [patients]” [ 5 ]. Mobility issues related to MH symptoms or other conditions and technical literacy, such as difficulties remembering passwords and navigating smartphones or apps, created accessibility barriers as well ( Table 3 ) [ 5 , 25 , 27 , 28 ]. Additional concerns included apps that restricted use based on geographic location [ 19 ], user difficulty in finding relevant, useful apps [ 32 ], and limited mobile device memory for downloading apps [ 5 , 19 ].
Offline functionality, reported by 6 studies, was also captured under the technical considerations subtheme. A majority (5/9, 56%) of participants included in the study by Borghouts et al [ 19 ] expressed concern about their mobile data plans when using their devices. Correspondingly, “availability of Wi-Fi” was noted as a top barrier to the use of apps for depression by Stiles-Shields et al [ 37 ], and several veterans in another study reported that home Wi-Fi connectivity facilitated app use by eliminating cellular data fees [ 25 , 37 ]. Quotes from patients and HCPs echoed the concern about apps without offline functionality ( Table 3 ) [ 23 , 30 ].
Data fees were also captured under the costs subtheme, with hidden or additional costs described as a barrier to app use by 2 studies [ 26 , 37 ]. Parents of children with ADHD reported that difficulty paying phone bills could result in their phones being shut off, limiting DMHT use; one MH clinic administrator stated as follows: “We often encounter parents’ phones being shut off because they haven’t paid their bill...If the app were free or low cost, I imagine it could be very helpful” [ 26 ]. In addition to hidden costs, this quote identifies up-front app costs as a barrier. Quantitatively, more than half of a general population of surveyed college students expressed that cost was a top concern for the use of MH apps [ 34 ]. Qualitative findings from 2 additional studies likewise identified cost as a barrier to DMHT use [ 25 , 27 ].
Three novel cost attributes were identified by this SLR: willingness to pay, insurance restrictions, and cost savings compared with professional care. Four studies, 3 of which focused on apps, explored willingness to pay for DMHTs from a user perspective. Willingness to pay varied based on user preference; some surveyed college students and smartphone users among general populations valued free apps due to financial restrictions or uncertainty around app effectiveness, although 1 student commented that the quality of free trials might be inferior [ 34 , 35 ]. Some smartphone users also voiced a limit on how much they would be willing to spend for an app subscription ( Table 3 ) [ 35 ]. Forma et al [ 16 ] found that caregivers were willing to pay US $255.04 (95% CI US $123.21-US $386.86) more per month for a pill with an ingestible sensor that tracked medication adherence, physical activity, and rest and could connect to an app that also collected self-reported mood data. Moreover, the caregivers were willing to pay US $124.50 (95% CI US $48.18-US $200.81) more per month for an app-connected pill organizer alone than for a nondigital pill organizer [ 16 ]. In contrast, some veterans expressed total disinterest in paid apps, with 1 user citing poor technical literacy (“don’t have the knowledge”) in addition to cost as affecting willingness to pay [ 25 ].
In another novel finding, a speech-language pathologist working with children with ASD preferred a single app including multiple features over separate apps for particular features due to insurance restrictions: “I agree that teaching Apps should be an in-App feature versus their own app because sometimes insurance doesn’t allow us to open the iPads purchased through insurance” [ 20 ]. Although no further detail was provided for this finding, it suggests that there may be restrictions on the use of other apps on devices purchased under insurance, which may have implications for DMHT use in formal care settings due to the lack of financial support.
In a third novel cost-related finding, a small number of participants from a general population of students (3.6%) in one study preferred using an MH app to seeing an MH professional due to cost savings [ 29 ].
A total of 13% (2/16) of studies reported on the subtheme of app stability and technical difficulties, with crashes and poor display quality decreasing DMHT value [ 35 , 37 ]. Participants in the study by Schueller et al [ 35 ] reported that technical difficulties were often an issue for apps developed by medical institutions, which might be effective and safe but less usable than apps from other developers.
Theme 3: Privacy and Security
A total of 13 (50%) out of 26 studies reported findings related to the privacy and security theme, which covered the use and protection of user data by DMHTs. Subthemes were reported relatively equally: data collection and storage (5/13, 38%), personal health information (PHI; 5/13, 38%), privacy policies (4/13, 31%), general privacy (3/13, 23%), and security measures (3/13, 23%).
Quantitative and qualitative findings on general privacy (ie, evidence not categorized under any specific subtheme), the data collection and storage subtheme, and the privacy policies subtheme revealed heterogeneous concerns ( Table 3 ). A total of 74% of a general population of college students reported privacy as a top concern for MH apps, although further details on the specific area of concern were unclear [ 34 ]. In the study by Stiles-Shields et al [ 37 ], participants were highly concerned with data access but less so with general privacy. Echoing the concerns about data collection and storage, 59.1% of veterans with anxiety or MDD in 1 study were concerned about in-app PHI protection [ 32 ]; however, a qualitative study in veterans with posttraumatic stress disorder, alcohol use disorder, or MDD reported that a relatively small number of participants expressed privacy concerns. In the latter study, reasons for the concerns included distrust in Veterans Affairs, belief that digital data are inherently not confidential, and fear of phone hacking [ 25 ]. From an HCP perspective, none of the surveyed behavioral health HCPs agreed with the statement “My patients are concerned about data security,” despite multiple patients within the same study reporting privacy concerns [ 5 ].
Still, privacy policies were important overall, with 70.5% of smartphone MH app users rating having a privacy policy as “very important” or “important” [ 35 ]. Melcher et al [ 34 ] found that although users valued data protection, some reported a lack of awareness about data privacy, and others were concerned about obscure privacy policies and PHI use. As noted in the data collection and storage subtheme, veteran concerns about government use of PHI were heterogeneous [ 25 ].
A novel valuation factor not included in the APA framework related to user concern with PHI privacy and security regarding MH diagnoses and MH app use is a desire to upkeep their personal image or avoid stigma ( Table 3 ) [ 5 , 25 , 29 , 40 ]. For instance, 21.1% of a general college student population preferred MH app use to seeing an MH professional due to anonymity or reduced stigma [ 29 ]. One participant in a study of Veterans Affairs health service users described access to professional care via MH apps as convenient because they could avoid disclosing their use of MH services to explain leaving work early for an appointment [ 25 ].
In line with the overarching concern about PHI privacy and security, users valued app security measures. Schueller et al [ 35 ] reported that 74.2% of users rated data encryption as “important” or “very important.” Users in another study perceived the level of privacy protection as the highest for apps using a combination of a generic app name (ie, not reflecting the indicated MH disorder); easily hidden modules; and secure, user-authenticated web portals for making module changes [ 40 ]. Behavioral health clinic staff echoed the importance of discreet MH app names ( Table 3 ) [ 30 ].
Theme 4: Therapeutic Goal
There were 12 (46%) studies that reported on the factors relating to the integration of DMHTs with users’ therapeutic goals. The clinical actionability and therapeutic alliance subthemes were reported by 83% (10/12) and 58% (7/12) of studies, respectively.
A total of 9 studies reported the value of clinically actionable insights from apps where the users could acquire and practice new skills to make positive changes in their lives ( Table 3 ). For instance, patient and caregiver app users reported interests in “daily tips,” “new ideas,” and “solutions or recommendations” for symptom management [ 26 , 27 , 36 ]. Furthermore, an app that could serve as a resource for multiple management strategies was preferable [ 26 , 28 , 31 ]. Quantitatively, 4% of patients receiving acute treatment in a partial hospitalization program for MH conditions, including mood and psychotic disorders, reported that the primary purpose of their DMHT use was therapy skills practice [ 18 ]. HCPs similarly appreciated that DMHTs could facilitate patients practicing skills outside of formal treatment sessions [ 5 ]. In particular, clinicians from a youth behavioral health clinic noted that DMHTs might be especially beneficial for young users because they could be conveniently and discreetly incorporated into their daily lives [ 30 ].
Users valued easy data sharing with clinicians, particularly for mood- or symptom-tracking features, which could improve communication and the accuracy of symptom reporting during clinical visits [ 5 , 25 - 27 , 34 , 36 ]. For instance, 53% of a general college student population believed that the potential to share information with their clinician was “one of the top benefits” of using DMHTs [ 34 ]. In addition, many HCPs reported active use or interest in the use of DMHTs in clinical practice to facilitate asynchronous communication and increase patient engagement with treatments outside of formal appointments; however, some preferred traditional care strategies for their personalization and flexibility ( Table 3 ) [ 5 , 26 , 30 ].
Theme 5: Clinical Foundation
A total of 8 (31%) studies reported findings related to the clinical foundation of DMHTs, that is, their utility and appropriateness for patients. Clinical validity was the most reported subtheme, with evidence identified from 6 (75%) studies; 2 (25%) studies reported on the user feedback subtheme and 2 (25%) on the impressions of use subtheme, which captured users’ perceptions of app content as accurate and relevant.
Across subthemes, users valued evidence of DMHT benefit or efficacy from various sources. A total of 71.8% of surveyed veterans said that they would use a DMHT if they “saw proof that it worked” for their MH conditions [ 32 ]. Similarly, among the 811 general population participants surveyed, 69.5% ranked direct research evidence as “important” or “very important” for DMHT, and 66.8% ranked indirect research evidence the same [ 35 ]. Qualitative data identified recommendations from HCPs or academic institutions, as well as evidence of DMHT benefit from publications or research studies, as specific sources for clinically valid evidence of benefits ( Table 3 ) [ 27 , 34 , 35 ].
In addition to academic and professional support, the user feedback subtheme captured user interest in whether DMHTs were beneficial for peers or recommended by other trusted individuals. Patients with depression reported that other users’ experiences influenced their app use, with one user wanting to know “...if other people had success using it” [ 27 ]. Quantitatively, user ratings and user reviews were ranked as “important” or “very important” factors in DMHT use by 59.4% and 58.7% of the general population participants, respectively [ 35 ].
Quality Assessment
The risk of bias was overall moderate. Of the 14 studies including quantitative components, only 1 (7%) used relevant validated outcome measurement instruments [ 33 ]; all others used custom questionnaires. Of the 18 studies with qualitative components, 4 (22%) were at risk of selection bias due to participants being exclusively recruited using web-based postings and research registries [ 33 - 35 , 37 ], and only 1 (6%) considered the relationship between researcher and participant when interpreting the results [ 36 ]. Full quality assessments for qualitative and quantitative study components can be found in Tables S11 and S12 in Multimedia Appendix 1 , respectively.
Principal Findings
This SLR aimed to identify and synthesize qualitative and quantitative evidence on how DMHTs are valued by users, payers, and employers in the United States. Evidence from users with or without diagnosed relevant disorders, caregivers, and HCPs was captured across a wide range of demographics. No study reported evaluating an app from a payer or employer perspective. Furthermore, all but one included study focused on mobile apps.
No relevant appraisals of DMHTs were identified from the FDA website searches; however, 8 relevant FDA approval labels or notifications for MH apps or guidance documents for industry and FDA staff were identified. The content of these materials overlapped with some valuation factors identified in this SLR, including evidence of clinical efficacy and safety, app maintenance, and privacy and security.
Engagement style, although not covered by the FDA materials, was the most reported theme by the studies included in this SLR and was found to overlap heavily with other themes. Engagement may be a key consideration for app developers, as app user retention can be low: 1 study showed that >90% of users had abandoned free MH apps within 30 days of installation [ 41 ]. Engagement is also a key clinical concern in terms of DMHT efficacy; one meta-analysis of 25 studies showed that increased use of DMHT modules was significantly associated with positive outcomes regardless of the target MH condition [ 42 ]. The findings of this SLR may therefore be informative to both DMHT designers and HCPs who integrate DMHTs into clinical care by providing insight on DMHT valuation and thus how use and benefit can be improved. For instance, users valued DMHTs that were easy to use and aligned with their needs and priorities, particularly through features that supported their therapeutic goals. In addition, content presented through multiple delivery modes, such as both text and visuals, promoted engagement as well as accessibility.
However, engagement and feature preference varied across populations. For instance, DMHT valuation was affected by technical literacy, which may relate to user demographics; in this SLR, veterans repeatedly emphasized technical literacy as a barrier to DMHT use [ 25 ]. Similarly, offline functionality may be more important for some users. Although 85% of the total United States population owns smartphones, only 59% of Medicare beneficiaries have access to a smartphone with a wireless plan. Moreover, beneficiaries who are older, less educated, disabled, or Black or Hispanic have even lower digital access [ 43 , 44 ]. These findings emphasize the importance of customizability and suggest that app development and selection in the clinical setting should consider the demographics of the target population, particularly in relation to ease of use and offline functionality.
Background and accessibility findings also identified up-front and hidden costs as barriers to DMHT use, with the willingness to pay varying among individuals. This has important implications for app development, considering that many MH apps currently on the market are direct-to-consumer sales and require out-of-pocket payment. App developers often take this approach as it does not require the accumulation of formal evidence of clinical benefit for FDA approval [ 45 ], but it may present a financial barrier to use for consumers.
Privacy and security, reported by 13 (50%) out of 26 studies, was a prevalent theme, with users primarily concerned with data and PHI security within apps. This finding reflects wider research; a 2019 review of 116 depression-related apps retrieved from iTunes and Google Play stores in 2017 found that only 4% of the identified apps had acceptable transparency in privacy and security, with many completely lacking a privacy policy [ 46 ]. Similarly, 39% of MH apps recommended by college counseling centers had no privacy policy, and of those with a policy, 88% collected user data, and 49% shared that data with third parties [ 4 ]. Most evidence identified in this SLR under this theme, as well as findings previously published in the wider literature, focuses on these remote privacy risks. However, local privacy concerns are also important to users. In particular, inconspicuous naming and the ability to hide sensitive modules within MH apps were rated as highly important by both patients and HCPs to maintain user privacy. Users emphasized a desire to avoid the stigma associated with mental illness, which was also reflected by the findings in the engagement style theme: more young adults with psychosis were more interested in in-app messaging with other patients in psychosis recovery (67.1%) than a provider and family member together (47.3%) or their personal support network (59.8%) [ 22 ]. Similarly, youths were interested in apps that could be used discreetly in school or other public settings to avoid potential MH stigma. This is a key, novel finding of this SLR, considering that many app or DMHT components on the market are named after their target disorder.
The use of DMHTs to achieve therapeutic goals was discussed from patient, caregiver, and HCP perspectives, all of which valued DMHTs that had evidence of efficacy, presented clinically actionable information, and facilitated patient-clinician relationships. Of the 5 studies that explored how HCPs value DMHTs in clinical practice, 2 (40%) were restricted to the oncology or ASD settings and were not readily generalizable to wider MH settings [ 20 , 28 ]. In other studies, providers reported interest in using DMHTs to facilitate asynchronous communication with patients and their caregivers, promote patient skill practice, and improve care for children through the use of games and visuals [ 26 , 30 ]. However, while HCPs overall believed that DMHTs improved care, some believed that their clinical training allowed for care personalization beyond what DMHTs could provide. Feature customizability and receipt of input from HCPs and users during app development and testing may be a way to mitigate these concerns, as well as concerns about safety and efficacy, as many available apps do not appropriately address user health concerns [ 47 ].
Findings additionally suggested that training and resources on DMHTs would be beneficial to ensure that HCPs were equipped to integrate DMHTs into their practices [ 5 ]. Collaboration between DMHT specialists and HCPs, along with a shift from randomized controlled trials to effectiveness-implementation hybrid trials, may be a way to streamline the integration of DMHTs into clinical care and provide more training and resources for HCPs [ 30 , 48 ].
This review followed a prespecified protocol and used systematic methods in line with the York Centre for Reviews and Dissemination guidelines [ 49 ] to conduct an exhaustive search of the literature, identifying evidence relevant to the review objectives from multiple databases and supplementary sources. The framework synthesis approach allowed for the inclusion and analysis of both qualitative and quantitative data, providing a detailed picture of not only what DMHT features users value but why they value them, especially in areas where valuation varies across patient demographics. In addition, the APA framework is a robust model created with patient and HCP input that incorporates key valuation themes broadly shared by other frameworks and widely acknowledged in the literature [ 11 - 13 ].
Limitations
Methodological limitations should be considered when interpreting the findings of this SLR. Only publications in English and in United States populations were included. As perceptions of value are influenced by factors including cultures, laws, and health care settings, the findings of this SLR should not be generalized to other countries. For instance, trust in HCPs and rates of longstanding relationships between patients and primary care providers are lower in the United States than in many European nations [ 50 , 51 ], which could impact the type of support users want from DMHTs (ie, engagement style) or interest in DMHT integration with therapeutic goals.
In addition to the prespecified eligibility criteria, deprioritization strategies were implemented due to the large volume of the identified evidence, and this may have resulted in missing relevant articles. In particular, the deprioritization of secondary research and opinion pieces likely led to the exclusion of relevant discussion around payer perspectives and reimbursement, for which no evidence was included in this SLR. Furthermore, although unlikely, there may have been reporting biases in the included studies due to missing results, which this SLR was not able to assess.
This SLR identified no evidence for 3 subthemes included in the APA framework: business model (background and accessibility), which covers DMHT funding sources and potential sources of conflict, medical claims (background and accessibility), which examines whether DMHTs claim to be medical and the trustworthiness of their creators, and data ownership, access, and export (therapeutic goal), which includes sharing data with eHealth records or wellness devices (eg, Apple HealthKit [Apple Inc], Fitbit [Google LLC]). The valuation of these subthemes should be evaluated in future research.
Conclusions
In summary, app usability, cost, accessibility and other technical considerations, and alignment with therapeutic goals were the most reported valuation factors identified by this SLR. Many studies also reported user preference for apps that incorporated privacy and security features that provided protection from stigma. However, individual DMHTs and their features are valued differently across individuals based on demographics and personal preferences. MH apps should be developed and selected with these specific user needs in mind. Feature customizability and input from users and HCPs during development may improve app usability and clinical benefit.
Acknowledgments
The authors thank Max Lee, Costello Medical, US, for medical writing and editorial assistance based on the authors’ input and direction.
Conflicts of Interest
DCM is a consultant for Otsuka Pharmaceutical Development & Commercialization (OPDC) Inc for this project and has received consulting funds from Pear Therapeutics, Sanofi, Avidity, Sarepta, Novartis, and BioMarin. ML, HG, and HCW are employees of OPDC. JC, SB, RSK, and EW are employees of Costello Medical. This research was funded by OPDC.
Electronic database and supplementary search terms, systematic literature review eligibility criteria, publications excluded or deprioritized at full-text review, quality assessments of included studies, and the PRISMA (Preferred Reporting Items for Systematic Reviews and Meta-Analyses) flow diagram of the identified publications.
PRISMA checklist.
- Content of premarket submissions for device software functions: guidance for industry and Food and Drug Administration staff. U.S. Food and Drug Administration. Jun 14, 2023. URL: https://www.fda.gov/media/153781/download [accessed 2024-07-19]
- Patel NA, Butte AJ. Characteristics and challenges of the clinical pipeline of digital therapeutics. NPJ Digit Med. Dec 11, 2020;3(1):159. [ FREE Full text ] [ CrossRef ] [ Medline ]
- Policy for device software functions and mobile medical applications: guidance for industry and Food and Drug Administration staff. U.S. Food and Drug Administration. URL: https://www.fda.gov/media/80958/download [accessed 2023-01-06]
- Melcher J, Torous J. Smartphone apps for college mental health: a concern for privacy and quality of current offerings. Psychiatr Serv. Nov 01, 2020;71(11):1114-1119. [ CrossRef ] [ Medline ]
- Hoffman L, Benedetto E, Huang H, Grossman E, Kaluma D, Mann Z, et al. Augmenting mental health in primary care: a 1-year study of deploying smartphone apps in a multi-site primary care/behavioral health integration program. Front Psychiatry. 2019;10:94. [ FREE Full text ] [ CrossRef ] [ Medline ]
- Tong A, Flemming K, McInnes E, Oliver S, Craig J. Enhancing transparency in reporting the synthesis of qualitative research: ENTREQ. BMC Med Res Methodol. Nov 27, 2012;12(1):181. [ FREE Full text ] [ CrossRef ] [ Medline ]
- Moher D, Liberati A, Tetzlaff J, Altman DG, PRISMA Group. Preferred reporting items for systematic reviews and meta-analyses: the PRISMA statement. BMJ. Jul 21, 2009;339(jul21 1):b2535. [ FREE Full text ] [ CrossRef ] [ Medline ]
- CASP critical appraisal checklists. Critical Appraisal Skills Programme. URL: https://casp-uk.net/casp-tools-checklists/ [accessed 2022-01-06]
- Carroll C, Booth A, Cooper K. A worked example of "best fit" framework synthesis: a systematic review of views concerning the taking of some potential chemopreventive agents. BMC Med Res Methodol. Mar 16, 2011;11(1):29. [ FREE Full text ] [ CrossRef ] [ Medline ]
- Carroll C, Booth A, Leaviss J, Rick J. "Best fit" framework synthesis: refining the method. BMC Med Res Methodol. Mar 13, 2013;13(1):37. [ FREE Full text ] [ CrossRef ] [ Medline ]
- Kolasa K, Kozinski G. How to value digital health interventions? a systematic literature review. Int J Environ Res Public Health. Mar 23, 2020;17(6):2119. [ FREE Full text ] [ CrossRef ] [ Medline ]
- Lagan S, Aquino P, Emerson MR, Fortuna K, Walker R, Torous J. Actionable health app evaluation: translating expert frameworks into objective metrics. NPJ Digit Med. 2020;3:100. [ FREE Full text ] [ CrossRef ] [ Medline ]
- Lagan S, Emerson MR, King D, Matwin S, Chan SR, Proctor S, et al. Mental health app evaluation: updating the American Psychiatric Association's framework through a stakeholder-engaged workshop. Psychiatr Serv. Sep 01, 2021;72(9):1095-1098. [ CrossRef ] [ Medline ]
- Hong QN, Pluye P, Bujold M, Wassef M. Convergent and sequential synthesis designs: implications for conducting and reporting systematic reviews of qualitative and quantitative evidence. Syst Rev. Mar 23, 2017;6(1):61. [ FREE Full text ] [ CrossRef ] [ Medline ]
- Beel J, Gipp B, Langer S, Genzmehr M. Docear: an academic literature suite for searching, organizing and creating academic literature. In: Proceedings of the 11th annual international ACM/IEEE joint conference on Digital libraries. 2011. Presented at: JCDL '11; June 13-17, 2011:565-566; Ottawa, ON. URL: https://dl.acm.org/doi/10.1145/1998076.1998188 [ CrossRef ]
- Forma F, Chiu K, Shafrin J, Boskovic DH, Veeranki SP. Are caregivers ready for digital? caregiver preferences for health technology tools to monitor medication adherence among patients with serious mental illness. Digit Health. 2022;8:20552076221084472. [ FREE Full text ] [ CrossRef ] [ Medline ]
- Afra P, Bruggers CS, Sweney M, Fagatele L, Alavi F, Greenwald M, et al. Mobile software as a medical device (SaMD) for the treatment of epilepsy: development of digital therapeutics comprising behavioral and music-based interventions for neurological disorders. Front Hum Neurosci. 2018;12:171. [ FREE Full text ] [ CrossRef ] [ Medline ]
- Beard C, Silverman AL, Forgeard M, Wilmer MT, Torous J, Björgvinsson T. Smartphone, social media, and mental health app use in an acute transdiagnostic psychiatric sample. JMIR Mhealth Uhealth. Jun 07, 2019;7(6):e13364. [ FREE Full text ] [ CrossRef ] [ Medline ]
- Borghouts J, Neary M, Palomares K, de Leon C, Schueller SM, Schneider M, et al. Understanding the potential of mental health apps to address mental health needs of the deaf and hard of hearing community: mixed methods study. JMIR Hum Factors. Apr 11, 2022;9(2):e35641. [ FREE Full text ] [ CrossRef ] [ Medline ]
- Boster JB, McCarthy JW. Designing augmentative and alternative communication applications: the results of focus groups with speech-language pathologists and parents of children with autism spectrum disorder. Disabil Rehabil Assist Technol. May 10, 2018;13(4):353-365. [ CrossRef ] [ Medline ]
- Buck B, Chander A, Monroe-DeVita M, Cheng SC, Stiles B, Ben-Zeev D. Mobile health for caregivers of young adults with early psychosis: a survey study examining user preferences. Psychiatr Serv. Aug 01, 2021;72(8):955-959. [ FREE Full text ] [ CrossRef ] [ Medline ]
- Buck B, Chander A, Tauscher J, Nguyen T, Monroe-DeVita M, Ben-Zeev D. mHealth for young adults with early psychosis: user preferences and their relationship to attitudes about treatment-seeking. J Technol Behav Sci. 2021;6(4):667-676. [ CrossRef ] [ Medline ]
- Carpenter-Song E, Noel VA, Acquilano SC, Drake RE. Real-world technology use among people with mental illnesses: qualitative study. JMIR Ment Health. Nov 23, 2018;5(4):e10652. [ FREE Full text ] [ CrossRef ] [ Medline ]
- Casarez RL, Barlow E, Iyengar SM, Soares JC, Meyer TD. Understanding the role of m-health to improve well-being in spouses of patients with bipolar disorder. J Affect Disord. May 01, 2019;250:391-396. [ CrossRef ] [ Medline ]
- Connolly SL, Miller CJ, Koenig CJ, Zamora KA, Wright PB, Stanley RL, et al. Veterans' attitudes toward smartphone app use for mental health care: qualitative study of rurality and age differences. JMIR Mhealth Uhealth. Aug 22, 2018;6(8):e10748. [ FREE Full text ] [ CrossRef ] [ Medline ]
- Cummings JR, Gaydos LM, Mensa-Kwao A, Song M, Blake SC. Perspectives on caregiver-focused mHealth technologies to improve mental health treatment for low-income youth with ADHD. J Technol Behav Sci. Mar 9, 2019;4(1):6-16. [ FREE Full text ] [ CrossRef ] [ Medline ]
- Dinkel D, Harsh Caspari J, Fok L, Notice M, Johnson DJ, Watanabe-Galloway S, et al. A qualitative exploration of the feasibility of incorporating depression apps into integrated primary care clinics. Transl Behav Med. Sep 15, 2021;11(9):1708-1716. [ CrossRef ] [ Medline ]
- Huberty J, Bhuiyan N, Neher T, Joeman L, Mesa R, Larkey L. Leveraging a consumer-based product to develop a cancer-specific mobile meditation app: prototype development study. JMIR Form Res. Jan 14, 2022;6(1):e32458. [ FREE Full text ] [ CrossRef ] [ Medline ]
- Kern A, Hong V, Song J, Lipson SK, Eisenberg D. Mental health apps in a college setting: openness, usage, and attitudes. Mhealth. Jun 2018;4:20. [ FREE Full text ] [ CrossRef ] [ Medline ]
- Knapp AA, Cohen K, Nicholas J, Mohr DC, Carlo AD, Skerl JJ, et al. Integration of digital tools into community mental health care settings that serve young people: focus group study. JMIR Ment Health. Aug 19, 2021;8(8):e27379. [ FREE Full text ] [ CrossRef ] [ Medline ]
- Kornfield R, Meyerhoff J, Studd H, Bhattacharjee A, Williams JJ, Reddy MC, et al. Meeting users where they are: user-centered design of an automated text messaging tool to support the mental health of young adults. In: Proceedings of the 2022 CHI Conference on Human Factors in Computing Systems. 2022. Presented at: CHI '22; April 29-May 5 2022:1-6; New Orleans, LA. URL: https://dl.acm.org/doi/abs/10.1145/3491102.3502046
- Lipschitz J, Miller CJ, Hogan TP, Burdick KE, Lippin-Foster R, Simon SR, et al. Adoption of mobile apps for depression and anxiety: cross-sectional survey study on patient interest and barriers to engagement. JMIR Ment Health. Jan 25, 2019;6(1):e11334. [ FREE Full text ] [ CrossRef ] [ Medline ]
- Mata-Greve F, Johnson M, Pullmann MD, Friedman EC, Griffith Fillipo I, Comtois KA, et al. Mental health and the perceived usability of digital mental health tools among essential workers and people unemployed due to COVID-19: cross-sectional survey study. JMIR Ment Health. Aug 05, 2021;8(8):e28360. [ FREE Full text ] [ CrossRef ] [ Medline ]
- Melcher J, Camacho E, Lagan S, Torous J. College student engagement with mental health apps: analysis of barriers to sustained use. J Am Coll Health. Oct 13, 2022;70(6):1819-1825. [ CrossRef ] [ Medline ]
- Schueller SM, Neary M, O'Loughlin K, Adkins EC. Discovery of and interest in health apps among those with mental health needs: survey and focus group study. J Med Internet Res. Jun 11, 2018;20(6):e10141. [ FREE Full text ] [ CrossRef ] [ Medline ]
- Schueller SM, Neary M, Lai J, Epstein DA. Understanding people's use of and perspectives on mood-tracking apps: interview study. JMIR Ment Health. Aug 11, 2021;8(8):e29368. [ FREE Full text ] [ CrossRef ] [ Medline ]
- Stiles-Shields C, Montague E, Lattie EG, Kwasny MJ, Mohr DC. What might get in the way: barriers to the use of apps for depression. Digit Health. Jun 08, 2017;3:2055207617713827. [ FREE Full text ] [ CrossRef ] [ Medline ]
- Storm M, Venegas M, Gocinski A, Myers A, Brooks J, Fortuna KL. Stakeholders' perspectives on partnering to inform the software development lifecycle of smartphone applications for people with serious mental illness: enhancing the software development lifecycle through stakeholder engagement. In: Proceedings of the 2021 IEEE Global Humanitarian Technology Conference. 2021. Presented at: GHTC '21; October 19-23, 2021:195-199; Seattle, WA. URL: https://ieeexplore.ieee.org/document/9612444 [ CrossRef ]
- Torous J, Wisniewski H, Liu G, Keshavan M. Mental health mobile phone app usage, concerns, and benefits among psychiatric outpatients: comparative survey study. JMIR Ment Health. Nov 16, 2018;5(4):e11715. [ FREE Full text ] [ CrossRef ] [ Medline ]
- Zhou L, Parmanto B. User preferences for privacy protection methods in mobile health apps: a mixed-methods study. Int J Telerehabil. Dec 08, 2020;12(2):13-26. [ FREE Full text ] [ CrossRef ] [ Medline ]
- Baumel A, Muench F, Edan S, Kane JM. Objective user engagement with mental health apps: systematic search and panel-based usage analysis. J Med Internet Res. Sep 25, 2019;21(9):e14567. [ FREE Full text ] [ CrossRef ] [ Medline ]
- Gan DZ, McGillivray L, Han J, Christensen H, Torok M. Effect of engagement with digital interventions on mental health outcomes: a systematic review and meta-analysis. Front Digit Health. 2021;3:764079. [ FREE Full text ] [ CrossRef ] [ Medline ]
- Mobile fact sheet. Pew Research Center. 2021. URL: https://www.pewresearch.org/internet/fact-sheet/mobile/ [accessed 2024-04-29]
- Roberts ET, Mehrotra A. Assessment of disparities in digital access among Medicare beneficiaries and implications for telemedicine. JAMA Intern Med. Oct 01, 2020;180(10):1386-1389. [ FREE Full text ] [ CrossRef ] [ Medline ]
- Powell AC, Torous JB, Firth J, Kaufman KR. Generating value with mental health apps. BJPsych Open. Feb 05, 2020;6(2):e16. [ FREE Full text ] [ CrossRef ] [ Medline ]
- O'Loughlin K, Neary M, Adkins EC, Schueller SM. Reviewing the data security and privacy policies of mobile apps for depression. Internet Interv. Mar 2019;15:110-115. [ FREE Full text ] [ CrossRef ] [ Medline ]
- Akbar S, Coiera E, Magrabi F. Safety concerns with consumer-facing mobile health applications and their consequences: a scoping review. J Am Med Inform Assoc. Feb 01, 2020;27(2):330-340. [ FREE Full text ] [ CrossRef ] [ Medline ]
- Curran GM, Bauer M, Mittman B, Pyne JM, Stetler C. Effectiveness-implementation hybrid designs: combining elements of clinical effectiveness and implementation research to enhance public health impact. Med Care. Mar 2012;50(3):217-226. [ FREE Full text ] [ CrossRef ] [ Medline ]
- Systematic reviews: CRD's guidance for undertaking reviews in health care. Centre for Reviews and Dissemination. 2008. URL: https://www.york.ac.uk/media/crd/Systematic_Reviews.pdf [accessed 2024-04-29]
- Gumas ED, Lewis C, Horstman C, Gunja MZ. Finger on the pulse: the state of primary care in the U.S. and nine other countries. The Commonwealth Fund. URL: https://www.commonwealthfund.org/publications/issue-briefs/2024/mar/finger-on-pulse-primary-care-us-nine-countries [accessed 2024-04-29]
- Huang EC, Pu C, Chou YJ, Huang N. Public trust in physicians-health care commodification as a possible deteriorating factor: cross-sectional analysis of 23 countries. Inquiry. Mar 05, 2018;55:46958018759174. [ FREE Full text ] [ CrossRef ] [ Medline ]
Abbreviations
attention-deficit/hyperactivity disorder |
American Psychiatric Association |
autism spectrum disorder |
bipolar disorder |
digital mental health technology |
Food and Drug Administration |
health care provider |
major depressive disorder |
mental health |
personal health information |
Preferred Reporting Items for Systematic Reviews and Meta-Analyses |
systematic literature review |
Sample, Phenomenon of Interest, Design, Evaluation, Research type |
Edited by J Torous; submitted 15.02.24; peer-reviewed by A Mathieu-Fritz, K Stawarz; comments to author 05.05.24; revised version received 20.06.24; accepted 21.06.24; published 30.08.24.
©Julianna Catania, Steph Beaver, Rakshitha S Kamath, Emma Worthington, Minyi Lu, Hema Gandhi, Heidi C Waters, Daniel C Malone. Originally published in JMIR Mental Health (https://mental.jmir.org), 30.08.2024.
This is an open-access article distributed under the terms of the Creative Commons Attribution License (https://creativecommons.org/licenses/by/4.0/), which permits unrestricted use, distribution, and reproduction in any medium, provided the original work, first published in JMIR Mental Health, is properly cited. The complete bibliographic information, a link to the original publication on https://mental.jmir.org/, as well as this copyright and license information must be included.
How educational chatbots support self-regulated learning? A systematic review of the literature
- Open access
- Published: 30 August 2024
Cite this article
You have full access to this open access article
- Rui Guan ORCID: orcid.org/0009-0006-4789-3741 1 ,
- Mladen Raković ORCID: orcid.org/0000-0002-1413-1103 1 ,
- Guanliang Chen ORCID: orcid.org/0000-0002-8236-3133 1 &
- Dragan Gašević ORCID: orcid.org/0000-0001-9265-1908 1 , 2
Engagement in self-regulated learning (SRL) may improve academic achievements and support development of lifelong learning skills. Despite its educational potential, many students find SRL challenging. Educational chatbots have a potential to scaffold or externally regulate SRL processes by interacting with students in an adaptive way. However, to our knowledge, researchers have yet to learn whether and how educational chatbots developed so far have (1) promoted learning processes pertaining to SRL and (2) improved student learning performance in different tasks. To contribute this new knowledge to the field, we conducted a systematic literature review of the studies on educational chatbots that can be linked to processes of SRL. In doing so, we followed the PRISMA guidelines. We collected and reviewed publications published between 2012 and 2023, and identified 27 publications for analysis. We found that educational chatbots so far have mainly supported learners to identify learning resources, enact appropriate learning strategies, and metacognitively monitor their studying. Limited guidance has been provided to students to set learning goals, create learning plans, reflect on their prior studying, and adapt to their future studying. Most of the chatbots in the reviewed corpus of studies appeared to promote productive SRL processes and boost learning performance of students across different domains, confirming the potential of this technology to support SRL. However, in some studies the chatbot interventions showed non-significant and mixed effects. In this paper, we also discuss the findings and provide recommendations for future research.
Similar content being viewed by others
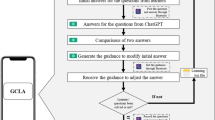
Empowering ChatGPT with guidance mechanism in blended learning: effect of self-regulated learning, higher-order thinking skills, and knowledge construction
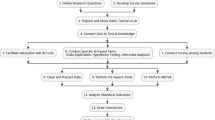

The role and impact of ChatGPT in educational practices: insights from an Australian higher education case study
Envisioned Pedagogical Uses of Chatbots in Higher Education and Perceived Benefits and Challenges
Explore related subjects.
- Artificial Intelligence
- Digital Education and Educational Technology
Avoid common mistakes on your manuscript.
1 Introduction
Self-regulated learning (SRL) is considered a complex set of recursive and goal-oriented learning processes (Panadero, 2017 ). Self-regulated learners set their learning goals and actively select, monitor and modify their learning strategies to accomplish these goals and succeed in different learning tasks (Zimmerman, 2013 ; Winne & Hadwin, 1998 ; Winne, 2022 ; Cleary et al., 2022 ). Self-regulated learners are thus in control over their learning processes and learning goals (Winne, 2018 ). As engagement in SRL processes has a potential to improve academic achievements and, more broadly, to support lifelong learning (Cleary & Chen, 2009 ; Klug et al., 2011 ; Recommendation, 2018 ; Theobald, 2021 ), it is critical for students to master their command of SRL and become productive learners in different domains of knowledge.
To advance understanding of SRL and identify the relationships among different learning processes involved, researchers have proposed several SRL theoretical frameworks, such as Winne and Hadwin ( 1998 ); Winne ( 2018 ); Zimmerman ( 2000 ); Pintrich ( 2000 ). Although differences among these theoretical models are noticeable, these models broadly agree that SRL is a cyclic process that involves a repertoire of learning goals and learning strategies (Panadero, 2017 ). For example, according to Zimmerman ( 2000 ), self-regulated learners selectively use specific processes to work on learning tasks, over three cyclical phases: forethought, performance and self-refection. Winne and Hadwin ( 1998 )’s theoretical model describes SRL as a dynamic set of skills where learning unfolds over five facets (conditions, operations, products, evaluations, and standards - COPES) and four phases (defining task requirements, setting goals and devising plans, enacting study tactics, and adapting future studying).
Even though researchers have made a substantial progress over the past several decades towards deeper understanding and more effective support for learning processes involved in SRL, development of SRL skills is still considered challenging for many students (Bjork et al., 2013 ). For example, students struggle to gather appropriate resources for a learning task (List & Du, 2021 ); set relevant, specific and attainable goals to guide their engagement with the task(McCardle et al., 2017 ); select appropriate learning strategies and effectively use them (Azevedo, 2018 ; List & Lin, 2023 ); and accurately monitor and evaluate their own progress (Zimmerman, 2002 ; Gutierrez de Blume, 2022 ; Lim et al., 2023 ). Students often need guidance to successfully enact these learning processes. Educational researchers and practitioners proposed different types of external support to students as they are developing SRL skills (Jivet et al., 2020 , 2021 ; Perez-Alvarez et al., 2022 ). Broadly, the SRL support has so far been provided in a more traditional way, e.g., via a classroom-style coaching on goal setting (McCardle et al., 2017 ; Morisano et al., 2010 ; Alessandri et al., 2020 ) and metacognitive strategies (Cleary et al., 2022 ; Dignath & Veenman, 2021 ), and, more recently, using technology-enhanced learning platforms, e.g., computer-based scaffolding environments that support task orientation, strategy use and metacognitive monitoring (Baker et al., 2020 ; Azevedo et al., 2017 ; Azevedo & Aleven, 2013 ; Pérez et al., 2020 ; Jivet et al., 2020 , 2021 ; Dever et al., 2023 ; Srivastava et al., 2022 ; Lim et al., 2023 ).
In recent years, researchers have become increasingly interested in using chatbots to address educational problems (Wollny et al., 2021 ; Li et al., 2023 ; Dai et al., 2023 ). One of the main reasons for such increased interest is that chatbots have a potential to scaffold or externally regulate learning processes in dynamically changing learning contexts like SRL (Azevedo & Hadwin, 2005 ), because chatbots use artificial intelligence and natural language processing to simulate and adapt to conversation with humans. Following the growing interest in educational chatbots, researchers have recently published several literature reviews on the topic (Winkler & Söllner, 2018 ; Pérez et al., 2020 ; Smutny & Schreiberova, 2020 ). All these reviews have contributed a significant knowledge to this field, providing valuable findings about the currently available educational chatbots across disciplines and the benefits of using chatbot technologies in education to, e.g., supplement teaching or recommend learning content to students. However, to our knowledge, researchers have yet to learn how educational chatbots developed so far have supported processes theorised in SRL. These new findings may add to the current educational research and practice given the documented benefits of SRL skills for academic performance and life-long learning. To contribute new research knowledge to the fields of educational technology and learning sciences, we conducted the present systematic review of the literature explicitly focusing on how educational chatbots have been used to support SRL processes and learning achievements. Our analysis was based on Winne and Hadwin ( 1998 )’s theoretical framework that defined facets and phases of SRL. Our findings may inform future research related to development and implementation of educational chatbots that provide a more comprehensive SRL support to learners.
2 Background
2.1 srl theoretical framework to guide this systematic review.
Different theoretical frameworks have been proposed to date to define SRL processes and to understand the relationships among them, and, in this way, help researchers to measure and support learners’ engagement in SRL. For an overview of major SRL theoretical frameworks, see Panadero ( 2017 ). To theoretically ground our systematic literature review, we utilized the SRL theoretical model proposed by Winne and Hadwin ( 1998 ). According to this framework, students’ SRL processes unfold over four general phases: task definition, goal setting and planning, enacting study tactics, and adaptation to future studying, and five facets: conditions, operations, products, evaluations and standards. We opted to use this framework because (1) it is one of the six most cited frameworks in the literature, signifying its robustness and widespread acceptance among researchers, and it is particularly welcomed in research involving computer assisted learning (Panadero et al., 2016 ; 2) it provides a comprehensive account of cognitive, metacognitive and motivational processes that interweave in SRL offering a holistic view of the learning process; and (3) the model is distinguished by its detailed depiction of how different phases interact with each other over time as learning unfolds, affording researchers and educators ways to design specific and time-sensitive SRL support to learners (Greene & Azevedo, 2007 ).
The first phase in Winne and Hadwin’s model of SRL is task definition where learners make inferences and develop perceptions about the features of the task, and survey available resources for studying. The next phase is goal setting and planning where learners set their learning goals, devise plans and determine learning strategies which will be used to accomplish goals for learning. In the following phase, students enact their learning strategies and oversee (i.e., metacognitively monitor) the effectiveness of those strategies in addressing the task. For example, learners might highlight key concepts and construct a vocabulary list during a reading task, and, if they deem this strategy to be ineffective, they may decide to modify (i.e., metacognitively control) it, e.g., engage in note-taking instead of highlighting. In the adaptation phase, learners reflect on their studying during the previous stages and make forward-reaching adaptations for similar tasks in the future, e.g., a learner may decide to include note-taking in a repository of preferable learning strategies for the upcoming reading comprehension tasks, as note-taking worked well for the learner in the present task. In this way, learners reach beyond the present task and change their cognitive conditions for future learning (Greene & Azevedo, 2007 ).
Learning activities that unfold over the four general phases of SRL can be characterised relative to five common dimensions, i.e., facets: conditions, operations, products, evaluations and standards (COPES). Conditions encompass different internal and external factors that affect how a learner will engage with a task. For example, internal conditions include the learner’s prior knowledge of a domain, knowledge of learning strategies, experience with a task, and motivation and interest in a task; whereas external conditions include available learning resources, task instructions, scoring rubrics and time constraints. Operations are the processes by which learners manipulate information at hand and, in that way, induce actual learning (Winne, 2022 ). Winne ( 2018 ) defined five fundamental operations including searching, monitoring, assembling, rehearsing and translating (SMART). As learners engage in operations, they create products of learning, e.g., a note, essay draft or program code. Self-regulated learners actively evaluate their learning products against standards , e.g., a scoring rubric or instructional objectives. Upon evaluating their learning products, self-regulated learners may engage in metacognitive control, i.e., they may decide to modify their learning goals and strategies, and revise the products (Greene & Azevedo, 2007 ; Raković et al., 2022a ).
2.2 Educational chatbots
A chatbot is an interactive computer program enhanced by artificial intelligence (AI) and natural language processing (NLP) to simulate conversation with humans through text and voice. Since the development of the earliest chatbot Eliza (Weizenbaum, 1966 ) in 1966, various chatbots have evolved providing interactive interface for users to engage with different services, resources, and data in a natural conversational style (McTear, 2020 ). As well, chatbots have been used as tools to understand and model human behavior (McTear, 2020 ). The use of chatbots has seen a significant increase over the past several years (Zawacki-Richter et al., 2019 ), offering support to users in different contexts, e.g., customer services, online shopping and banking (Illescas-Manzano et al., 2021 ).
Due to its characteristics to dynamically and adaptively interact with users, educational chatbots have been considered a viable option to support learning in different settings (Smutny & Schreiberova, 2020 ), including SRL. For example, as metacognitive processes of monitoring and control are considered central in SRL (Winne, 2022 ), learners need to continuously engage those processes to succeed in a learning task. Many learners, however, struggle to sustain these metacognitive processes throughout a learning session (Azevedo & Aleven, 2013 ), which further prevents them from productively engaging in SRL and performing well in a task. Educational chatbot may provide external regulation to learners by performing a part of metacognitive monitoring instead of students having to conduct these processes by themselves (Molenaar, 2022 ), e.g, a bot may identify two learning strategies that a learner had used previously in the task and ask a learner to compare the effectiveness of these two strategies relative to task requirements. In this way, a chatbot may help the learner preserve cognitive resources for other aspects of the task, e.g., constructing deeper understanding of concepts studied. As well, by providing SRL guidance to students, chatbot may help learners increase their engagement across phases and facets of SRL, which may further benefit their development of SRL skills and boost their academic achievements.
Recent literature reviews (Winkler & Söllner, 2018 ; Pérez et al., 2020 ; Smutny & Schreiberova, 2020 ; Wollny et al., 2021 ) have reported that chatbots have been used for the two main purposes in educational settings, including (1) service support and (2) teaching support. Building on the success of chatbots in the area of customer service, chatbots have been used at many educational institutions to provide service support to students, e.g., support with enrolment, library and campus resources (Sweidan et al., 2021 ; Allison, 2012 ). For example, an interactive bot SIAAA-C (Sweidan et al., 2021 ) is designed to provide students with important campus resources, e.g., campus map and notifications during COVID-19. On the other hand, teaching-oriented chatbots have been commonly used in formal education to supplement traditional teaching in different domains, e.g., languages, math and science. Harnessing their conversational features, those chatbots typically play the role of human tutor and provide learners with content knowledge and practice questions. For instance, Wu et al. ( 2020 ) developed a multi-module chatbot that supported students studying mathematics and Chinese history, whereas Mageira et al. ( 2022 ) and Vázquez-Cano et al. ( 2021 ) created the chatbots to help students learn English and Spanish, respectively, e.g., through prompting and recommending additional learning resources. The literature reviews published so far (Winkler & Söllner, 2018 ; Pérez et al., 2020 ; Smutny & Schreiberova, 2020 ; Wollny et al., 2021 ) identifed different types of educational chatbots and technologies used to implement those bots. These reviews have also revealed the potential of using chatbots to facilitate teaching and learning processes, to recommend learning content and to provide service support to students. While there have been several studies investigating the use of chatbots for SRL, there has been insufficient understanding about the extent to which different aspects of SRL have been supported by chatbots. To address this gap, we conducted a systematic literature review to learn (1) how educational chatbots have provided support for learners’ SRL and (2) how that support has affected learners’ SRL skills and performance. This inquiry is critical because by identifying and synthesizing the ways in which educational chatbots contribute to or hinder SRL, our study could potentially offer valuable insights into the design of more effective educational technologies that are aligned with pedagogical goals. Second, understanding the impact of chatbots on learners’ SRL skills and performance can inform educators and policymakers about the potential benefits and limitations of integrating these technologies into the learning environments. More formally, the following Research Questions guided our systematic review:
RQ1: How have educational chatbots been used to support students’ SRL processes relative to (i) phases and (ii) facets theorised in Winne and Hadwin ( 1998 ) and Winne ( 2018 )?
RQ2: To what extent has the use of educational chatbots improved learners’ SRL processing and learning performance?
3 Methodology
We conducted a systematic review of the literature to answer our research questions. To ensure a thorough and transparent systematic literature review process, we carried this review using the Preferred Reporting Items for Systematic Reviews and Meta-Analysis (PRISMA) framework as a guideline (Page et al., 2021 ; Moher et al., 2009 ). The systematic literature review involved three major phases (1) search for relevant publications in multiple bibliographical databases, (2) select relevant publications following the PRISMA framework, (3) extract and analyse relevant information in selected publications to answer research questions.
3.1 Literature search
We utilized the SPIDER framework (Cooke et al., 2012 ) to define parameters for the literature search. The SPIDER framework proposes five general groups of search criteria, including sample, phenomenon of interest, design, evaluation and research type. As per our inclusion criteria (detailed in the next section) our Sample (S) involved students studying in formal educational settings at primary, secondary and tertiary levels. The Phenomena of our Interest (PI) were self-regulated learning and educational chatbots. We searched for research studies that have been Designed to empirically evaluate the effects of chatbots on SRL (D) and that have reported outcome measures based on these Evaluations (E). We included qualitative, quantitative and mixed-methods studies (R) in our search.
We used the following search query: (“chatbot” OR “educational chatbot” OR “conversational agent”) AND “self-regulated learning” AND “formal education” AND (“student” OR “learner”) AND “research article” to search for titles, abstracts and keywords of publications in bibliographical databases. We included studies published between 2012 and 2023, inclusively, as we deemed this time range to be sufficient to capture the state-of-the-art in the emerging field of educational chatbots. We searched the following bibliographical databases: Scopus, Elsevier, ACM, IEEE Xplore, Web of Science, ERIC, PsychInfo, Wiley library, Google Scholar, ResearchGate and the library database at our university. The search was conducted in October 2023. At this stage, we retrieved 598 publications. After removing 72 duplicates, 526 publications remained in our dataset for further analysis.
3.2 Abstract screening and full paper review
To identify relevant publications for our review we performed two reviewing steps, following the PRISMA guidelines (1) abstract screening and (2) full paper review. In other words, publications selected in the abstract screening step were reviewed in full for their relevance at the full paper review step. For these two reviewing steps, we followed our inclusion and exclusion criteria. Specifically, we included research studies that:
Reported on the use of chatbots in formal educational settings
Reported on the use of chatbots to support students to engage in SRL processing (e.g., goal setting, strategy use, and monitoring)
Described characteristics of educational chatbots (e.g., chatbot architecture and types of utterances exchanged between student and bot)
Reported on the effectiveness of educational chatbots in supporting SRL skills and/or learning outcomes
Were published in peer-reviewed journals and conference proceedings in English between Jan 2012 and Oct 2023
We excluded:
Publications that reported on using chatbots outside of formal educational settings (e.g., school administration and customer service)
Publications from which it could not be clearly inferred what SRL processes have been supported by the chatbot (e.g., studies applying a third-party chatbot as a black box intervention or using a chatbot to conduct a quiz)
Publications that did not provide a clear description of chatbot characteristics
Publications that did not provide the evaluation of chatbot effectiveness
Technical reports, conceptual and design papers
Non-peer reviewed publications and publications without available full-text
At the screening step, two reviewers screened the titles and abstracts of 526 publications, i.e., those publications that remained from the previous phase in this review. Each reviewer had an opportunity to vote “Yes”, “Maybe” or “No” for the study, relative to whether the study should be included in the next stage of the review. The reviewers had the agreement on 456 papers ( 86.7%, Fleiss kappa = 0.734, p <0.001). The remaining 70 conflicts were resolved through discussion between the reviewers. The main reasons for conflicts came from abstracts that did not explicitly state whether the chatbot evaluation was performed in the study. The reviewers agreed to keep such articles in the dataset and fully assess those in the next stage. A total of 101 publications remained in the dataset after this stage.
At the full paper review step, the reviewers randomly selected 15 out of 101 publications (nearly 15%), separately reviewed those and voted whether the paper should be included in the study or not, following the inclusion and exclusion criteria. The reviewers agreed on 12 out of 15 publications (80%, Fleiss kappa = 0.52, p =0.04). The common disagreement between the reviewers at this stage was about whether the study provided a sufficiently clear description of the bot characteristics. This disagreement was resolved through discussion between the reviewers and the decision was made to include in the final review only those publications that described types of utterances exchanged between a student and a bot. The reviewers evenly split the remaining publications in the dataset (i.e., 86 publications were randomly assigned to each reviewer) and reviewed those separately. A total of 27 papers were extracted for the review. We summarized our review process in Fig. 1 (Page et al., 2021 ).
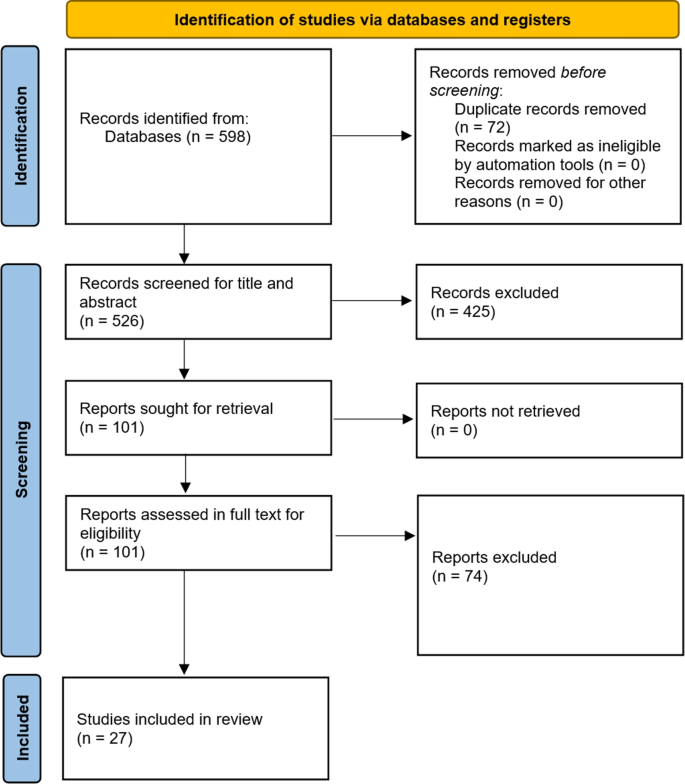
PRISMA flow diagram
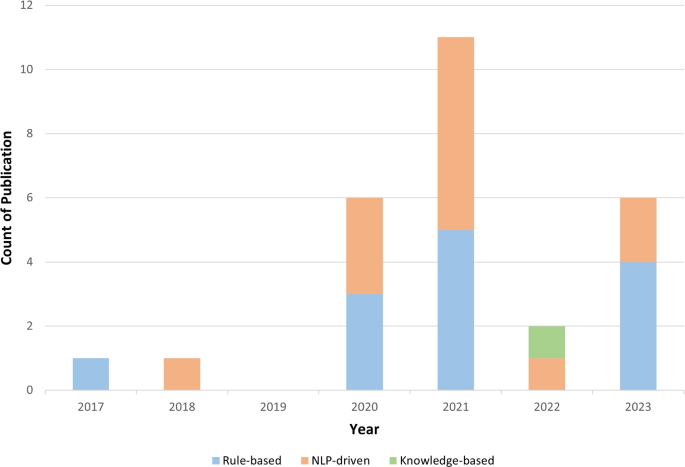
Number of publications by year color-coded with chatbot architectures
3.3 Analysis of extracted publications
The first author of this review extracted data from each publication as per following categories: general information (publication title, authors, year, sample size, level of education, domain of education and learning task), chatbot type, SRL facet (conditions, operations, products, evaluations, and standards), SRL phase (task understanding, goal setting and planning, enactment, and adaptation), and reported effects (on SRL processes and learning achievements). To categorise publications into suitable SRL facets and phases, the first author closely followed definitions of constructs provided in Winne and Hadwin ( 1998 ). See the section SRL Theoretical Framework to Guide This Review for details. The analysis of SRL facets and phases in selected publications was used to address RQ1, while the analysis of the reported effects of chatbots on SRL processes and learning achievements was used to address RQ2.
4.1 General information
We summarised the studies included in our systematic literature review in Fig. 2 . Out of the 526 studies that we assessed in this review, 27 studies fit the inclusion criteria for full review. Over 92% of these studies were published in 2020 onward, i.e, six in 2020, 11 in 2021, two studies in 2022 and six studies in 2023, whereas only two studies were published before 2020. We observed that 13 studies utilized a natural language processing (NLP)-driven approach in their chatbot design to interpret and respond to user inputs in a conversational manner. On the other hand, 13 studies employed rule-based architectures in their chatbot design, i.e., following predefined pathways or rules to respond to specific commands or keywords, offering predictable and consistent interactions within a structured framework (Fig. 2 ). Additional architectures in the reviewed studies include an NLP-driven architecture with contextual bandit algorithm (Cai et al., 2021 ) and knowledge-based system accessing a vast domain-specific database to deliver accurate information (Chang et al., 2022b ).
Further, the chatbots we reviewed provided SRL support to students in different domains of education, including language learning, math, science, computer programming, accounting and educational psychology, with language learning being slightly more prominent than the other domains (Fig. 3 ). Moreover, the chatbots included in this review have been mainly utilised in higher education, i.e., researchers provided chatbots to university students in 21 studies. Two studies were conducted in primary schools, three studies were conducted in secondary school and one study involved a diverse student population recruited from Amazon Mechanical Turk (Fig. 3 ).
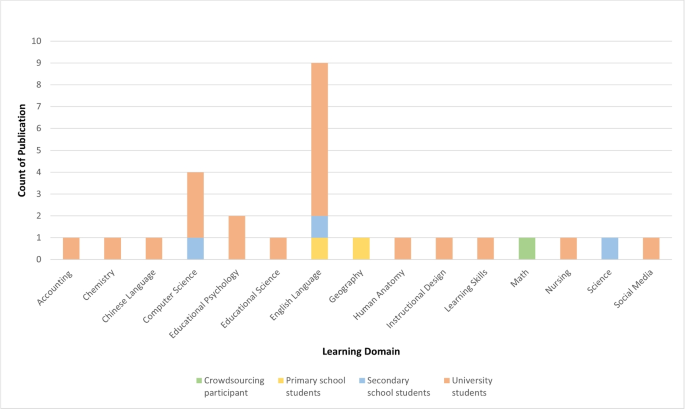
Domain of education supported by chatbot color-coded with participants’ level of education
4.2 RQ1: How have educational chatbots been used to support students’ SRL processes relative to (1) phases and (2) facets theorised in Winne and Hadwin ( 1998 ) and Winne ( 2018 )?
Of 27 articles included in this review, 15 reported on using chatbots to support student SRL processing in a single SRL phase, 11 articles reported on support across two and 1 article reported on support across three SRL phases. None of the reviewed studies appeared to utilise educational chatbots to provide comprehensive SRL support across all four phases of SRL defined in Winne and Hadwin ( 1998 ). More specifically, in 25 articles researchers used chatbots to facilitate SRL during the strategy enactment phase, i.e., the phase in which students are to select and use learning tactics and strategies. In these studies, chatbots were mainly utilised to guide students to enact learning tactics/strategies to accomplish a particular learning task, such as writing a thesis statement (Lin & Chang, 2020 ) or an essay (Neumann et al., 2021 ), learning a programming language (Ait et al., 2023 ; Tian et al., 2021 ) and developing a project report (Kumar, 2021 ). Six chatbots supported students at the task definition stage, e.g., “Make sure to re-read the question!” (Cai et al., 2021 ). Five chatbots supported students to set goals and devise plans for learning, e.g., by scaffolding students to specify their achievement goals (Hew et al., 2021 , 2023 ) and by guiding goal setting with questions (Du et al., 2021 ; Al-Abdullatif et al., 2023 ). Four chatbots supported students to adapt to their future studying, e.g., by providing students with the opportunity to monitor their learning progress (Harati et al., 2021 ; Oliveira et al., 2021 ) (Fig. 4 ).
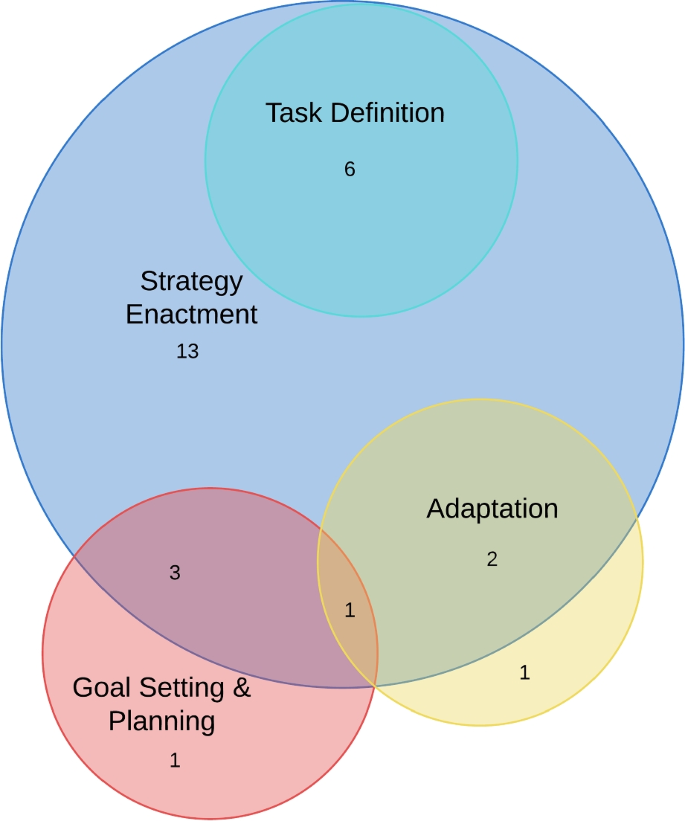
Venn diagram showing the number of studies over SRL phases
In all the studies we reviewed authors have reported on using chatbots to promote SRL processes at conditions, operations, and products, the three cognitive facets of SRL. For instance, researchers have used chatbots to promote students’ internal conditions for a task that include activation of domain knowledge (Cai et al., 2021 ; Neumann et al., 2021 ), task interest and motivation (Fryer et al., 2017 , 2020 ; Yin et al., 2021 ), self-efficacy (Chang et al., 2022a ), and outcome expectation (Hew et al., 2021 )). Researchers have also utilised chatbots to support students to leverage external conditions for a task. For example, chatbots recommended learning resources to students (Bailey et al., 2021 ; Chang et al., 2022b ), and guided students to manage their studying time (Harati et al., 2021 ) and to understand task requirements Du et al. ( 2021 ); Mellado-Silva et al. ( 2020 ); Chen et al. ( 2020 )). We also found that in 17 studies chatbots supported students to engage in cognitive operations of assembling. These include integrating and consolidating conceptual knowledge in math (Cai et al., 2021 ), English language learning (Fryer et al., 2017 ; Xia et al., 2023 ), physical sciences (Deveci Topal et al., 2021 ) and accounting (Mellado-Silva et al., 2020 ). Nine chatbots provided support for cognitive operations of translating. These include guiding students to transform knowledge from readings into a written narrative (Bailey et al., 2021 ) and to apply knowledge in a practical project (Kumar, 2021 ). 13 chatbots provided support for metacognitive operations of monitoring. These include guiding students to monitor for domain knowledge acquisition (Harati et al., 2021 ), for learning goals and responses to questions (Hew et al., 2021 ), for learning strategy employment (Song & Kim, 2021 ) and for progress and performance (Cai et al., 2021 ; Neumann et al., 2021 ; Oliveira et al., 2021 ; Zhang et al., 2023a , b ). Five chatbots provided support for searching operations. These include guiding students to search for course materials and learning content (Chang et al., 2022a , b ; Oliveira et al., 2021 ), for specific learning strategies (Du et al., 2021 ) and for learning tools (Jones & Castellano, 2018 ). And one chatbot included support for a cognitive operation of rehearsing by guiding students to formulate acquired knowledge in their own words (Jeon, 2021 ). Next, we found that the most common learning products that students created while studying with chatbots were answers to questions on tests/quizzes (Cai et al., 2021 ; Chang et al., 2022b ; Jeon, 2021 ), and only a few chatbots have supported students to produce essays (Neumann et al., 2021 ), thesis statements (Lin & Chang, 2020 ), project reports (Kumar, 2021 ) and learning goals (Du et al., 2021 ).
Further, 16 chatbots in the corpus we reviewed have appeared to provide support for learning processes theorised to occur at the evaluations facet of SRL. For instance, chatbots utilised in Cai et al. ( 2021 ), Oliveira et al. ( 2021 ), Zhang et al. ( 2023a ) and Lin and Chang ( 2020 ) assisted students to engage in judgment of learning, whereas chatbots in Jones and Castellano ( 2018 ), Hew et al. ( 2021 ), Song and Kim ( 2021 ) and Yin et al. ( 2021 ) promoted student engagement in self-reflection. Last, 14 chatbots provided a guidance to students to better comprehend task standards. Specifically, these chatbots provided students with initial explanations of task requirements and other task features (Bailey et al., 2021 ; Lin & Chang, 2020 ; Jones & Castellano, 2018 ; Chen et al., 2020 ), task-related tips (Tian et al., 2021 ), opportunities for progress check relative to task topics (Harati et al., 2021 ), and questions for goal setting (Du et al., 2021 ; Hew et al., 2023 ). We provide the summary table of the SRL phases and facets supported by the educational chatbots included in this review in the appendix (Figs. 5 , 6 , 7 , 8 , and 9 ).
4.3 RQ2: To what extent the use of educational chatbots improved students’ SRL processing and learning performance?
Among the publications reviewed, we found mixed effects of educational chatbots on students’ SRL processes and learning performance. In terms of promoting SRL processing, researchers have reported that students who studied with chatbots tended to: use more effective learning strategies (Bailey et al., 2021 ; Chang et al., 2022b ; Mellado-Silva et al., 2020 ), increase their awareness of the importance of setting learning goals (Du et al., 2021 ; Hew et al., 2023 ), control the learning process over their study pace (Yin et al., 2021 ; Tian et al., 2021 ), enhance their learning engagement and self-efficacy (Chang et al., 2022a ; Hew et al., 2021 ; Oliveira et al., 2021 ), and transfer some of their SRL skills to a new learning activity (Jones & Castellano, 2018 ). Researchers have also found that students who studied with a chatbot did not sustain well their interest in task, attributed to the novelty effect (Fryer et al., 2017 ), and did not increase their SRL processing (Harati et al., 2021 ). Moreover, the use of chatbot in one of the studies did not appear to statistically significantly boost student internal conditions, i.e., need for cognition, perception of learning, creativity, self-efficacy and motivational beliefs – conditions critical for productive SRL (Kumar, 2021 ). The systematic review also shows that chatbots were used to improve students’ learning performance in tasks spanning different subjects, including English as a second language (Bailey et al., 2021 ), obstetrics (Chang et al., 2022a ), physical education (Chang et al., 2022b ), science (Deveci Topal et al., 2021 ), accounting (Mellado-Silva et al., 2020 ), geography (Jones & Castellano, 2018 ) and educational psychology (Lin & Chang, 2020 ; Kumar, 2021 ). We also note that the use of chatbot had limited effects on learning performance of students working on a chemistry task (Harati et al., 2021 ), and statistically non-significant effects on performance of students working on tasks in math (Cai et al., 2021 ), computer science (Oliveira et al., 2021 ) and geography (Jones & Castellano, 2018 ). We summarised descriptive and inferential statistics on SRL processes and learning performance across the reviewed studies in the appendix (Figs. 5 , 6 , 7 , 8 , and 9 ).
5 Discussion
Even though chatbot is not a new technology, our results indicate that the application of chatbots for promoting SRL has only recently attracted attention from educational researchers and practitioners, i.e., over 90% of the papers in the reviewed corpus were published after 2020. Unlike some other educational technologies that have been widely researched as support for student SRL over the past decade – e.g., intelligent tutoring systems (Duffy & Azevedo, 2015 ; Dever et al., 2023 ; Taub et al., 2021 ) and computer-based scaffolding environments (Molenaar et al., 2012 ; Srivastava et al., 2022 ; Lim et al., 2023 ) – the use of chatbots to this purpose appears yet to be more deeply explored.
The two most prominent chatbot architectures in the reviewed corpus were NLP-driven and rule-based chatbots. NLP-driven chatbots utilize NLP and machine learning methods to derive the meaning from user input and understand user intents. Even though the NLP-driven models often require extensive training before they can be applied, chatbots based on this architecture typically offer more robustness in interpreting insufficiently clear and grammatically incorrect student inputs. We found DialogFlow to be a commonly used NLP platform powering NLP-driven chatbots for SRL (Deveci Topal et al., 2021 ; Bailey et al., 2021 ). On the other hand, rule-based chatbots use a set of predefined rules, e.g., a tree-like decision flow, to map student input to appropriate chatbot response. These rules are created after anticipating users’ input and pre-scripted during the bot design. Rule-based chatbot provides better behavior control and may be a particularly applicable architecture for researchers aiming to explicitly map user inputs to SRL processes, e.g., “What should I do first?” can be mapped to goal setting, and, based on that, chatbot may provide a series of prompts to guide a learner to set their goals. In this review, researchers utilised chatbots to support learning in a very diverse set of educational domains (i.e., 15 different domains identified in our corpus) and this finding aligns with findings from the previously conducted literature reviews (Winkler & Söllner, 2018 ; Pérez et al., 2020 ; Smutny & Schreiberova, 2020 ) that also reported that researchers tended to apply educational chatbots in diverse domains. The main reason for this cross-domain popularity of chatbots may be because this technology was designed to adapt to conversation with different users and on different topics.
Educational chatbots for self-regulated learning have mainly supported learners’ processes at one or two phases of the Winne and Hadwin model of SRL. Our findings suggest that the current design and implementation of educational chatbots lack the ability to aid the whole SRL cycle and thus provide students with comprehensive SRL support addressing all the four phases of SRL defined in Winne and Hadwin ( 1998 ) and Winne ( 2018 ). Commonly, almost all of the reviewed chatbots were designed to promote the enactment of learning tactics and strategies that educators deemed to be important for success in different learning tasks. For example, in the writing thesis statement task (Lin & Chang, 2020 ), educators may guide students to strategically engage the following learning activities “identify relevant passage” \(\rightarrow \) “identify claims” \(\rightarrow \) “compose thesis statement” \(\rightarrow \) “evaluate your conceptual understanding” \(\rightarrow \) “revise thesis statement”. The bot was designed to provide guidance to students on these activities, in any order they prefer. For this reason, utterances between learners and chatbots have been often mapped to specific learning tactics to reinforce the learning of students, taking into account required learning activities. In this way, chatbots have served as a potentially effective supplement to traditional classroom teaching, the trend also identified in the previous literature (Pérez et al., 2020 ). This finding ties with another finding from our review showing that chatbots mainly supported operations of rehearsing, assembling and translating, i.e., cognitive operations that are typically contingent upon task conditions (Winne, 1995 ), such as integrate information from several readings in an essay or recap a math formula in a quiz. Together, these findings may suggest that design of SRL chatbots was primarily informed by the nature of specific learning tasks, e.g., persuasive writing, numerical conversion and software programming, and, as such, dependent upon expected sequences of actions that learners should take to address those tasks.
To a lesser extent, chatbots supported students to metacognitively evaluate their immediate and past studying, and to adapt their studying accordingly. For instance, by using interactive and personalised feedback from chatbots the students were afforded the opportunity to engage in judgement of learning (Cai et al., 2021 ; Chang et al., 2022b ; Lin & Chang, 2020 ; Oliveira et al., 2021 ), and evaluate and adapt learning strategies they used during the task. In two of the studies, engagement in metacognitive judgement of learning was reported to be associated with increased student engagement in critical thinking (Chang et al., 2022b ) and writing performance (Lin & Chang, 2020 ), further confirming the potential of educational chatbots to support student metacognition, which is considered to be one of the central processes for productive SRL (Winne, 2018 ). Students’ internal conditions such as motivation, self-efficacy, and interest in a task, are often measured using self-report questionnaires, interviews and self-reflection prompts administered before or after the learning session. Data that dynamically capture student internal conditions as they evolve during the session is rarely collected, making it hard for educational technologies to provide immediate support adaptive to learning conditions. Even though some chatbots we reviewed have demonstrated ability to promote internal conditions, e.g., learning motivation (Yin et al., 2021 ), perception of learning (Neumann et al., 2021 ) and self-efficacy (Chang et al., 2022a ), the capability of educational chatbots to provide responses sensitive to evolving internal conditions remains limited. We also note that many chatbots in the reviewed corpus supported students to search for, gather and access learning resources for their tasks. As chatbots have been traditionally used in dialogue systems for customer service and information acquisition (Serban et al., 2017 ; Winkler & Söllner, 2018 ), we speculate the popularity of this feature in educational chatbots may have been naturally inherited from the field of customer service and adapted to support students as they gather learning content. Moreover, the recent explosion of advanced generative language models that generate sophisticated human-like responses and engage in natural language conversations, such as ChatGPT, has opened up new possibilities to improve educational chatbots from being tools mainly used for information acquisition to a powerful pedagogical tools that can revolutionize how students learn by offering personalized learning experiences and real-time guidance adapted to the student’s learning skills and knowledge of content.
We found that chatbots in the reviewed studies generally promoted increase in productive SRL processes and learning performance of students across different domains, confirming the potential of this technology to support SRL. Non-significant effects were identified in a group of studies and we attribute this finding to several possible reasons. Student motivation and engagement in learning sessions facilitated with chatbot may have dropped as many students may feel isolated in such learning context and may prefer direct support from teachers instead (Zhang et al., 2020 ). This may further lead to challenges in sustaining students’ learning interest in a task, as indicated in one of the reviewed studies (Fryer et al., 2017 ). As the reviewed chatbots have mainly supported university students, it may be expected that many students in this population already possessed a preferred catalogue of learning strategies and that one-time session with chatbot may not be sufficient to help those students alter their approaches to learning. Another reason for non-significant effects may be related to chatbot’s challenges to always provide satisfactory and accurate responses, that clearly target particular learning processes (Deveci Topal et al., 2021 ).
6 Conclusion and implications for further studies
The findings of this systematic literature review indicate the increasing interest of researchers in using educational chatbots to support self-regulated learning. The reviewed studies predominantly employed NLP-driven and rule-based chatbot architectures. Both architectures have shown potential in promoting various processes in SRL, particularly in the enactment of learning strategies and cognitive operations such as assembling, translating, and monitoring. Despite these advancements, the review identifies significant gaps in the comprehensive support of SRL. None of the chatbots have provided SRL support across all the four phases of SRL, as proposed by Winne and Hadwin Winne and Hadwin ( 1998 ). The support often involved guiding students through the steps within specific learning tasks rather than offering a holistic support to student SRL processing. The effects of chatbots on students’ SRL processes and learning performance appeared to be mixed. While many studies reported improvements in the use of learning strategies, student engagement, and self-efficacy, others found limited or non-significant effects on learning performance.
Based on the findings from this systematic literature review, we propose the following areas of investigation towards advancing research on chatbots and SRL.
1. Create chatbots that provide a comprehensive SRL support across all the phases
Our results suggest that, to date, there has been no chatbot designed to provide a comprehensive support across all the four phases of SRL defined in the Winne and Hadwin model. For instance, even though student engagement in goal settings, planning, and adaptation has been widely documented to benefit student learning experiences and performance (Alessandri et al., 2020 ; Raković et al., 2022a ; Rakovic et al., 2022b ), SRL processes at these stages have been rarely supported in the reviewed corpus, which may partially explain small, insignificant and limited effects of several chatbots on student achievements in this review. Within the SRL framework, each phase builds upon the previous one creating a cyclical process that allows students to continuously improve their learning strategies and accomplish their learning goals. Supporting studying in each phase of SRL may provide students with better control over their learning and may lead to greater academic success, increased confidence and motivation in one’s ability to learn.
2. Identify specific learning tasks in which chatbots can provide most effective support
While it is important to apply chatbots in different subject domains, it is equally important to identify specific tasks within those domains where chatbots can be most effective. By doing so, researchers and educators can ensure that chatbots are used in a targeted and effective manner, maximizing the impact of this technology on students’ learning experience. In this way, chatbots may help learners develop a catalogue of task-specific learning skills and transfer these skills to similar tasks in the future.
3. Evaluate the effectiveness of SRL chatbots in longitudinal studies
Most of the educational chatbots in this review have been evaluated in small scale studies, e.g., a one-time intervention administered in one class. Such a lack of longitudinal data might impede researchers from gaining a deeper understanding of the long term benefits of SRL chatbots. Therefore, it may benefit future research in educational technology and learning sciences if researchers conduct a longitudinal study, e.g., a study spanning over one or several semesters, to examine the effects of chatbot on the development of students’ SRL skills over time.
4. Use chatbot to elicit students’ internal conditions
Student internal conditions including prior knowledge, motivation, interest, self-efficacy, achievement goals, utility value and outcome expectations can have a significant impact on how learners approach and engage with the learning process (Meece, 2023 ). However, there are very limited existing efforts in learning analytics focusing on understanding and eliciting internal conditions (Matcha et al., 2019 ). These constructs have been typically measured at the beginning of a learning task. Since SRL is a dynamic and cyclic process (Panadero, 2017 ), student internal conditions may often change during the learning session, also affecting other processes that learners enact. For example, use of effective learning strategies and accomplishment of some learning goals early in a learning session may increase students’ self-efficacy and motivation later in the session, compared to what learners reported at the outset of the session. Given the conversational and interactive nature of chatbots, researchers may consider using this technology as an instrument that dynamically captures changes in internal conditions and helps learners to reflect on their own learning process, e.g., by engaging in dialogues with learners, asking questions that gauge students’ understanding, their learning goals and confidence levels, analyzing the content and frequency of student interactions, providing feedback on their progress, and offering suggestions for improvement.
5. Record and analyse what students did, not only what they say they did
Digital trace-data, e.g., navigation logs, text annotations and keystrokes, that students generate in digital learning environments have been increasingly harnessed to unobtrusively measure SRL (Fan et al., 2022 ; Rakovic et al., 2022b ; Lim et al., 2023 ). For instance, trace-data are often mapped to theorised SRL processes and dynamically analysed (e.g., by using process mining and natural language processing approaches) to obtain a more complete picture of student learning behaviors. To our knowledge, educational chatbots developed to date have mainly gathered information about student learning in two ways (1) via self-reports, e.g., based on what students said to the bot they did, and (2) via student performance data, e.g., correct/incorrect answers on a test. Researchers, however, have identified several challenges related to those methods. For example, students self-reports may often be insufficiently accurate and biased towards student beliefs or social desirability (Winne, 2022 ), whereas performance data often cannot directly inform the intervention (Arizmendi et al., 2022 ). Researchers may consider introducing trace-data as an additional input to SRL chatbots to ensure bots more accurately monitor student SRL processes as they dynamically unfold and, based on that, provide students with a more accurate and timely SRL support.
6. Support students at all educational levels
The chatbots in the reviewed corpus have mainly supported university students. More research is needed to adapt chatbots to cater to the needs of students at other levels of education, e.g., primary and secondary. Students at different levels of education may have different learning needs and preferences (Ambrose et al., 2010 ). By conducting research and adapting chatbots to cater these specific needs from different levels of students, we can ensure that the benefits of educational chatbots are accessible to students of all developmental stages, potentially creating more effective, engaging and inclusive learning environment for them. Also, university students have become more experienced learners and have often formed certain learning habits. This may make it challenging for the educational chatbot to induce some changes to learning. An early intervention at a primary or secondary education level could help in preparing students to better self-regulate their learning at a tertiary education level and throughout life.
7. Improve the effectiveness and accuracy of chatbot responses by harnessing the potential of large language models and generative AI
In our review, a notable limitation of current educational chatbots is their often unsatisfactory responses (Deveci Topal et al., 2021 ), highlighting a gap in understanding users’ intentions and providing relevant support. This indicates that the current chatbots may have limited ability to interpret users’ intentions and provide adequate support, which further may hamper student engagement and motivation. Researchers may utilise the rapidly emerging technologies of generative AI specifically large language models like ChatGPT, that can handle complex language problems, e.g., large language models such as ChatGPT, to enhance the chatbots’ ability to understand learners’ intentions and provide appropriate responses in the context of SRL. In this way, the volume of productive interactions between students and SRL chatbots may improve student learning experiences and interest in studying with a bot, marking a step forward in AI-driven education.
Data availability statement
Data sharing not applicable to this article as no datasets were generated or analyzed during the current study. This systematic literature review is based entirely on data from publicly available sources. The analysis synthesizes findings from a wide range of publications, including journal articles and conference papers, all of which are cited in the references section of this paper. These sources can be accessed through academic libraries and online databases. Supplementary materials created during this review, including summary tables (Figs. 5 - 9 ) and figures (Figs. 1 - 4 ), are available upon request.
Ait Baha, T., El Hajji, M., Es-Saady, Y., & Fadili, H. (2023). The impact of educational chatbot on student learning experience. Education and InformationTechnologies , 1–24.
Al-Abdullatif, A. M., Al-Dokhny, A. A., & Drwish, A. M. (2023). Implementing the bashayer chatbot in saudi higher education: Measuring the inuence on students’ motivation and learning strategies. Frontiers in Psychology, 14 , 1129070–1129070.
Article Google Scholar
Alessandri, G., Borgogni, L., Latham, G. P., Cepale, G., Theodorou, A., & De Longis, E. (2020). Self-set goals improve academic performance through nonlinear effects on daily study performance. Learning and Individual Differences, 77 , 101784.
Allison, D. (2012). Chatbots in the library: Is it time? Library Hi tech, 30 (1), 95–107.
Arizmendi, C. J., Bernacki, M. L., Raković, M., Plumley, R. D., Urban, C. J., Panter, A., Greene, J. A., & Gates, K. M. (2022). Predicting student outcomes using digital logs of learning behaviors: Review, current standards, and suggestions for future work. Behavior Research Methods , 1–29.
Ambrose, S. A., Bridges, M. W., DiPietro, M., Lovett, M. C., & Norman, M. K. (2010). How learning works: Seven research-based principles for smart teaching. John Wiley & Sons.
Azevedo, R. (2018). Using hypermedia as a metacognitive tool for enhancing student learning? the role of self-regulated learning. In: Educational psychologist (pp. 199–209). Routledge.
Azevedo, R., & Aleven, V. (2013). Metacognition and learning technologies: An overview of current interdisciplinary research. International Handbook of Metacognition and Learning Technologies , 1–16.
Azevedo, R., & Hadwin, A. F. (2005). Scaffolding self-regulated learning and metacognition - implications for the design of computer-based sca olds. Instructional Science, 33 (5/6), 367–379.
Azevedo, R., Taub, M., Mudrick, N. V., Millar, G. C., Bradbury, A. E., & Price, M. J. (2017). Using Data Visualizations to Foster Emotion Regulation During Self- Regulated Learning with Advanced Learning Technologies. In J. Buder & F. W. Hesse (Eds.), Informational environments : Effects of use, effective designs (pp. 225–247). https://doi.org/10.1007/978-3-319-64274-110
Bailey, D., Southam, A., & Costley, J. (2021). Digital storytelling with chatbots: Mapping l2 participation and perception patterns. Interactive Technology and Smart Education, 18 (1), 85–103.
Baker, R., Xu, D., Park, J., Yu, R., Li, Q., Cung, B., & Smyth, P. (2020). The benefits and caveats of using clickstream data to understand student self-regulatory behaviors: Opening the black box of learning processes. International Journal of Educational Technology in Higher Education, 17 , 1–24.
Bjork, R. A., Dunlosky, J., & Kornell, N. (2013). Self-regulated learning: Beliefs, techniques, and illusions. Annual Review of Psychology, 64 , 417–444.
Cai, W., Grossman, J., Lin, Z. J., Sheng, H., Wei, J.T.-Z., Williams, J. J., & Goel, S. (2021). Bandit algorithms to personalize educational chatbots. Machine Learning, 110 (9), 2389–2418.
Article MathSciNet Google Scholar
Chang, C.-Y., Hwang, G.-J., & Gau, M.-L. (2022). Promoting students’ learning achievement and self-efficacy: A mobile chatbot approach for nursing training. British Journal of Educational Technology, 53 (1), 171–188.
Chang, C.-Y., Kuo, S.-Y., & Hwang, G.-H. (2022). Chatbot-facilitated nursing education. Educational Technology & Society, 25 (1), 15–27.
Google Scholar
Chen, H.-L., Vicki Widarso, G., & Sutrisno, H. (2020). A chatbot for learning chinese: Learning achievement and technology acceptance. Journal of Educational Computing Research, 58 (6), 1161–1189.
Cleary, T. J., & Chen, P. P. (2009). Self-regulation, motivation, and math achievement in middle school: Variations across grade level and math context. Journal of School Psychology, 47 (5), 291–314.
Cleary, T. J., Kitsantas, A., Peters-Burton, E., Lui, A., McLeod, K., Slemp, J., & Zhang, X. (2022). Professional development in self-regulated learning: Shifts and variations in teacher outcomes and approaches to implementation. Teaching and Teacher Education, 111 , 103619.
Cooke, A., Smith, D., & Booth, A. (2012). Beyond pico: The spider tool for qualitative evidence synthesis. Qualitative Health Research, 22 (10), 1435–1443.
Dai, W., Lin, J., Jin, H., Li, T., Tsai, Y. S., Gašević, D., & Chen, G. (2023). Can large language models provide feedback to students? A case study on ChatGPT. In 2023 IEEE International Conference on Advanced Learning Technologies (ICALT) (pp. 323-325). IEEE.
Deveci Topal, A., Dilek Eren, C., & Kolburan Geçer, A. (2021). Chatbot application in a 5th grade science course. Education and Information Technologies, 26 (5), 6241–6265.
Dever, D. A., Sonnenfeld, N. A., Wiedbusch, M. D., Schmorrow, S. G., Amon, M. J., & Azevedo, R. (2023). A complex systems approach to analyzing pedagogical agents’ scaffolding of self-regulated learning within an intelligent tutoring system. Metacognition and Learning , 1–33.
Dignath, C., & Veenman, M. V. (2021). The role of direct strategy instruction and indirect activation of self-regulated learning|evidence from classroom observation studies. Educational Psychology Review, 33 (2), 489–533.
Du, J., Huang, W., & Hew, K. F. (2021). Supporting students goal setting process using chatbot: Implementation in a fully online course. 2021 IEEE International Conference on Engineering, Technology & Education (TALE) , 35–41.
Duffy, M. C., & Azevedo, R. (2015). Motivation matters: Interactions between achievement goals and agent scaffolding for self-regulated learning within an intelligent tutoring system. Computers in Human Behavior, 52 , 338–348.
Fan, Y., Lim, L., van der Graaf, J., Kilgour, J., Raković, M., Moore, J., Molenaar, I., Bannert, M., & Gašević, D. (2022). Improving the measurement of self-regulated learning using multi-channel data. Metacognition and Learning , 1–31.
Fryer, L. K., Ainley, M., Thompson, A., Gibson, A., & Sherlock, Z. (2017). Stimulating and sustaining interest in a language course: An experimental comparison of chatbot and human task partners. Computers in Human Behavior, 75 , 461–468.
Fryer, L. K., Thompson, A., Nakao, K., Howarth, M., & Gallacher, A. (2020). Supporting self-efficacy beliefs and interest as educational inputs and outcomes: Framing ai and human partnered task experiences. Learning and Individual Differences, 80 , 101850.
Greene, J. A., & Azevedo, R. (2007). A theoretical review of winne and hadwin’s model of self-regulated learning: New perspectives and directions. Review of Educational Research, 77 (3), 334–372.
Gutierrez de Blume, A. P. (2022). Calibrating calibration: A meta-analysis of learning strategy instruction interventions to improve metacognitive monitoring accuracy. Journal of Educational Psychology, 114 (4), 681.
Harati, H., Sujo-Montes, L., Tu, C.-H., Armfield, S. J., & Yen, C.-J. (2021). Assessment and learning in knowledge spaces (aleks) adaptive system impact on students’ perception and self-regulated learning skills. Education Sciences, 11 (10), 603.
Hew, K. F., Huang, W., Du, J., & Jia, C. (2021). Using chatbots in ipped learning online sessions: Perceived usefulness and ease of use. International Conference on Blended Learning , 164–175.
Hew, K. F., Huang, W., Du, J., & Jia, C. (2023). Using chatbots to support student goal setting and social presence in fully online activities: Learner engagement and perceptions. Journal of Computing in Higher Education, 35 (1), 40–68.
Illescas-Manzano, M. D., Vicente López, N., Afonso González, N., & Cristofol Rodríguez, C. (2021). Implementation of chatbot in online commerce, and open innovation. Journal of Open Innovation: Technology, Market, and Complexity, 7 (2), 125.
Jeon, J. (2021). Chatbot-assisted dynamic assessment (ca-da) for l2 vocabulary learning and diagnosis. Computer Assisted Language Learning , 1–27.
Jivet, I., Scheffel, M., Schmitz, M., Robbers, S., Specht, M., & Drachsler, H. (2020). From students with love: An empirical study on learner goals, self-regulated learning and sense-making of learning analytics in higher education. The Internet and Higher Education, 47 , 100758.
Jivet, I., Wong, J., Scheffel, M., Valle Torre, M., Specht, M., & Drachsler, H. (2021). Quantum of choice: How learners’ feedback monitoring decisions, goals and self-regulated learning skills are related. LAK21: 11th International Learning Analytics and Knowledge Conference , 416–427.
Jones, A., & Castellano, G. (2018). Adaptive robotic tutors that support self-regulated learning: A longer-term investigation with primary school children. International Journal of Social Robotics, 10 (3), 357–370.
Klug, J., Ogrin, S., Keller, S., Ihringer, A., & Schmitz, B. (2011). A plea for selfregulated learning as a process: Modelling, measuring and intervening.
Kumar, J. A. (2021). Educational chatbots for project-based learning: Investigating learning outcomes for a team-based design course. International Journal of Educational Technology in Higher Education, 18 (1), 1–28.
Li, Y., Sha, L., Yan, L., Lin, J., Raković, M., Galbraith, K., Lyons, K., Gašević, D., & Chen, G. (2023). Can large language models write reflectively. Computers and Education: Artificial Intelligence, 4 , 100140. https://doi.org/10.1016/j.caeai.2023.100140 .
Lim, L., Bannert, M., van der Graaf, J., Singh, S., Fan, Y., Surendrannair, S., Rakovic, M., Molenaar, I., Moore, J., & Gašević, D. (2023). Effects of real-time analytics-based personalized scaffolds on students’ self-regulated learning. Computers in Human Behavior , 107547.
Lin, M.P.-C., & Chang, D. (2020). Enhancing post-secondary writers’ writing skills with a chatbot. Journal of Educational Technology & Society, 23 (1), 78–92.
List, A., & Du, H. (2021). Reasoning beyond history: Examining students’ strategy use when completing a multiple text task addressing a controversial topic in education. Reading and Writing, 34 (4), 1003–1048.
List, A., & Lin, C.-J. (2023). Content and quantity of highlights and annotations predict learning from multiple digital texts. Computers & Education, 199 , 104791.
Mageira, K., Pittou, D., Papasalouros, A., Kotis, K., Zangogianni, P., & Daradoumis, A. (2022). Educational ai chatbots for content and language integrated learning. Applied Sciences, 12 (7), 3239.
Matcha, W., Gašević, D., Pardo, A., et al. (2019). A systematic review of empirical studies on learning analytics dashboards: A self-regulated learning perspective. IEEE Transactions on Learning Technologies, 13 (2), 226–245.
McCardle, L., Webster, E. A., Haffey, A., & Hadwin, A. F. (2017). Examining students’ self-set goals for self-regulated learning: Goal properties and patterns. Studies in Higher Education, 42 (11), 2153–2169.
McTear, M. (2020). Conversational ai: Dialogue systems, conversational agents, and chatbots. Synthesis Lectures on Human Language Technologies, 13 (3), 1–251.
Meece, J. L. (2023). The role of motivation in self-regulated learning. In Self-regulation of learning and performance (pp. 25–44). Routledge.
Mellado-Silva, R., Faúndez-Ugalde, A., & Blanco-Lobos, M. (2020). Effective learning of tax regulations using different chatbot techniques. Advances in Science, Technology and Engineering Systems, 5 (6), 439–446.
Moher, D., Liberati, A., Tetzlaff, J., Altman, D. G., Altman, D., Antes, G., . . . Berlin, J. A., et al. (2009). Preferred reporting items for systematic reviews and metaanalyses: The prisma statement (chinese edition). Journal of Chinese Integrative Medicine , 7 (9), 889–896.
Molenaar, I. (2022). The concept of hybrid human-ai regulation: Exemplifying how to support young learners’ self-regulated learning. Computers and Education: Artificial Intelligence, 3 , 100070.
Molenaar, I., Roda, C., van Boxtel, C., & Sleegers, P. (2012). Dynamic scaffolding of socially regulated learning in a computer-based learning environment. Computers & Education, 59 (2), 515–523.
Morisano, D., Hirsh, J. B., Peterson, J. B., Pihl, R. O., & Shore, B. M. (2010). Setting, elaborating, and reecting on personal goals improves academic performance. Journal of Applied Psychology, 95 (2), 255.
Neumann, A. T., Arndt, T., Köbis, L., Meissner, R., Martin, A., de Lange, P., & Wollersheim, H.-W. (2021). Chatbots as a tool to scale mentoring processes: Individually supporting self-study in higher education. Frontiers in Artificial Intelligence, 4 , 668220.
Oliveira, E., de Barba, P. G., & Corrin, L. (2021). Enabling adaptive, personalised and context-aware interaction in a smart learning environment: Piloting the icollab system. Australasian Journal of Educational Technology, 37 (2), 1–23.
Page, M. J., McKenzie, J. E., Bossuyt, P. M., Boutron, I., Hoffmann, T. C., Mulrow, C. D., Brennan, S. E., et al. (2021). The prisma 2020 statement: An updated guideline for reporting systematic reviews. Systematic Reviews, 10 (1), 1–11.
Panadero, E. (2017). A review of self-regulated learning: Six models and four directions for research. Frontiers in Psychology , 422.
Panadero, E., Klug, J., & Järvelä, S. (2016). Third wave of measurement in the self-regulated learning field: When measurement and intervention come hand in hand. Scandinavian Journal of Educational Research, 60 (6), 723–735.
Pérez, J. Q., Daradoumis, T., & Puig, J. M. M. (2020). Rediscovering the use of chatbots in education: A systematic literature review. Computer Applications in Engineering Education, 28 (6), 1549–1565.
Perez-Alvarez, R., Jivet, I., Pérez-Sanagustin, M., Scheffel, M., & Verbert, K. (2022). Tools designed to support self-regulated learning in online learning environments: A systematic review. IEEE Transactions on Learning Technologies .
Pintrich, P. R. (2000). The role of goal orientation in self-regulated learning. In: Handbook of self-regulation (pp. 451–502). Elsevier.
Raković, M., Bernacki, M. L., Greene, J. A., Plumley, R. D., Hogan, K. A., Gates, K. M., & Panter, A. T. (2022a). Examining the critical role of evaluation and adaptation in self-regulated learning. Contemporary Educational Psychology, 68 , 102027.
Rakovic, M., Fan, Y., Van Der Graaf, J., Singh, S., Kilgour, J., Lim, L., Moore, J., Bannert, M., Molenaar, I., & Gasevic, D. (2022b). Using learner trace data to understand metacognitive processes in writing from multiple sources. LAK22: 12th International Learning Analytics and Knowledge Conference , 130–141.
Recommendation, E. C. (2018). Key competences for lifelong learning. [SPACE] https://doi.org/10.1111/bjep.12173
Serban, I. V., Sankar, C., Germain, M., Zhang, S., Lin, Z., Subramanian, S., . . . Ke, N. R., et al. (2017). A deep reinforcement learning chatbot. arXiv:1709.02349 .
Smutny, P., & Schreiberova, P. (2020). Chatbots for learning: A review of educational chatbots for the facebook messenger. Computers & Education, 151 , 103862.
Song, D., & Kim, D. (2021). Effects of self-regulation scaffolding on online participation and learning outcomes. Journal of Research on Technology in Education, 53 (3), 249–263.
Srivastava, N., Fan, Y., Rakovic, M., Singh, S., Jovanovic, J., Van Der Graaf, J., Lim, L., Surendrannair, S., Kilgour, J., Molenaar, I., et al. (2022). Effects of internal and external conditions on strategies of self-regulated learning: A learning analytics study. LAK22: 12th International Learning Analytics and Knowledge Conference , 392–403.
Sweidan, S. Z., Abu Laban, S. S., Alnaimat, N. A., & Darabkh, K. A. (2021). Siaaa-c: A student interactive assistant android application with chatbot during covid-19 pandemic. Computer Applications in Engineering Education, 29 (6), 1718–1742.
Taub, M., Azevedo, R., Rajendran, R., Cloude, E. B., Biswas, G., & Price, M. J. (2021). How are students’ emotions related to the accuracy of cognitive and metacognitive processes during learning with an intelligent tutoring system? Learning and Instruction, 72 , 101200.
Theobald, M. (2021). Self-regulated learning training programs enhance university students’ academic performance, self-regulated learning strategies, and motivation: A meta-analysis. Contemporary Educational Psychology, 66 , 101976.
Tian, X., Risha, Z., Ahmed, I., Lekshmi Narayanan, A. B., & Biehl, J. (2021). Let’s talk it out: A chatbot for effective study habit behavioral change. Proceedings of the ACM on Human-computer Interaction, 5 (CSCW1), 1–32.
Vázquez-Cano, E., Mengual-Andrés, S., & López-Meneses, E. (2021). Chatbot to improve learning punctuation in spanish and to enhance open and exible learning environments. International Journal of Educational Technology in Higher Education, 18 (1), 1–20.
Weizenbaum, J. (1966). Eliza|a computer program for the study of natural language communication between man and machine. Communications of the ACM, 9 (1), 36–45.
Winkler, R., & Söllner, M. (2018). Unleashing the potential of chatbots in education: A state-of-the-art analysis. Academy of Management Annual Meeting (AOM) .
Winne, P. H. (1995). Inherent details in self-regulated learning. Educational Psychologist, 30 (4), 173–187.
Winne, P. H. (2018). Theorizing and researching levels of processing in self-regulated learning. British Journal of Educational Psychology, 88 (1), 9–20.
Winne, P. H. (2022). Modeling self-regulated learning as learners doing learning science: How trace data and learning analytics help develop skills for self-regulated learning. Metacognition and Learning , 1–19.
Winne, P. H., & Hadwin, A. F. (1998). Studying as self-regulated engagement in learning. in metacognition in educational theory and practice. Metacognition in Educational Theory and Practice , 277–304.
Wollny, S., Schneider, J., Di Mitri, D., Weidlich, J., Rittberger, M., & Drachsler, H. (2021). Are we there yet?-a systematic literature review on chatbots in education. Frontiers in Artificial Intelligence, 4 , 654924.
Wu, E.H.-K., Lin, C.-H., Ou, Y.-Y., Liu, C.-Z., Wang, W.-K., & Chao, C.-Y. (2020). Advantages and constraints of a hybrid model k-12 e-learning assistant chatbot. Ieee Access, 8 , 77788–77801.
Xia, Q., Chiu, T. K. F., Chai, C. S., & Xie, K. (2023). The mediating effects of needs satisfaction on the relationships between prior knowledge and self-regulated learning through artificial intelligence chatbot. British Journal of Educational Technology, 54 (4), 967–986.
Yin, J., Goh, T.-T., Yang, B., & Xiaobin, Y. (2021). Conversation technology with micro-learning: The impact of chatbot-based learning on students’ learning motivation and performance. Journal of Educational Computing Research, 59 (1), 154–177.
Zawacki-Richter, O., Marín, V. I., Bond, M., & Gouverneur, F. (2019). Systematic review of research on artificial intelligence applications in higher education- where are the educators? International Journal of Educational Technology in Higher Education, 16 (1), 1–27.
Zhang, J.-H., Zou, L.-C., Miao, J.-J., Zhang, Y.-X., Hwang, G.-J., & Zhu, Y. (2020). An individualized intervention approach to improving university students’ learning performance and interactive behaviors in a blended learning environment. Interactive Learning Environments, 28 (2), 231–245.
Zhang, R., Zou, D., & Cheng, G. (2023a). Chatbot-based learning of logical fallacies in e writing: Perceived effectiveness in improving target knowledge and learner motivation (pp. 1–18). Ahead-of-print(ahead-of-print): Interactive Learning Environments.
Zhang, R., Zou, D., & Cheng, G. (2023b). Chatbot-based training on logical fallacy in efl argumentative writing. Innovation in Language Learning and Teaching, 17 (5), 932–945.
Zimmerman, B. J. (2000). Attaining self-regulation: A social cognitive perspective. In Handbook of self-regulation (pp. 13–39). Elsevier.
Zimmerman, B. J. (2002). Becoming a self-regulated learner: An overview. Theory Into Practice, 41 (2), 64–70.
Zimmerman, B. J. (2013). Theories of self-regulated learning and academic achievement: An overview and analysis. Self-regulated Learning and Academic Achievement , 1–36.
Download references
Acknowledgements
This work was in part supported by funding from the Australian Research Council (DP220101209, DP240100069) and Jacobs Foundation.
Open Access funding enabled and organized by CAUL and its Member Institutions.
Author information
Authors and affiliations.
Centre for Learning Analytics at Monash, Faculty of Information Technology, Monash University, 25 Exhibition walk, Clayton, VIC, 3800, Australia
Rui Guan, Mladen Raković, Guanliang Chen & Dragan Gašević
School of Informatics, University of Edinburgh, Edinburgh, UK
Dragan Gašević
You can also search for this author in PubMed Google Scholar
Contributions
Rui Guan: Conceptualization, Methodology, Formal analysis, Writing - Original Draft, Data Curation.Mladen Raković: Conceptualization, Methodology, Formal analysis, Writing - Original Draft, Data Curation, Supervision.Guanliang Chen: Conceptualization, Methodology, Writing - Original Draft, Supervision.Dragan Gašević: Conceptualization, Methodology, Writing - Original Draft, Supervision
Corresponding author
Correspondence to Mladen Raković .
Ethics declarations
Competing interest.
The authors have no competing interests to declare that are relevant to the content of this article.
Financial Interest
All authors certify that they have no affiliations with or involvement in any organization or entity with any financial interest or non-financial interest in the subject matter or materials discussed in this manuscript.
Ethical Standard
This systematic review has been conducted in accordance with the principles of academic integrity and honesty. All sources have been properly cited, and no copyrighted material has been used without permission. The review does not involve any original data collection from human or animal subjects, and therefore, ethical approval was not sought.
Appendix A: Summary of the reviewed studies
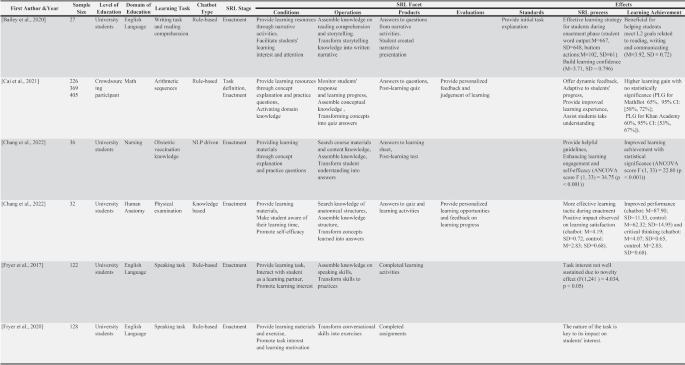
Summary table of reviewed chatbot articles including supported SRL stages and facets
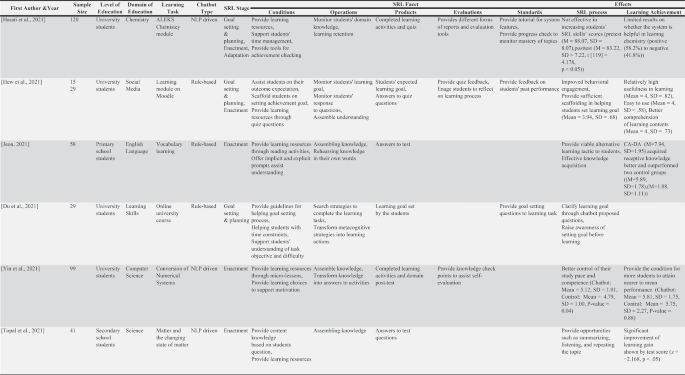
Rights and permissions
Open Access This article is licensed under a Creative Commons Attribution 4.0 International License, which permits use, sharing, adaptation, distribution and reproduction in any medium or format, as long as you give appropriate credit to the original author(s) and the source, provide a link to the Creative Commons licence, and indicate if changes were made. The images or other third party material in this article are included in the article’s Creative Commons licence, unless indicated otherwise in a credit line to the material. If material is not included in the article’s Creative Commons licence and your intended use is not permitted by statutory regulation or exceeds the permitted use, you will need to obtain permission directly from the copyright holder. To view a copy of this licence, visit http://creativecommons.org/licenses/by/4.0/ .
Reprints and permissions
About this article
Guan, R., Raković, M., Chen, G. et al. How educational chatbots support self-regulated learning? A systematic review of the literature. Educ Inf Technol (2024). https://doi.org/10.1007/s10639-024-12881-y
Download citation
Received : 18 March 2024
Accepted : 21 June 2024
Published : 30 August 2024
DOI : https://doi.org/10.1007/s10639-024-12881-y
Share this article
Anyone you share the following link with will be able to read this content:
Sorry, a shareable link is not currently available for this article.
Provided by the Springer Nature SharedIt content-sharing initiative
- Educational chatbot
- Self-regulated learning
- Scaffolding
- Artificial intelligence
- Find a journal
- Publish with us
- Track your research
Thank you for visiting nature.com. You are using a browser version with limited support for CSS. To obtain the best experience, we recommend you use a more up to date browser (or turn off compatibility mode in Internet Explorer). In the meantime, to ensure continued support, we are displaying the site without styles and JavaScript.
- View all journals
- Explore content
- About the journal
- Publish with us
- Sign up for alerts
- Open access
- Published: 27 August 2024
Charting sustainable urban development through a systematic review of SDG11 research
- Abdulaziz I. Almulhim ORCID: orcid.org/0000-0002-5384-7219 1 ,
- Ayyoob Sharifi ORCID: orcid.org/0000-0002-8983-8613 2 ,
- Yusuf A. Aina ORCID: orcid.org/0000-0002-0763-9865 3 ,
- Shakil Ahmad 4 ,
- Luca Mora 5 , 6 ,
- Walter Leal Filho 7 , 8 &
- Ismaila Rimi Abubakar ORCID: orcid.org/0000-0002-7994-2302 9
Nature Cities ( 2024 ) Cite this article
1384 Accesses
1 Altmetric
Metrics details
- Environmental studies
- Social policy
The Sustainable Development Goal (SDG) 11 underscores the imperative of creating inclusive, safe, resilient and sustainable cities and communities by 2030. Here we employ bibliometric techniques to assess the evolving landscape of SDG11 research. Using a comprehensive dataset of over 21,000 scholarly publications, we investigate publication trends, thematic focus areas, authorship patterns, keyword co-occurrences and citation networks related to SDG11 research. The results reveal a consistent increase in research output, reflecting the growing global interest in urban sustainability studies. We identify influential authors, organizations and countries shaping the research landscape, highlighting existing global collaborative networks and emerging research hubs. Core thematic areas emphasize critical topics and interdisciplinary connections. Citation networks underscore the impacts of disseminating research outputs, including seminal works. This study offers insights for policymakers, academics and practitioners to align their collective efforts toward sustainable, inclusive and climate-resilient urban development. Moreover, it advances SDG11 by noting opportunities for further research, knowledge dissemination and international collaboration.
Similar content being viewed by others
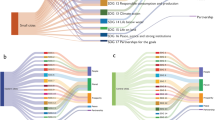
A big data approach to assess progress towards Sustainable Development Goals for cities of varying sizes
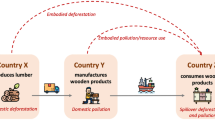
Examining the unsustainable relationship between SDG performance, ecological footprint and international spillovers
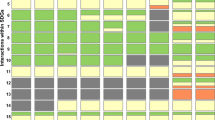
Sustainable Development Goals (SDGs): Are we successful in turning trade-offs into synergies?
The growing interest in sustainable urban development is driven by challenges posed by urbanization, socioeconomic activities and environmental issues 1 . Urban areas contribute 80% of the world’s gross domestic product 2 , but also account for around 75% of global resource consumption, 65% of energy use and over 70% of carbon emissions 3 . The ecological footprint of urban environments, which measures the resources required to sustain socioeconomic activities, has been increasing 4 , 5 , and the global urban extent is projected to double by 2030 6 . Similarly, the global urban population is projected to reach 68% by 2050 7 , which could surpass the capacity of most urban areas 8 . Africa and Asia will host most of the future urban populations despite housing and infrastructure inadequacies 7 . Rapid urbanization, poverty and climate change (CC) further intensify the vulnerability of urban dwellers 9 .
Sustainable urban development aims to balance economic production, environmental protection and social inclusiveness. It emerged as a response to the critique of modernist views that prioritized physical appearance and order in cities over context, equity and inclusion 6 . Due to the limited progress in achieving the Millennium Development Goals, the Sustainable Development Goals (SDGs) were established in 2015 to ensure that no country is left behind in achieving sustainable development by 2030 10 . Many of the SDGs are closely related to urban settings, where sustainability challenges are complex and interwoven 11 . SDG11 specifically focuses on urban challenges and aims to make ‘cities and human settlements inclusive, safe resilient and sustainable’ by reducing the negative effects of urban development while improving socioeconomic development 10 .
The importance of SDG11 stems from the principles of inclusive, safe and resilient city. An inclusive city is characterized by the idea that all individuals, irrespective of their economic status, gender, race, ethnicity or religion, have the ability and empowerment to actively engage in the social, economic and political opportunities available within urban environments 6 . It seeks to address environmental racism and promote inclusive and fair urban development through social justice and equitable distribution of environmental benefits and burdens. In such a city, everyone is afforded equal access and participation in the diverse aspects that cities provide. On the other hand, a safe city refers to a city that possesses the capacity to provide protection and security against potential dangers, harm or risks, while a resilient city denotes a city’s ability to recover and restore its fundamental functions and structures following natural disasters and crises caused by human activities 6 , 8 . SDG11 is significant because it aims to ensure that cities develop sustainably.
However, SDG11 has been criticized for its limited emphasis on urban inequalities, decentralization and funding for local authorities 6 . Other challenges include localizing the universal indicators 12 , governance issues 13 , data accessibility and comparability 14 and smart city development 12 , 15 . Nevertheless, SDG11 serves as a platform for directing and monitoring urban development, fostering socioeconomic development and ensuring equity, inclusion and environmental protection 16 . Therefore, it is crucial to assess the literature on progress toward SDG11 targets 10 , especially at the halfway point to the target year, to inform interventions necessary for their achievement 17 .
While SDG11 has attracted significant global research attention 18 , comprehensive reviews of SDG11 literature are limited. Existing studies have primarily focused on assessing all the SDGs 19 , 20 , which obscures specific challenges and makes it difficult to track progress or design targeted interventions for individual goals. Recent work has highlighted the insufficient achievement of the SDGs and the need for transformative governance and participatory approaches 21 . Other studies have underscored the gap between research and policies, the underutilization of cities as pivotal arenas for achieving SDGs 22 and the lack of indicators to measure progress toward implementing SDGs 15 . Some studies have assessed SDGs’ implementation in specific region 17 , their impacts on addressing risks 23 and crises 1 , and their implications for health and well-being 24 , environmental research 25 and private sector involvement 26 . Most of the SDG research emanates from developed countries, showing a gap in the coverage of developing countries 27 . The few SDG11 studies in the Global South have narrow focus. While one paper investigated the impact of SDG11 on forest-based livelihoods 28 , another study researched the challenges of SDG11 implementation using a single-country experience 6 . Therefore, an in-depth and broad review of SDG11 literature is necessary to bridge this knowledge gap and identify key challenges and opportunities as well as potential pathways for achieving the targets set in SDG11.
Therefore, this research aims to assess the SDG11 research trends and themes using a bibliometric technique. It is the first global and comprehensive scientometric study on the SDG11 domain. By focusing on research conducted since the formulation of the SDGs, the study addresses the following research questions: (1) what are the global trends in SDG11 research? (2) How has the thematic focus of SDG11 research evolved over time? (3) What are the challenges and priority areas for SDG11 research? The contributions of the study to theory and practice are to:
Identify significant thematic areas and trends in SDG11 research since the promulgation of the SDGs, which can inform researchers, policymakers and practitioners about the current state of knowledge within the field and highlight priority areas for SDG11 research.
Map research clusters, knowledge sharing and collaboration patterns, thereby providing insights into the dynamics of research networks and facilitating the formulation of strategies to foster research excellence, interdisciplinary and international collaborations and the effective allocating of research resources.
Underscore the knowledge gaps, emerging topics and challenges within SDG11 research, offering evidence-based insights to align urban development initiatives with SDG11 research frontiers, enhance the efficacy of interventions and contribute to the development of inclusive, safe, resilient and sustainable cities.
SDG11 research trends
Research on SDG11 has significantly grown in terms of annual publications and citations since 2016, indicating a rising interest in this field (Fig. 1 ). The number of publications has increased by 1.3-fold, and this upward trajectory is expected to continue. Notable emerging research areas include the institutionalization of SDGs within local and global settings 18 and the impact of smart cities on advancing the SDGs 12 , 15 . Previously, studies on the epistemology and challenges of urban population growth were prevalent 29 . However, SDG11 research has now evolved into multidisciplinary fields, driven by heightened attention to urban challenges such as CC, urbanization and population growth.
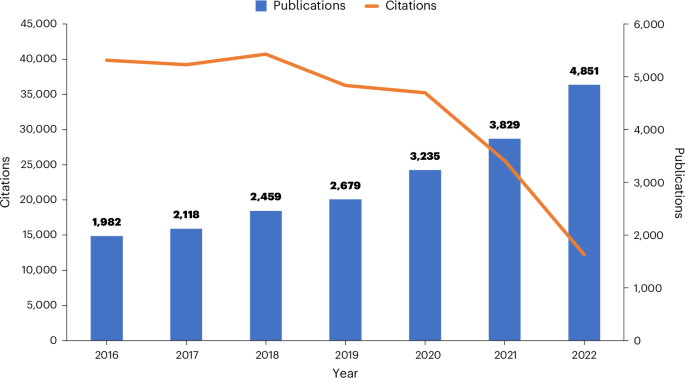
A total of 21,153 articles were published, receiving 229,182 citations. The number of publications rose from 9,238 in period 1 (2016–2019) to 11,915 in period 2 (2020–2022).
Source data
The increasing trend in SDG11 publications can be attributed to several factors, including the desire to improve institutional rankings, a supportive research environment, investments and endowments, faculty promotion requirements and advancements in information and communication technology. There are also socioeconomic factors, such as increasing urbanization rates and gross domestic product, urban expansion and transformation, a deeper understanding of urban dynamics and challenges. Additionally, the policy environments in different countries can influence academic interests and research in urban studies, shaping research priorities and collaborations. Other contributing factors include research challenges faced by low-income countries and research support by governments, the private sector, international development agencies and scholars, all focusing on sustainable urban development.
SDG11 research is further propelled by recent international summits and collaborations that highlight the urgency of protecting the ecosystem and ensuring human safety 1 . Since 2015, CC issues have received greater attention due to key factors. The adoption of the Paris Agreement raised awareness and urgency for action on CC, resulting in a greater focus on related issues in various sectors, including urban planning and policy 13 . Scientific consensus on CC impacts and the role of human activities has also strengthened over the years, with Intergovernmental Panel on Climate Change assessments emphasizing the significance of cities in addressing CC 23 . As a result, CC considerations are increasingly integrated into research, policy and planning processes.
Urban planning and development strategies have prioritized climate mitigation and adaptation measures, such as reducing greenhouse gas emissions, promoting renewable energy, enhancing resilience to extreme weather events and incorporating green infrastructure. The focus on CC has accelerated the transition toward low-carbon and resilient cities, with efforts directed toward sustainable transportation, energy-efficient buildings, green spaces and climate-responsive infrastructure 6 . Collaboration and international cooperation are essential in addressing climate change, with cities and countries sharing best practices, knowledge and resources to develop and implement climate action plans 24 . Initiatives such as the C40 Cities Climate Leadership Group facilitate knowledge exchange and collective action among cities 30 . The increased attention to CC signifies a shift toward more sustainable and resilient urban development, emphasizing the need for proactive measures to mitigate greenhouse gas emissions, adapt to climate risks and promote equitable and sustainable urban environments.
Thematic focus of SDG11 research
There is an imbalance in the attention given to research themes within SDG11 as revealed by co-occurrence map (Supplementary Fig. 1 ). The dominant themes are affordable housing (SDG11.1), urban transport (SDG11.2), policy and governance (SDG11.3) and access to public spaces (SDG11.7). Housing affordability issues have consistently remained a focal point in SDG11 research, with urban studies, policy development and community-driven efforts for finding solutions to these complex challenges 30 , 31 . These issues were highlighted in Habitat I (Vancouver, 1976), which emphasized the importance of shifting governance and planning paradigms to develop policies and strategies to address rapid urbanization challenges, including shelter shortages and urban inequalities, and promote affordable housing options 30 , 32 . Habitat I has laid the foundation for subsequent global efforts and policy frameworks, such as Habitat II (Istanbul, 1996) and the New Urban Agenda, which continue to prioritize housing as a pivotal component of sustainable urban development. The persistent focus on affordable housing shows that cities still face many challenges in providing adequate housing for all 30 .
Urban policy and governance are other significant terms, indicating scholarly focus on strategies for promoting inclusive and sustainable urban development, enhancing participatory, integrated and sustainable urban planning and management. However, many cities lack the capacity to address urban inequalities, provide adequate housing 31 , public spaces and other urban services, which disproportionately affect women and racial minorities 30 . Moreover, urban redevelopment practices that lead to gentrification exacerbate existing inequalities 32 . Governance-based approaches seek to improve collaboration between public agencies and civil society to prioritize the implementation of urban planning strategies that enhance livability standards while addressing challenges such as CC and sustainability 30 .
Urban transport, which is related to SDG11.2 aiming to ensure safe, affordable, accessible and sustainable transport systems for all, has emerged as a key research theme. Important issues related to mobility, transportation and urban form include increased automobile dependence amid growing urbanization and suburbanization, challenges faced by public transit systems, growing awareness of environmental concerns, shift toward sustainable and multimodal transportation, transit-oriented development, integration of technology in transportation systems and the relationship between transportation and urban densification, compact development, CC adaptation and resilience, equity and social inclusion, and shifts in policy and governance approaches 1 , 6 , 11 . This theme also emphasizes the importance of walkability, public transit infrastructure and their role in enhancing transportation accessibility and influencing mode choice 33 . The transportation cluster also suggests that improving accessibility through urban form and built environment interventions can impact the travel behavior of urban residents and offer cobenefits for human health and environmental sustainability 24 . Incorporating such cobenefits in SDG11.2 could provide more incentives for access to safe efficient, equitable and sustainable transport infrastructure and systems in cities.
The implications of urbanization and land-use changes for sustainability, resilience and CC adaptation and mitigation in cities are also major themes. SDG11.6 aims to reduce the environmental impacts of cities, particularly in relation to air pollution and waste. The literature suggests that regulating urban growth 6 , controlling land-use changes, conserving biodiversity 27 and promoting green infrastructure are essential for achieving this target 34 . These actions, when implemented within integrated planning frameworks, can also reduce vulnerability, enhance resilience and contribute to progress in CC adaptation and mitigation, as emphasized in SDG11.5 (ref. 6 ). Such integrated frameworks should recognize the interconnections between various urban systems, including water, food, energy, waste and transportation, to promote sustainable and resilient urban development 35 . Cities are adopting strategies to reduce their carbon footprint, enhance energy efficiency and prepare for climate risks.
Smart cities and innovation enabled by information and communication technologies have increasingly been utilized to tackle urban development challenges and facilitate innovative and transformative urban governance mechanisms that contribute to the SDGs 15 . The rapid development and integration of digital technologies, such as the Internet of Things, artificial intelligence, big data analytics and sensor networks, have opened new possibilities for improving urban services, infrastructure and quality of life 33 . Smart cities leverage these technologies to enhance efficiency, connectivity and sustainability. The interest in smart cities stems from the recognition that technology can play a transformative role in addressing urban challenges, improving quality of life, promoting sustainability and fostering economic growth 12 , 36 . However, it is important to ensure that smart city initiatives are inclusive, equitable and responsive to the needs and aspirations of all residents.
Comparing the co-occurrence maps of period 1 and period 2 reveals limited changes in key thematic areas, despite the emergence of the coronavirus disease 2019 (COVID-19) pandemic during period 2 (Fig. 2 ). The key thematic areas in period 2, including urban governance and policy, transportation, urban sustainability and resilience, and urbanization and urban growth, remain consistent with period 1, indicating the continued relevance of these topics in research, albeit with potential expansions. However, a closer analysis of the clusters reveals that COVID-19 has emerged as a new area of SDG11 research in period 2, as attention has shifted toward adapting to the pandemic’s detrimental effects on cities. The pandemic has triggered paradigm shifts in various SDG11 domains, including public health, remote work, digitalization, vulnerabilities, inequalities, resilience, sustainability, urban spaces, proximity-based planning approaches such as the 15-minute city and global cooperation 9 . These shifts have influenced work, health, social equity, environmental stewardship 2 and urban planning, shaping innovative approaches and priorities in the postpandemic world. Urban inequality terms, such as slums and informality, inadequate housing and poverty, are brought to the forefront by the pandemic. Controlling the pandemic and addressing the citizen demand in slums and informal settlements has received significant attention 37 , 38 , 39 , 40 . Mobility restrictions and lockdowns to curb the virus’s transmission have presented challenges for service accessibility, particularly in disadvantaged neighborhoods where vulnerable groups reside. Lastly, the connection between sustainability and resilience has strengthened in the postpandemic period. The pandemic has offered new insights into the susceptibility of cities to various stressors and highlighted the inseparable connections between urban resilience and SDG11 (ref. 28 ).
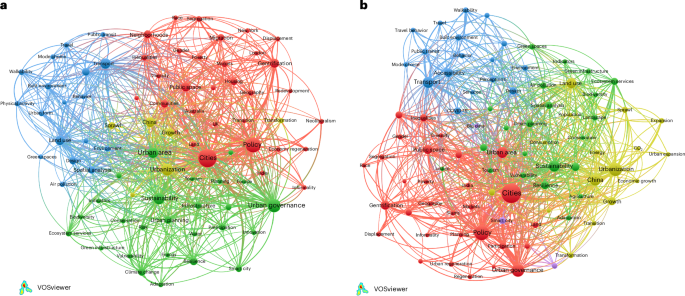
a , b , The key thematic areas in period 1 (2016–2019) ( a ) are urban governance and policy (red), transportation (blue), urban sustainability and resilience (green), and urbanization and urban growth (yellow), while period 2 (2020–2022) ( b ) primarily focuses on urban governance and policies (red), urban studies (red), transportation (blue) and urbanization (green), particularly after the pandemic.
However, three SDG11 targets are not well-represented in both periods. One such target is SDG11.4, which aims to enhance efforts in preserving and conserving natural heritage, vital for improving urban sustainability 41 . Another target, SDG11.a, which focuses on strengthening urban–rural linkages, is also not adequately reflected in Fig. 2 . The intrinsic connection between cities and their surrounding rural areas necessitates the incorporation and strengthening of ties between urban and rural regions to achieve SDG11 (ref. 6 ). Gaps related to rural–urban linkages include limited understanding of interdependencies, inadequate infrastructure and services in rural areas, weak governance and coordination mechanisms, and social and cultural disconnect 13 . These gaps hinder the development of integrated strategies, contribute to economic disparities, limit access to services, impact agricultural productivity and food security, and create environmental and social challenges. Lastly, there is a lack of research on SDG11.c, which aims to support least-developed nations in developing safe and resilient urban areas, which is not surprising as these countries are often underrepresented in urban studies research 30 .
Major contributors to SDG11 research
Various countries, institutions, journals and authors have contributed to SDG11 research between 2016 and 2022. China leads in terms of the number of publications and citations generated, followed by the United States and the United Kingdom (Supplementary Fig. 2 and Supplementary Table 1 ). Among the top 20 productive countries, 14 are from the Global North countries, with South Africa and Brazil as the sole representative of Africa and Latin America and the Caribbean, respectively (Supplementary Fig. 3 and Supplementary Table 2 ). Increasing research collaboration among the top countries (Fig. 3 ), research infrastructure and facilities, manpower and financial support significantly contribute to their high SDG11 research output.
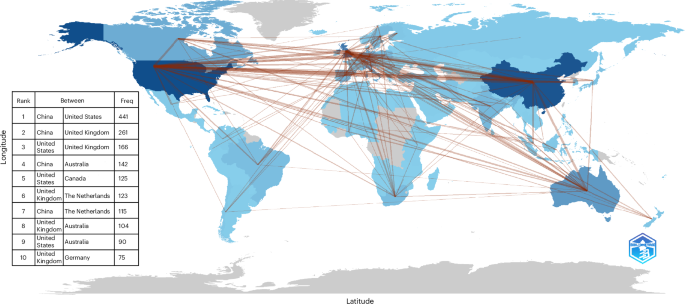
China followed by the United States and the United Kingdom dominates SDG11 research collaborations. There are significant connections among European, North American and Asian institutions, while Africa is less connected with Asia and Latin America and the Caribbean. Freq, frequently.
A co-citation analysis (Supplementary Table 3 ) reveals that Chinese institutions, such as the Chinese Academy of Sciences, have the highest number of articles and citation counts, followed by University College London and the University of Melbourne. The leading affiliations have changed over time, highlighting the strengthening of research institutes and the correlation between research collaboration and societal impacts (Supplementary Table 4 ). In terms of influential journals for SDG11 research, ‘land’ followed by ‘cities and land use’ policy tops the list (Supplementary Tables 5 and 6 ), with a growing interest in fields related to smart and sustainable cities, transport policies, regional planning and environmentally conscious building practices (Supplementary Fig. 4 ). These journals also address multiple issues related to environmental concerns, technological advancements, economic benefits, quality of life, justice and public awareness, driving the development of smart and sustainable cities.
The 15 most published authors in both periods focused on urbanization and urban growth, and the implementation, challenges and achievements of SDG11 (Supplementary Fig 5 ). This indicates an increased recognition of the SDG11 targets and their implementation over time, with the contributions of these authors significantly increasing from 2002 to 2016. Supplementary Table 7 shows that Chinese authors dominate the SDG11 publications, which correlates with China’s lead in institutions, affiliations and collaborations related to SDG11 research. The most cited SDG11 articles are revealed in Supplementary Table 8 , while the prominent authors that influenced SDG11 research are reported in Supplementary Table 9 . The top cited papers by SDG11 research are presented in Supplementary Tables 10 and 11 .
Key facts from the bibliometric analysis
The research on SDG11 has gained significant prominence across various fields, including urban studies, environmental sciences, geography, transportation and urban governance (Supplementary Table 12 ). The increasing environmental concerns, urbanization and global economic growth have spurred academic interest in SDG11 research from disciplines such as human geography, transportation, forestry, CC and sustainability science (Supplementary Table 13 ). Key thematic areas within SDG11 research encompass urban governance, affordable housing, transportation, urban sustainability and resilience, smart cities, urbanization and urban growth, which align closely with SDG11 targets 18 , 20 , 42 , 43 . However, research focus on SDG11 has remained relatively stable, with limited attention given to urban inequalities, safeguarding cultural and natural heritage 41 and specific impacts of the COVID-19 pandemic on urban sustainability.
This study reveals a notable increase in the total SDG11 research output from 2016 to 2022, reflecting the growing emphasis on SDG11 research in recent years compared with earlier periods. China emerges as the leaders in terms of research outputs, citations, authors, institutions and collaborations, closely followed by the United States and the United Kingdom. These three countries contribute 47.71% of SDG11 research productivity within this period, which is higher than 31% reported in a previous similar study 28 .
The dominance of Global North countries in the top 20 countries with the highest number of publications and citations related to SDG11 research is expected given their strong institutional capacity, research funding, highly ranked universities and collaborations. China’s surge in publications on SDG11 can be attributed to rapid urbanization, economic growth, government support and active international collaborations 2 , 11 . Generally, the landscape of research on SDG11 demonstrates an Anglo–American hegemony, which may reinforce power asymmetries and have significant implications for sustainability and resilience 30 . It is concerning that while projections indicate that 90% of future urban population growth will occur in cities of the Global South, particularly Africa and Asia, there is limited research on urban development challenges in these regions 7 .
The debate about the politics of knowledge production in SDG11 research often revolves around the controls of knowledge production processes. Large, well-funded institutions in developed countries tend to dominate research agendas, focusing on themes and solutions relevant to their own contexts, overlooking the unique needs and challenges of the Global South, which perpetuate existing inequalities and privileging certain types of knowledge. Also, knowledge production involves recognizing and integrating diverse ways of knowing. While Western scientific paradigms have traditionally dominated SDG11 research, there is an increasing recognition of the importance of indigenous and non-Western knowledge systems. Integrating these diverse epistemologies enriches understanding and leads to more effective and culturally relevant solutions.
Additionally, SDG11 research is inherently interdisciplinary, involving fields such as urban planning, sociology, environmental science and public policy. However, interdisciplinary collaboration can be challenging due to differing terminologies, methodologies and research priorities. Navigating these differences becomes crucial in the politics of knowledge production to create cohesive and comprehensive research outputs. Finally, bridging the gap between knowledge production and its implementation faces political, economic and social barriers. Researchers and practitioners are increasingly considering how knowledge on urban sustainability can effectively influence policymaking and practice in diverse urban contexts. Mobilizing knowledge to address these barriers becomes a key consideration in the politics of knowledge production.
Challenges to achieving SDG11
There are several challenges to achieving SDG11 targets, including inadequate provision of affordable housing 31 , essential services 24 , green spaces 2 , 34 , efficient transportation 33 and conservation of cultural and natural assets 25 . Rapid urbanization 1 , 7 , CC impacts 44 , insufficient investment in public infrastructure 30 , poor governance 13 and widening livelihood, land and resources inequalities 43 further exacerbate these challenges. For example, rapid urbanization puts immense pressure on housing, infrastructure, services and resources, making it challenging to effectively manage urban growth and ensure sustainable urban development 11 . Inadequate urban planning and land-use policies lead to inefficient land utilization, urban sprawl and inadequate provision of basic services 7 , 21 . The existence of slums and informal settlements where a large portion of the urban dwellers live in substandard housing conditions without tenure security 14 and limited access to electricity, water, sanitation, education, healthcare and employment opportunities 23 , 37 , and marginalized and vulnerable populations facing social exclusion, add to the complexity.
Moreover, competing priorities and trade-offs, lack of integration among various urban sectors and agencies 35 , inadequate human, technical and material resources at local government levels 45 , and insufficient local indicators and methods for implementation and monitoring 46 often hamper the implementation of SDG11 targets. Additionally, limited awareness of SDG-related challenges for policy formulation and implementation hinders context-depended decision-making and targeted interventions 21 , 27 . Addressing social inequalities, ensuring inclusivity in urban development and synergy among multiple fields, including social, technical, environmental, policy and management are crucial for achieving SDG11 (refs. 14 , 26 , 46 ). A valuable lesson can be learned from the success of the framework for assessing the implementation of SDG11 targets at the local level in Japan 42 .
Conclusions
This study aims to enhance our understanding of urban sustainability and provide insights for future research, policies and actions needed to achieve SDG11 targets. By conducting a comprehensive bibliometric assessment of over 21,000 publications from 2016 to 2022, it significantly contributes to the existing body of knowledge, highlighting trends, thematic areas and knowledge gaps related to SDG11 research across countries, institutions, authors and journals. SDG11 research has evolved into a multidisciplinary field, encompassing diverse themes, such as transportation, housing, urban sustainability, smart cities, urbanization and urban governance and policy. However, there is a need to address the gaps in research on urban safety and inclusion, which are critical dimensions often overlooked in favor of environmental and economic aspects of sustainability. This imbalance in research thematic areas risks perpetuation of already existing disparities within SDG11 research and its goals.
China, the United States and the United Kingdom emerge as the top contributors to SDG11 research and collaboration. To foster more SDG11 research in low-income economies, it is essential to provide increased funding support, capacity building and training for scholars, promote collaboration and knowledge exchange, and improve research infrastructure and data collection. Despite global challenges such as armed conflicts, CC and the COVID-19 pandemic, progress toward achieving the SDGs will become apparent by 2030. However, there are still opportunities for further research, knowledge dissemination and international collaboration toward developing safe, sustainable and inclusive urban development. The following are priority areas for SDG11 research:
Urban policy and governance: reforms should focus on providing equitable access to basic services such as water, sanitation, electricity, healthcare and education; upgrading and formalizing informal settlements; and improving living conditions of over one billion people residing in slums 37 . Participatory governance, community engagement and empowerment can enhance social inclusion by considering the voices and needs of marginalized groups 13 , 23 . Urban policy should also prioritize preserving historic and natural resources, protecting vulnerable areas and implementing sustainable urban design principles 47 . Future studies can help understand the dynamics, challenges and opportunities and monitor progress toward SDG11 targets 15 .
Localizing SDG11 targets: spatial planning and land-use strategies should consider the needs of diverse urban populations, promote inclusive zoning and engage local communities and stakeholders in decision-making processes, crucial for fostering ownership, empowerment and social cohesion, leading to more sustainable and inclusive urban development 3 . However, enhancing the capacity for localizing SDG11 targets requires building the knowledge and skills of local governments, policymakers and practitioners. Capacity-building initiatives, such as training programs, workshops and knowledge exchange, can promote interdisciplinary understanding and sharing of best practices.
Concerted and collaborative efforts: the international community, academics, policymakers and stakeholders can work together to create inclusive, safe, resilient and sustainable communities. Collaborative efforts can facilitate a comprehensive understanding of urban challenges and potential solutions by integrating diverse perspectives, data and methodologies. Disseminating research findings contributes to evidence-based policy development and informed decision-making, enabling the learning of lessons and replication of successful interventions.
Breaking down silos: integrated and cross-sectoral approaches help narrow the gaps between sectors, local governments, policymakers and stakeholders, leveraging local resources and capacities while fostering communication, knowledge sharing and collaboration 31 . Cross-sectoral working groups, joint planning processes and integrated policy frameworks promote holistic and coordinated decision-making among various sectors, including urban planning, housing, transportation, health, education, environment and social welfare 47 .
Digitalization and smart city development: maximizing the benefits of digitalization and smart city solutions requires addressing challenges such as bridging digital divides and ensuring data access, privacy and security. Prioritizing citizen-centric approaches and public accessibility to technology 36 are essential for leveraging expertise and resources 15 . Interoperability, scalability, data-driven decision-making and inclusivity contribute to evidence-based planning and equitable access to smart city technologies 12 , 48 , 49 , 50 , 51 .
This study comprehensively assessed SDG11 research, emphasizing significant thematic areas, trends, challenges and suggestions for prioritizing SDG11, including effective urban policy and governance, localizing SDG11 targets, concerted and collaborative efforts, and digitalization and smart city development. To broaden the scope of SDG11 research, future bibliometric reviews should encompass non-Web of Science databases and gray literature, including publications from government and nongovernmental agencies. Despite its limitations, this study’s findings provide valuable references for further research on SDG11.
The present study utilized a bibliometric technique to analyze academic publication on SDG11, tracing the research trend, the evolving key themes and identifying contributing authors, institutions and countries. Bibliometrics is a quantitative technique that allows for the analysis of trends in scholarly publications, such as research articles, conference papers and books, and visualizes scholarly publication patterns 52 . This technique is instrumental in analyzing extensive literature sets by relying on statistical observations and text-mining capabilities, which qualitative review methods such as systematic reviews cannot accomplish 53 . Additionally, it presents a scientific landscape of authors, countries, organizations and collaborations that contribute to worldwide scientific literature.
Bibliometric analysis requires interpretation, introducing an element of subjectivity 54 . Therefore, a sensemaking approach was adopted to transition from describing the bibliometric results to interpreting them. Sensemaking helps derive insightful information from bibliometric analysis and can be integrated into systematic literature reviews 55 , 56 . It applies to various international indexing, abstracting and citation databases, such as Scopus, Web of Science, Dimensions, PubMed and Education Resources Information Center, which cover journals, books, reviews and conference proceedings from around the world and different regions. For this study, Web of Science was chosen as the database to obtain bibliographic data due to its wide range of topics in various fields of study such as natural sciences, health sciences, engineering, social science, computer science and materials sciences. It is one of the world’s largest peer-reviewed scientific literature databases, with 87 million indexed items.
Specialized bibliometrics software were employed, including VOSviewer (version 1.6.19) 52 , Biblioshiny (version 4.1.3) 55 and BibExcel (version 2017) 57 . VOSviewer, known for its user-friendly interface, was used to understand the thematic focus and evolution of research on SDG11. It generates networks of nodes and links, with node size representing the frequency of the studied item, and link width indicating the strength of connections between items. Clusters of intricately linked nodes are shown in distinct colors. The thematic focus was examined for two periods: period 1 (2016–2019) and period 2 (2020–2022), considering the time since the SDGs were introduced to the time of data collection in this study. Another reason for this categorization is that evidence shows that the pandemic has significantly affected progress toward achieving SDGs 58 . VOSviewer allows for various types of analysis, including term co-occurrence, co-citation, citation and bibliographic coupling 53 . A term co-occurrence analysis was used in this study to highlight key thematic areas. To ensure accuracy and avoid separate counting of synonyms, a thesaurus file was developed and added to the software before the analysis. A summary of the data, including the number of authors and journals, used in the analysis is presented in Table 1 and will be further explained below.
A comprehensive search query was formulated to retrieve relevant data on SDG11, and it was executed in the title, abstract and keywords fields (TS) in Web of Science on 5 July 2023. The initial query shown the following box resulted in a total of 334,224 documents. Co-citation analysis was employed to identify the most influential journals contributing to SDG11 research. Two works are considered co-cited when they are both mentioned in the works cited section of a subsequent publication 59 (Zhao, 2006).
TS = ((‘city’ OR ‘cities’ OR ‘human settlement*’ OR ‘urban’ OR ‘metropoli*’ OR ‘town*’ OR ‘municipal*’ OR ‘peri-urban*’ OR ‘urban-rural’ OR ‘rural-urban’) AND (‘gentrification’ OR ‘congestion’ OR ‘transport*’ OR ‘housing’ OR ‘slum*’ OR ‘informal settlement*’ OR ‘sendai framework’ OR ‘Disaster Risk Reduction’ OR ‘disaster’ OR ‘DRR’ OR ‘smart cit*’ OR ‘resilient building*’ OR ‘sustainable building*’ OR ‘building design’ OR ‘buildings design’ OR ‘urbani?ation’ OR ‘zero energy’ OR ‘zero-energy’ OR ‘basic service*’ OR ‘governance’ OR ‘citizen participation’ OR ‘collaborative planning’ OR ‘participatory planning’ OR ‘inclusiveness’ OR ‘cultural heritage’ OR ‘natural heritage’ OR ‘UNESCO’ OR ‘ecological footprint’ OR ‘environmental footprint’ OR ‘waste’ OR ‘pollution’ OR ‘pollutant*’ OR ‘waste water’ OR wastewater* OR waste-water* OR ‘recycling’ OR ‘circular economy’ OR ‘air quality’ OR ‘green space’ OR ‘green spaces’ OR ‘nature inclusive’ OR ‘nature inclusive building’ OR ‘nature inclusive buildings’ OR ‘resilient’ OR ‘resilience’ OR ‘healthy cit*’ OR ‘sustainable’ OR ‘sustainability’ OR ‘green’ OR ‘nature*’ OR ‘Green infrastructure*’ OR ‘nature-based solution*’ OR ‘nature based solution*’ OR ‘child*’ OR ‘wom?n’ OR ‘elderl*’ OR ‘disabl*’ OR ‘disabilit*’ OR ‘disabled’)) AND PY = (2016–2022) NOT PY = (2023)
The Preferred Reporting Items for Systematic Reviews and Meta-Analyses (PRISMA) framework was used to report document search and filtration process. The PRISMA framework is designed to help scholars transparently report why their review study is conducted, what activities are performed and what discoveries are made, ideal for both systematic reviews and bibliometric studies 60 . PRISMA presents the four stages of the above query’s overall searching and filtration process (Fig. 4 ). The identification stage yielded 334,224 records, which were then screened to select only article-type documents ( n = 277,165). Subsequently, documents were further screened based on language, selecting only English documents ( n = 257,374). In the final stage, documents were screened based on specific categories closely related to cities and SDG11, resulting in a selection of six major categories: urban studies, environmental studies, geography, urban and regional planning, architecture, transportation and physical geography ( n = 21,168). Finally, 15 duplicated documents were removed, resulting in a final dataset of 21,153 documents.
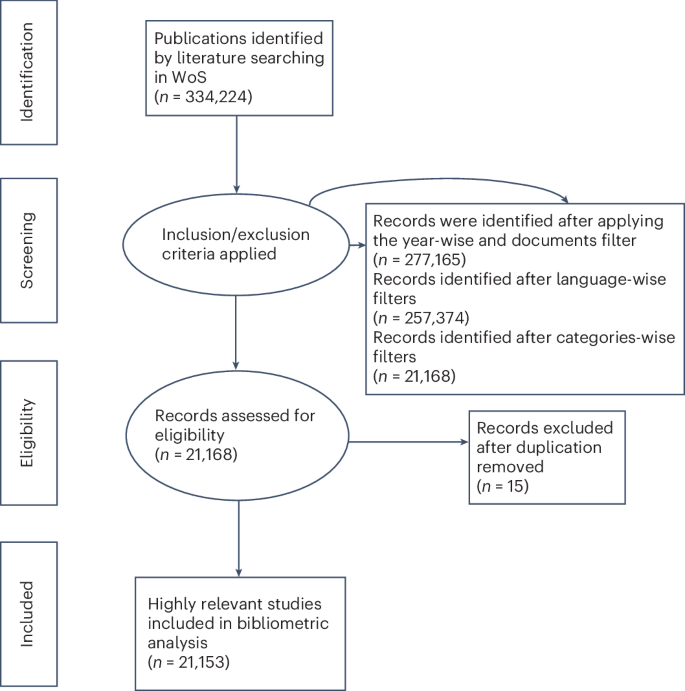
A four-phase flow diagram of the data extraction and filtration process of SDG11 literature, adapted from Priyadarshini 57 . WoS, Web of Science.
Reporting summary
Further information on research design is available in the Nature Portfolio Reporting Summary linked to this article.
Data availability
The data that support the findings of this study are available as supplementary information. The steps for curating the data from the Web of Science have been provided in the text. If there is a further need, data are available on figshare at https://doi.org/10.6084/m9.figshare.26360125 . Source data are provided with this paper.
Almulhim, A. I. & Cobbinah, P. B. Can rapid urbanization be sustainable? The case of Saudi Arabian cities. Habitat Int. 139 , 102884 (2023).
Article Google Scholar
Huang, W. et al. Evaluating green city development in China using an integrated analytical toolbox. J. Clean. Prod. 400 , 136703 (2023).
Feng, T. & Zhou, B. Impact of urban spatial structure elements on carbon emissions efficiency in growing megacities: the case of Chengdu. Sci. Rep. 13 , 9939 (2023).
Javeed, S., Siddique, H. M. A. & Javed, F. Ecological footprint, globalization, and economic growth: evidence from Asia. Environ. Sci. Pollut. Res. 30 , 77006–77021 (2023).
Rees, W. E. Cities, energy, and the uncertain future of urban civilization. Oxf. Dev. Stud. 51 , 11–17 (2023).
Abubakar, I. R. & Aina, Y. A. The prospects and challenges of developing more inclusive, safe, resilient, and sustainable cities in Nigeria. Land Use Policy 87 , 104105 (2019).
UN-Habitat World Cities Report 2022: Envisaging the Future of Cities. (UN-Habitat, 2022).
Almulhim, A. I. & Cobbinah, P. B. Framing resilience in Saudi Arabian cities: on climate change and urban policy. Sustain. Cities Soc. 101 , 105172 (2024).
Barbier, E. B. & Burgess, J. C. Sustainability and development after COVID-19. World Dev. 135 , 105082 (2020).
The Sustainable Development Goals Report 2018 (United Nations, 2018).
Chen, M., Chen, L., Cheng, J. & Yu, J. Identifying interlinkages between urbanization and Sustainable Development Goals. Geogr. Sustain. 3 , 339–346 (2022).
Clement, J., Ruysschaert, B. & Crutzen, N. Smart city strategies—a driver for localizing sustainable development goals? Ecol. Econ. 213 , 107941 (2023).
Hansson, S., Arfvidsson, H. & Simon, D. Governance for sustainable urban development: the double function of SDG indicators. Area Dev. Policy 4 , 217–235 (2019).
Leal Filho, W. et al. Using the sustainable development goals towards a better understanding of sustainability challenges. Int. J. Sustain. Dev. World Ecol. 26 , 179–190 (2019).
Parra-Dominguez, J., Gil-Egido, A. & Rodríguez-González, S. SDGs as one of the drivers of smart city development: the indicator selection process. Smart Cities 5 , 1025–1038 (2022).
Grainger-Brown, J., Malekpour, S., Raven, R. & Taylor, E. Exploring urban transformation to inform the implementation of the Sustainable Development Goals. Cities 131 , 103928 (2022).
Xu, Z. et al. Assessing progress towards sustainable development over space and time. Nature 577 , 74–78 (2020).
Salvia, A. L., Leal Filho, W., Brandli, L. L. & Griebeler, J. S. Assessing research trends related to Sustainable Development Goals: local and global issues. J. Clean. Prod. 208 , 841–849 (2019).
Indana, F. & Pahlevi, R. W. A bibliometric approach to Sustainable Development Goals (SDGs) systematic analysis. Cogent Bus. Manag. 10 , 2224174 (2023).
Yamaguchi, N. U. et al. Sustainable development goals: a bibliometric analysis of literature reviews. Environ. Sci. Pollut. Res. 30 , 5502–5515 (2023).
Pattberg, P. & Bäckstrand, K. Enhancing the achievement of the SDGs: lessons learned at the half-way point of the 2030 Agenda. Int. Environ. Agree. 23 , 107–114 (2023).
Keith, M. et al. A new urban narrative for sustainable development. Nat. Sustain. 6 , 115–117 (2023).
Pedersen, A. B. et al. SDGs at the halfway point: how the 17 global goals address risks and wicked problems. Ambio 52 , 679–682 (2023).
Sweileh, W. M. Bibliometric analysis of scientific publications on ‘sustainable development goals’ with emphasis on ‘good health and well-being’ goal (2015–2019). Global. Health 16 , 68 (2020).
Mihelcic, J. R. et al. Environmental research addressing Sustainable Development Goals. Environ. Sci. Technol. 57 , 3457–3460 (2023).
Palau-Pinyana, E., Llach, J. & Bagur-Femenías, L. Mapping enablers for SDG implementation in the private sector: a systematic literature review and research agenda. Manag. Rev. Q. 26 , 1–30 (2023).
Google Scholar
Mishra, M. et al. A bibliometric analysis of sustainable development goals (SDGs): a review of progress, challenges, and opportunities. Environ. Dev. Sustain. 26 , 11101–11143 (2023).
Devisscher, T. et al. in Sustainable Development Goals: Their Impacts on Forests and People (eds. Katila, P. et al.) (Cambridge Univ. Press, 2019).
Brenner, N. & Theodore, N. Cities and the geographies of ‘actually existing neoliberalism’. Antipode 34 , 349–379 (2002).
Sharifi, A., Khavarian-Garmsir, A. R., Allam, Z. & Asadzadeh, A. Progress and prospects in planning: a bibliometric review of literature in urban studies and regional and urban planning, 1956–2022. Prog. Plann. 173 , 100740 (2023).
Olanrewaju, A., Tan, S. Y. & Abdul-Aziz, A.-R. Housing providers’ insights on the benefits of sustainable, affordable housing. Sustain. Dev. 26 , 847–858 (2018).
Rice, J. L., Cohen, D. A., Long, J. & Jurjevich, J. R. Contradictions of the climate-friendly city: new perspectives on eco-gentrification and housing justice. Int. J. Urban Reg. Res. 44 , 145–165 (2020).
Park, S., Choi, K. & Lee, J. S. To walk or not to walk: testing the effect of path walkability on transit users’ access mode choices to the station. Int. J. Sustain. Transp. 9 , 529–541 (2015).
Jayasooriya, V. M., Ng, A. W. M., Muthukumaran, S. & Perera, B. J. C. Green infrastructure practices for the improvement of urban air quality. Urban For. Urban Green. 21 , 34–47 (2017).
Hachaichi, M. & Egieya, J. Water–food–energy nexus in global cities: addressing complex urban interdependencies. Water Resour. Manag. 37 , 1811–1825 (2023).
Mora, L., Deakin, M. & Reid, A. Combining co-citation clustering and text-based analysis to reveal the main development paths of smart cities. Technol. Forecast. Soc. Change 142 , 56–69 (2019).
McFarlane, C. Rethinking informality: politics, crisis, and the city. Plan. Theory Pract. 13 , 89–108 (2012).
Harvey, D. From managerialism to entrepreneurialism: the transformation in urban governance in late capitalism. Geogr. Ann. B 71 , 3–17 (1989).
Peck, J. Political economies of scale: fast policy, interscalar relations, and neoliberal workfare. Econ. Geogr. 78 , 331–360 (2002).
Cervero, R. & Landis, J. Twenty years of the Bay Area rapid transit system: land use and development impacts. Transport. Res. A 31 , 309–333 (1997).
Guzman, P. C., Roders, A. R. P. & Colenbrander, B. J. F. Measuring links between cultural heritage management and sustainable urban development: an overview of global monitoring tools. Cities 60 , 192–201 (2017).
Yamasaki, K. & Yamada, T. A framework to assess the local implementation of Sustainable Development Goal 11. Sustain. Cities Soc. 84 , 104002 (2022).
van Zanten, J. A. & van Tulder, R. Towards nexus-based governance: defining interactions between economic activities and Sustainable Development Goals (SDGs). Int. J. Sustain. Dev. World Ecol. 28 , 210–226 (2021).
Londono-Pineda, A. A. & Cano, J. A. Assessments under the United Nations sustainable development goals: a bibliometric analysis. Environ. Clim. Technol. 26 , 166–181 (2022).
Biggeri, M. A. ‘Decade for Action’ on SDG localization. J. Hum. Dev. Capabil. 22 , 706–712 (2021).
Benedek, J., Ivan, K., Török, I., Temerdek, A. & Holobâcă, I. H. Indicator-based assessment of local and regional progress toward the Sustainable Development Goals (SDGs): an integrated approach from Romania. Sustain. Dev. 29 , 860–875 (2021).
Abubakar, I. R. & Alshammari, M. S. Urban planning schemes for developing low-carbon cities in the Gulf Cooperation Council region. Habitat Int. 138 , 102881 (2023).
Batty, M. Smart cities, big data. Environ. Plann. B 39 , 191–193 (2012).
Smith, N. Toward a theory of gentrification, a back to the city movement by capital, not people. J. Am. Plann. Assoc. 45 , 538–548 (1979).
Jacobs, J. The Death and Life of Great American Cities (Random House, 1961).
Smith, N. The New Urban Frontier: Gentrification and the Revanchist City (Routledge, 1996).
Donthu, N., Kumar, S., Mukherjee, D., Pandey, N. & Lim, W. M. How to conduct a bibliometric analysis: an overview and guidelines. J. Bus. Res. 133 , 285–296 (2021).
van Eck, N. J. & Waltman, L. Software survey: VOSviewer, a computer program for bibliometric mapping. Scientometrics 84 , 523–538 (2010).
Hajek, P., Youssef, A. & Hajkova, V. Recent developments in smart city assessment: a bibliometric and content analysis-based literature review. Cities 126 , 103709 (2022).
Lim, W. M. & Kumar, S. Guidelines for interpreting the results of bibliometrics analysis: a sensemaking approach. Glob. Bus. Organ. Excell. 43 , 17–26 (2023).
Aria, M. & Cuccurullo, C. bibliometrix: an R-tool for comprehensive science mapping analysis. J. Informetr. 11 , 959–975 (2017).
Åström, F., Danell, R., Larsen, B. & Schneider, J. (eds) Celebrating Scholarly Communication Studies: A Festschrift for Olle Persson at His 60th Birthday Vol. 05-S (International Society for Scientometrics and Informetrics, 2009).
Priyadarshini, P. The COVID-19 pandemic has derailed the progress of Sustainable Development Goals. Anthr. Sci. 1 , 410–412 (2022).
Zhao, D. Towards all-author co-citation analysis. Inf. Process. Manag. 42 , 1578–1591 (2006).
Shamseer, L. et al. Preferred reporting items for systematic review and meta-analysis protocols (PRISMA-P) 2015: elaboration and explanation. BMJ 349 , g7647 (2015).
Download references
Acknowledgements
A.I.A. acknowledges Imam Abdulrahman Bin Faisal University in Dammam, Saudi Arabia, for their support in conducting this study. A.S. acknowledges the support of the Japan Society for the Promotion of Science KAKENHI grant number 22K04493. We appreciate Hiroshima University for supporting the open-access publication of this article.
Author information
Authors and affiliations.
Department of Urban and Regional Planning, College of Architecture and Planning, Imam Abdulrahman Bin Faisal University, Dammam, Saudi Arabia
Abdulaziz I. Almulhim
The IDEC Institute and Network for Education and Research on Peace and Sustainability, Hiroshima University, Hiroshima, Japan
Ayyoob Sharifi
Department of Geomatics Engineering Technology, Yanbu Industrial College, Yanbu, Saudi Arabia
Yusuf A. Aina
Directorate of Library Affairs, Imam Abdulrahman Bin Faisal University, Dammam, Saudi Arabia
Shakil Ahmad
The Business School, Edinburgh Napier University, Edinburgh, UK
Academy of Architecture and Urban Studies, Tallinn University of Technology, Tallinn, Estonia
Department of Natural Sciences, Manchester Metropolitan University, Manchester, UK
Walter Leal Filho
Research and Transfer Centre ‘Sustainable Development and Climate Change Management’, Hamburg University of Applied Sciences, Hamburg, Germany
College of Architecture and Planning, Imam Abdulrahman Bin Faisal University, Dammam, Saudi Arabia
Ismaila Rimi Abubakar
You can also search for this author in PubMed Google Scholar
Contributions
A.I.A.: conceptualization, methodology, formal analysis, data curation, writing—original draft, writing—review and editing, investigation and project administration. A.S.: methodology, software, formal analysis, visualization and writing—original draft. Y.A.A.: conceptualization, writing—original draft, investigation and validation. S.A.: methodology, software, formal analysis, visualization and data curation. L.M.: writing—review and editing, and investigation. W.L.F.: writing—review and editing, and investigation. I.R.A.: writing—review and editing, investigation, supervision, validation and resources.
Corresponding authors
Correspondence to Abdulaziz I. Almulhim or Ayyoob Sharifi .
Ethics declarations
Competing interests.
The authors declare no competing interests.
Peer review
Peer review information.
Nature Cities thanks Aliyu Salisu Barau, Oluwatobi Owojori, and the other, anonymous, reviewer(s) for their contribution to the peer review of this work.
Additional information
Publisher’s note Springer Nature remains neutral with regard to jurisdictional claims in published maps and institutional affiliations.
Supplementary information
Supplementary information.
Supplementary Figs. 1–5 and Tables 1–13.
Reporting Summary
Source data fig. 1.
Statistical source data in Excel format.
Source Data Fig. 2
Source data fig. 3, source data fig. 4, rights and permissions.
Open Access This article is licensed under a Creative Commons Attribution 4.0 International License, which permits use, sharing, adaptation, distribution and reproduction in any medium or format, as long as you give appropriate credit to the original author(s) and the source, provide a link to the Creative Commons licence, and indicate if changes were made. The images or other third party material in this article are included in the article’s Creative Commons licence, unless indicated otherwise in a credit line to the material. If material is not included in the article’s Creative Commons licence and your intended use is not permitted by statutory regulation or exceeds the permitted use, you will need to obtain permission directly from the copyright holder. To view a copy of this licence, visit http://creativecommons.org/licenses/by/4.0/ .
Reprints and permissions
About this article
Cite this article.
Almulhim, A.I., Sharifi, A., Aina, Y.A. et al. Charting sustainable urban development through a systematic review of SDG11 research. Nat Cities (2024). https://doi.org/10.1038/s44284-024-00117-6
Download citation
Received : 22 September 2023
Accepted : 30 July 2024
Published : 27 August 2024
DOI : https://doi.org/10.1038/s44284-024-00117-6
Share this article
Anyone you share the following link with will be able to read this content:
Sorry, a shareable link is not currently available for this article.
Provided by the Springer Nature SharedIt content-sharing initiative
Quick links
- Explore articles by subject
- Guide to authors
- Editorial policies
Sign up for the Nature Briefing newsletter — what matters in science, free to your inbox daily.

Literature review on achievement goals and classroom goal structure: Implications for future research
- December 2010
- Electronic Journal of Research in Educational Psychology 8(3):1363-1386
- 8(3):1363-1386

- Sultan Qaboos University
Discover the world's research
- 25+ million members
- 160+ million publication pages
- 2.3+ billion citations
- Huy P. Phan

- Alnabhani Hilal

- Vinika Chophy
- LEARN INDIVID DIFFER
- David B. Feldman
- Maximilian Kubota

- Michael J. Middleton

- Carol Midgley
- Elaine S. Elliott
- Carol S. Dweck

- Martin L. Maehr
- Marcy A. Church

- J Cognit Psychother
- Albert Bandura
- W.H. Freeman
- Richard Lightsey
- J.S. Coleman
- SCHOOL PSYCHOL REV
- Elizabeth A. Linnenbrink
- Paul R. Pintrich
- J. Donald Moon
- James S. Coleman
- Recruit researchers
- Join for free
- Login Email Tip: Most researchers use their institutional email address as their ResearchGate login Password Forgot password? Keep me logged in Log in or Continue with Google Welcome back! Please log in. Email · Hint Tip: Most researchers use their institutional email address as their ResearchGate login Password Forgot password? Keep me logged in Log in or Continue with Google No account? Sign up

IMAGES
VIDEO
COMMENTS
2. MOTIVATE YOUR RESEARCH in addition to providing useful information about your topic, your literature review must tell a story about how your project relates to existing literature. popular literature review narratives include: ¡ plugging a gap / filling a hole within an incomplete literature ¡ building a bridge between two "siloed" literatures, putting literatures "in conversation"
Where the emphasis is on an investigation or analysis of the literature (analytical evaluation) then your literature review is concentrating on the nature of the problem, its cause and effect as a basis for action to solve it. FORMATIVE When a literature review emphasizes explanation of what you believe the knowledge stemming from
Its goal is to bring the reader up-to-date with current hterature on a topic and form the basis for another goal, such as the justification for future research in the area. A good literature review gathers information about a particular subject from many sources. It is well written and contains few if any personal biases. It should contain a ...
lls the reader, and why it is necessary.3.2 Evaluate the nine basic steps taken to wr. te a well-constructed literature review.3.3 Conduct an electronic search using terms, phrases, Boolean operators, and filters.3.4 Evaluate and identify the parts of an empirical research journal article, and use that kn.
Literature reviews allow scientists to argue that they are expanding current. expertise - improving on what already exists and filling the gaps that remain. This paper demonstrates the literatu ...
Literature ReviewChapter 7 ObjectivesSection I: InstructionProvide an understanding of the. unction and purpose of a literature review (the "what").Describe the role of a research-based. ritical literature review in a dissertation (the "why").Outline the skills related to the various steps involved in conducting and presenting a ...
The Literature Review. September 2015. DOI: 10.23912/978-1-910158-51-7-2790. In book: Research Methods for Business and Management. Publisher: Goodfellow Publishers Limited. Editors: Robert ...
unearth literature that is appropriate to your task in hand, a literature review is the process of critically evaluating and summarising that literature. The Purpose of the Literature Review: The Question and Context Conceptualising the Literature Review Think of a topic that interests you in clinical practice. Imagine this as a wide-rimmed,
A literature review is a surveys scholarly articles, books and other sources relevant to a particular. issue, area of research, or theory, and by so doing, providing a description, summary, and ...
Examples of literature reviews. Step 1 - Search for relevant literature. Step 2 - Evaluate and select sources. Step 3 - Identify themes, debates, and gaps. Step 4 - Outline your literature review's structure. Step 5 - Write your literature review.
The goal of a literature review is to summarize and synthesize the previous research and arguments of other scholars, without adding new contributions. The goal of a research paper is to develop a new argument, and typically includes some form of data collection and analysis. A research paper usually includes a literature review as one of its ...
As mentioned previously, there are a number of existing guidelines for literature reviews. Depending on the methodology needed to achieve the purpose of the review, all types can be helpful and appropriate to reach a specific goal (for examples, please see Table 1).These approaches can be qualitative, quantitative, or have a mixed design depending on the phase of the review.
What are the goals of creating a Literature Review? A literature could be written to accomplish different aims: To develop a theory or evaluate an existing theory; To summarize the historical or existing state of a research topic; Identify a problem in a field of research ; Baumeister, R. F., & Leary, M. R. (1997). Writing narrative literature ...
The purpose of a literature review is to gain an understanding of the existing research and debates relevant to a particular topic or area of study, and to present that knowledge in the form of a written report. Conducting a literature review helps you build your knowledge in your field. You'll learn about important concepts, research methods ...
udy begins with a review of the literature. The purpose of the literature review section of a research article is to provide the reader with an overall framework for where this piece of work fits in the "big picture" of what is. known about a topic from previous research. Thus, the literature review serves to explain the topic of the ...
Literature reviews establish the foundation of academic inquires. However, in the planning field, we lack rigorous systematic reviews. In this article, through a systematic search on the methodology of literature review, we categorize a typology of literature reviews, discuss steps in conducting a systematic literature review, and provide suggestions on how to enhance rigor in literature ...
Referring to existing research, particularly in the work (literature review) section of a paper related. e.g. Although people's actual behaviour has previously been found to be non-privacy protecting [10,45,56], this may be because of poor user interfaces [23,30], or users' goal-driven behaviour [15,21,29] Use to build your. "state of the ...
The literature review is the nucleus of a research work that might when gotten right spotlights a work and can as well derail a research work when done wrongly. This paper seeks to unveil the practical guides to writing a literature review, from purpose, and components to tips. It follows through the exposition of secondary literature.
Now, use evidence from your sources to discuss your take on the literature. Be selective: Use information most directly relevant to the thesis. Maintain your own voice: Use quotes sparingly; summarize and paraphrase. Synthesize: Rephrase significance; relate information to your own argument.
GOALS OF A LITERATURE REVIEW: Before doing work in primary sources, historians must know what has been written on their topic. They must be familiar with theories and arguments-as well as facts-that appear in secondary sources. ... Your literature review must include enough works to provide evidence of both the breadth and the depth of the ...
apter_1.pdf). Purposes of review of literature The goal of review of literature is to provide a justification of the proposed research and this can be achieved through four main objectives. These are to review published literature (to identify and summarise relevant theories and researches), to critique the literature
The literature review establishes both the relevance and justifies why new research is relevant. It is through a literature review that a gap would be established, and which the new research would fix. Once the literature review sits properly in the research work, the objectives/research questions naturally fall into their proper perspective.
12 - Free download as Word Doc (.doc / .docx), PDF File (.pdf), Text File (.txt) or read online for free. The document discusses the four major goals of conducting a literature review. They are: 1) To justify one's research by identifying gaps in existing research. 2) To familiarize oneself with current knowledge on the topic. 3) To integrate and summarize prior research.
studies could examine the literature to see if there are differences between 100% online and hybrid e-learning as it related to knowledge sharing. This literature review is potentially beneficial to both e-learning and knowledge management fields. On the one hand, this review provides information to understand the
Background: Digital mental health technologies (DMHTs) have the potential to enhance mental health care delivery. However, there is little information on how DMHTs are evaluated and what factors influence their use. Objective: A systematic literature review was conducted to understand how DMHTs are valued in the United States from user, payer, and employer perspectives.
literature. Shale gas exploration and exploitation has been extensively debated in literature, but a comprehensive review of recent studies on the environmental impacts has yet to be carried out. Therefore, the goal of this article is to systemati-cally examine scientific articles published between 2010 and
We conducted a systematic review of the literature to answer our research questions. To ensure a thorough and transparent systematic literature review process, we carried this review using the Preferred Reporting Items for Systematic Reviews and Meta-Analysis (PRISMA) framework as a guideline (Page et al., 2021; Moher et al., 2009).The systematic literature review involved three major phases ...
Using bibliometric techniques, this Article assesses the evolving landscape of Sustainable Development Goal (SDG) 11 research, highlighting publication trends, thematic focus areas, authorship ...
The most prevalent research methods are literature review, interviews, workshops, discussions, etc. Besides, it is important to note that the field research available on automation's impacts in the mining context is scarce. Figure 2 shows a distribution of the keywords to explore the contents. The keywords examined are those used by writers in ...
This paper includes a review of literature discussing research related to achievement goal theory, stability of achievement goals, classroom goal structure, and multiple goals along with ...